Adaptive Toeplitz Convolution- enhanced Classifier for Anomaly Detection in ECG Big Data
Abstract
The
anomaly
detection
of
electrocardiogram
(ECG)
data
is
crucial
for
identifying
deviations
from
normal
heart
rhythm
patterns
and
providing
timely
interventions
high-risk
patients.
Various
autoencoder
(AE)
models
within
machine
learning
(ML)
have
been
proposed
this
task.
However,
these
often
do
not
explicitly
consider
the
specific
in
ECG
time
series,
thereby
impacting
their
efficiency.
In
contrast,
we
adopt
a
method
based
on
prior
knowledge
series
shapes,
employing
multi-stage
preprocessing,
adaptive
convolution
kernels,
Toeplitz
matrices
to
replace
encoding
part
AE.
This
approach
combines
inherent
features
with
symmetry
matrices,
effectively
extracting
signals
reducing
dimensionality.
Our
model
consistently
outperforms
state-of-the-art
detection,
achieving
an
overall
accuracy
exceeding
99.6%,
Precision
Area
Under
Receiver
Operating
Characteristic
Curve
(AUC)
reaching
99.8%,
Recall
peaking
at
99.9%.
Moreover,
runtime
significantly
reduced.
These
results
demonstrate
that
our
technique
detects
anomalies
through
automatic
feature
extraction
enhances
performance
ECG5000
dataset,
benchmark
collection
heartbeat
signals.
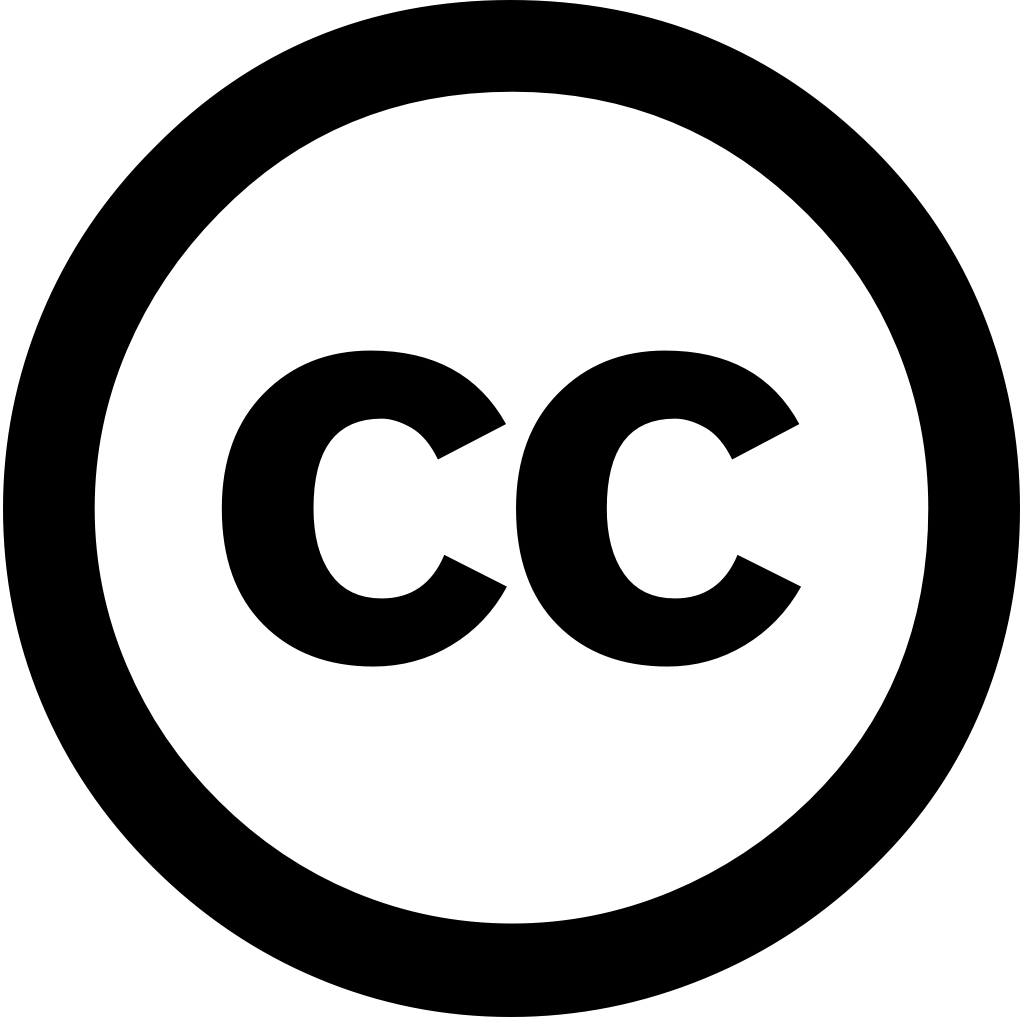
Research Square (Research Square), Год журнала: 2024, Номер unknown
Опубликована: Июль 30, 2024
Язык: Английский