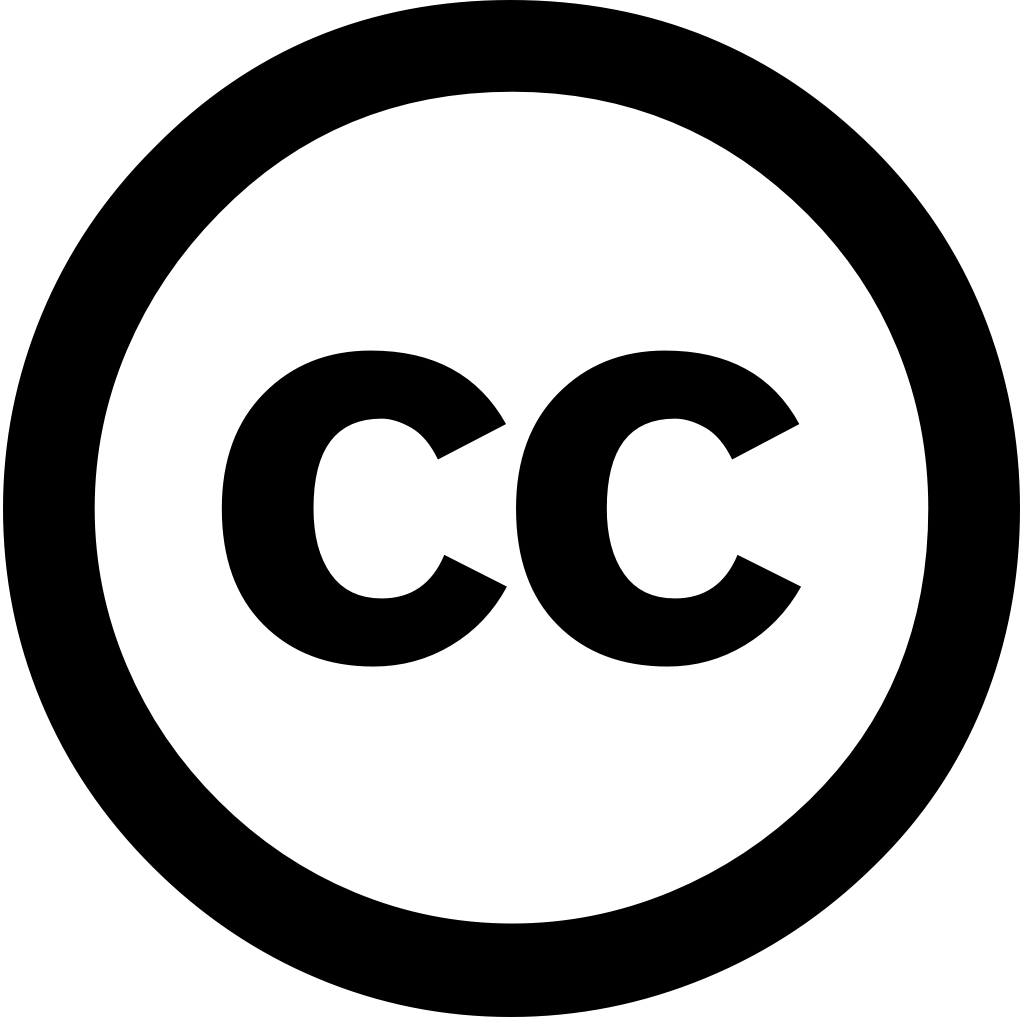
Desalination and Water Treatment, Год журнала: 2024, Номер 320, С. 100876 - 100876
Опубликована: Окт. 1, 2024
Язык: Английский
Desalination and Water Treatment, Год журнала: 2024, Номер 320, С. 100876 - 100876
Опубликована: Окт. 1, 2024
Язык: Английский
Journal of environmental chemical engineering, Год журнала: 2025, Номер unknown, С. 115621 - 115621
Опубликована: Янв. 1, 2025
Язык: Английский
Процитировано
0Advanced Theory and Simulations, Год журнала: 2025, Номер unknown
Опубликована: Апрель 17, 2025
Abstract Accurate nanofiber diameter estimation is crucial for optimizing their functionality in materials science. Traditional measurement methods from Scanning Electron Microscopy (SEM) images are often labor‐intensive and subjective. This study proposes a machine learning‐based approach using deep feature embeddings to predict average diameters directly SEM images. Eight learning models—Linear Regression (LR), k‐Nearest Neighbors (kNN), Decision Tree (DT), Random Forest (RF), Support Vector Machine (SVM), Neural Network (NN), Gradient Boosting (GB), AdaBoost—are evaluated 5‐fold 10‐fold cross‐validation. Performance assessed via Mean Squared Error (MSE), Root (RMSE), Absolute (MAE), Percentage (MAPE), R 2 . The kNN model consistently outperformed others across three datasets: smooth nanofibers, beaded combined set. It achieves the lowest error metrics highest (0.950) while demonstrating strong generalization morphologies. among first integrate with direct prediction, providing reliable alternative traditional methods.
Язык: Английский
Процитировано
0Desalination and Water Treatment, Год журнала: 2024, Номер 320, С. 100876 - 100876
Опубликована: Окт. 1, 2024
Язык: Английский
Процитировано
1