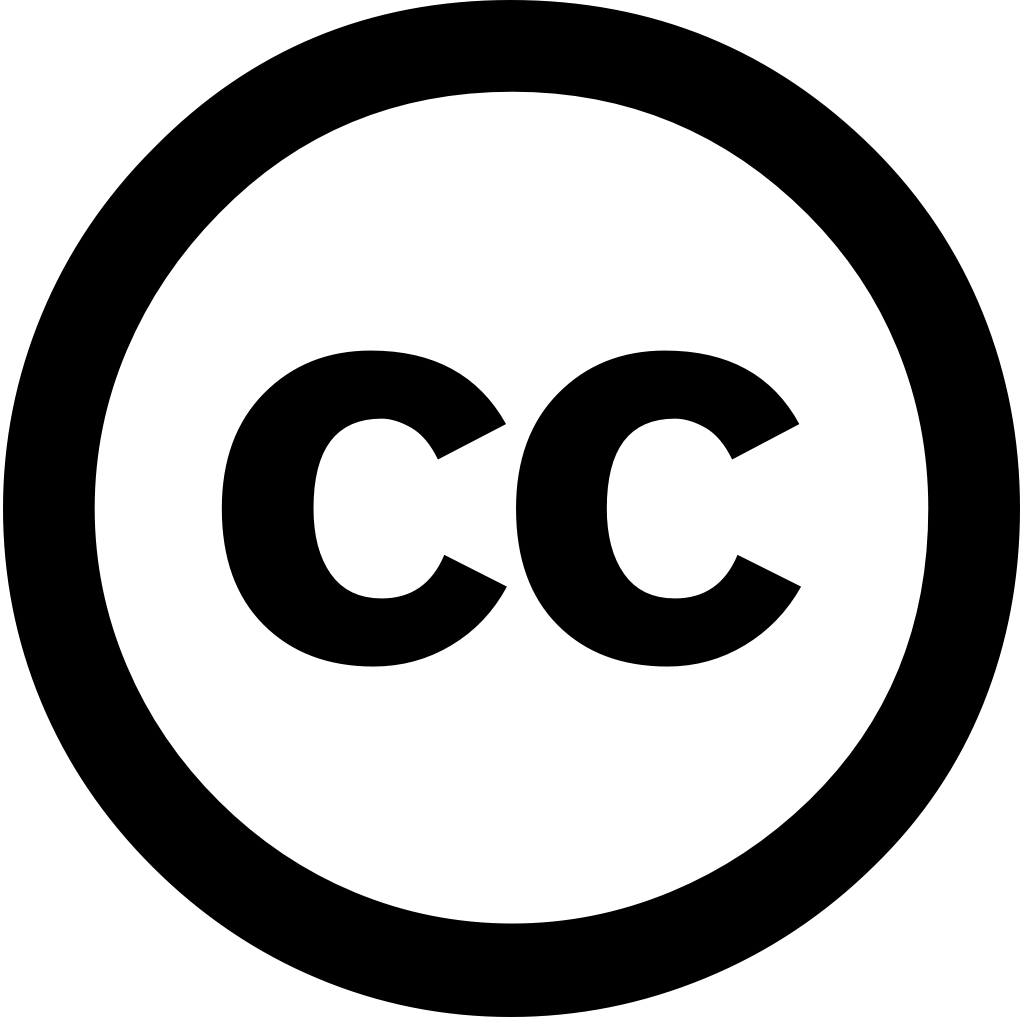
Geoscience Frontiers, Год журнала: 2024, Номер 16(1), С. 101960 - 101960
Опубликована: Ноя. 1, 2024
Язык: Английский
Geoscience Frontiers, Год журнала: 2024, Номер 16(1), С. 101960 - 101960
Опубликована: Ноя. 1, 2024
Язык: Английский
Springer eBooks, Год журнала: 2024, Номер unknown, С. 243 - 296
Опубликована: Янв. 1, 2024
Язык: Английский
Процитировано
5Journal of Hydroinformatics, Год журнала: 2024, Номер 26(8), С. 1852 - 1882
Опубликована: Июль 30, 2024
ABSTRACT The objective of this study was to develop a theoretical framework based on machine learning, the hydrodynamic model, and analytic hierarchy process (AHP) assess risk flooding downstream Ba River in Phu Yen. made up three main factors: flood risk, exposure, vulnerability. Hazard calculated from depth, velocity, susceptibility, which depth velocity were using susceptibility built namely, support vector machines, decision trees, AdaBoost, CatBoost. Flood exposure constructed by combining population density, distance river, land use/land cover. vulnerability poverty level road density. indices each factor integrated AHP. results showed that hydraulic model successful simulating events 1993 2020, with Nash–Sutcliffe efficiency values 0.95 0.79, respectively. All learning models performed well, area under curve (AUC) more than 0.90; among them, AdaBoost most accurate, an AUC value 0.99.
Язык: Английский
Процитировано
5International Journal of Disaster Risk Science, Год журнала: 2023, Номер 14(3), С. 475 - 487
Опубликована: Июнь 1, 2023
Abstract In contemporary cities, road collapse is one of the most common disasters. This study proposed a framework for assessing risk urban collapse. The first established indicator system that combined environmental and anthropogenic factors, such as soil type, pipeline, construction, well other indicators. Second, an oversampling technique was used to create dataset. then constructed trained convolutional neural network (CNN)-based model assessment. experimental results show CNN (accuracy: 0.97, average recall: 0.91) outperformed models. contribution analysis revealed distance between construction site (contribution: 0.132) size 0.144) are significant factors contributing According natural breaks, map Foshan City, Guangdong Province, created, level divided into five categories. Nearly 3% roads in area at very high risk, 6% levels, with concentrated east southeast. produced by this can be utilized local authorities policymakers help maintain safety.
Язык: Английский
Процитировано
13Bulletin of Engineering Geology and the Environment, Год журнала: 2023, Номер 82(10)
Опубликована: Сен. 16, 2023
Язык: Английский
Процитировано
12Geoscience Frontiers, Год журнала: 2024, Номер 16(1), С. 101960 - 101960
Опубликована: Ноя. 1, 2024
Язык: Английский
Процитировано
4