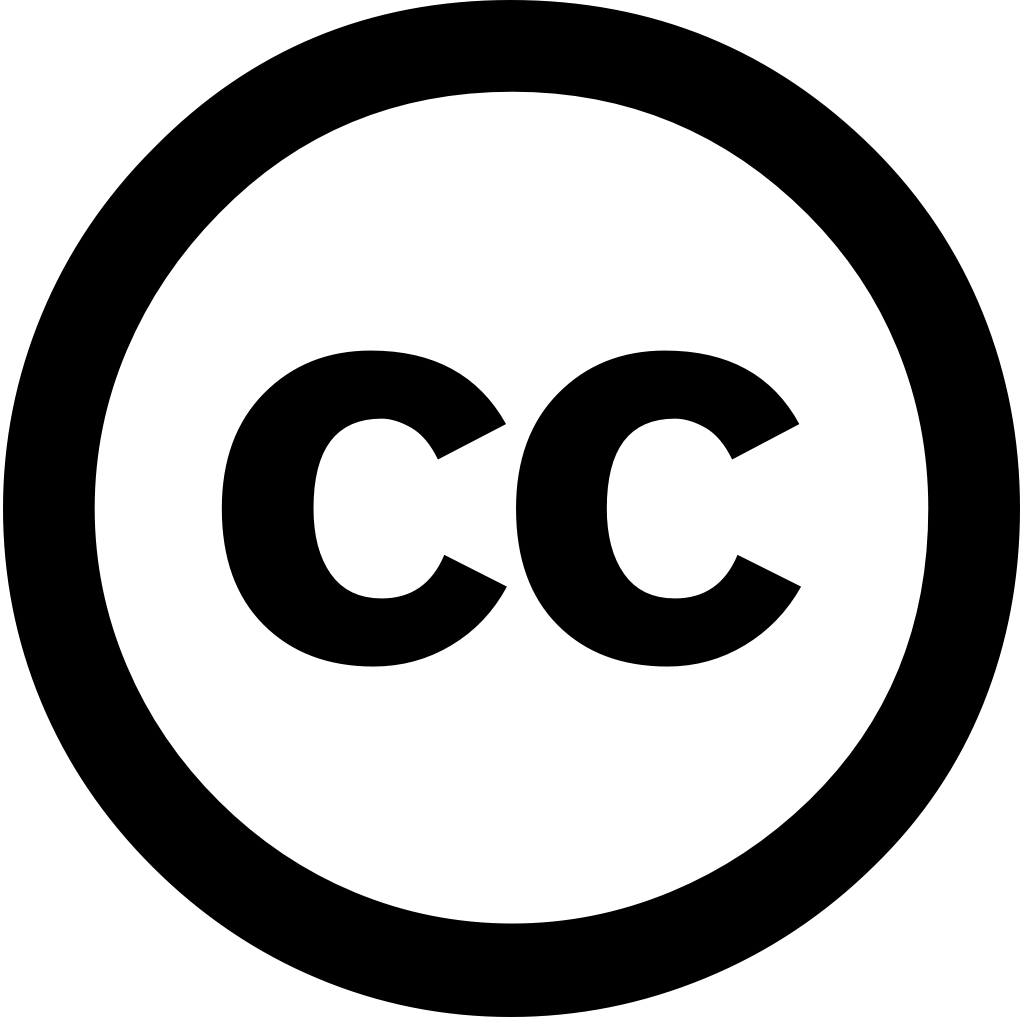
bioRxiv (Cold Spring Harbor Laboratory), Год журнала: 2025, Номер unknown
Опубликована: Март 6, 2025
Abstract The rapid expansion of three-dimensional (3D) biomolecular structure information, driven by breakthroughs in artificial intelligence/deep learning (AI/DL)-based predictions, has created an urgent need for scalable and efficient similarity search methods. Traditional alignment-based approaches, such as structural superposition tools, are computationally expensive challenging to scale with the vast number available macromolecular structures. Herein, we present a strategy designed navigate extensive repositories experimentally determined structures computed models predicted using AI/DL Our approach leverages protein language deep neural network architecture transform 3D into fixed-length vectors, enabling large-scale comparisons. Although trained predict TM-scores between single-domain structures, our model generalizes beyond domain level, accurately identifying full-length polypeptide chains multimeric assemblies. By integrating vector databases, method facilitates retrieval, addressing growing challenges posed expanding volume biostructure information.
Язык: Английский