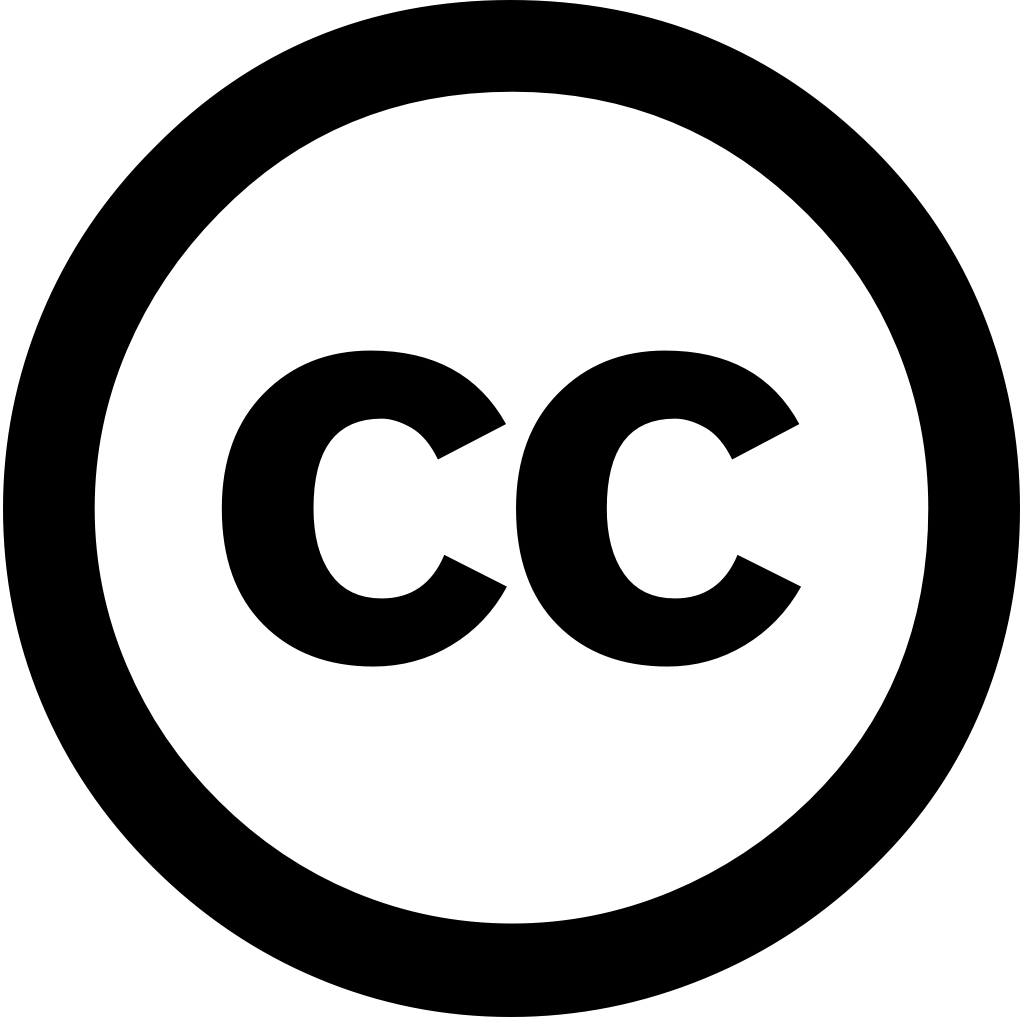
Artificial Intelligence Review, Год журнала: 2025, Номер 58(4)
Опубликована: Фев. 5, 2025
Язык: Английский
Artificial Intelligence Review, Год журнала: 2025, Номер 58(4)
Опубликована: Фев. 5, 2025
Язык: Английский
Computers in Biology and Medicine, Год журнала: 2024, Номер 179, С. 108803 - 108803
Опубликована: Июль 1, 2024
Язык: Английский
Процитировано
40Energy, Год журнала: 2024, Номер 294, С. 130726 - 130726
Опубликована: Фев. 16, 2024
Язык: Английский
Процитировано
36Knowledge-Based Systems, Год журнала: 2024, Номер 295, С. 111850 - 111850
Опубликована: Апрель 22, 2024
Язык: Английский
Процитировано
35Artificial Intelligence Review, Год журнала: 2024, Номер 57(5)
Опубликована: Апрель 24, 2024
Abstract Crayfish Optimization Algorithm (COA) is innovative and easy to implement, but the crayfish search efficiency decreases in later stage of algorithm, algorithm fall into local optimum. To solve these problems, this paper proposes an modified optimization (MCOA). Based on survival habits crayfish, MCOA environmental renewal mechanism that uses water quality factors guide seek a better environment. In addition, integrating learning strategy based ghost antagonism enhances its ability evade optimality. evaluate performance MCOA, tests were performed using IEEE CEC2020 benchmark function experiments conducted four constraint engineering problems feature selection problems. For constrained improved by 11.16%, 1.46%, 0.08% 0.24%, respectively, compared with COA. average fitness value accuracy are 55.23% 10.85%, respectively. shows solving complex spatial practical application The combination environment updating significantly improves MCOA. This discovery has important implications for development field optimization. Graphical
Язык: Английский
Процитировано
34Neural Computing and Applications, Год журнала: 2024, Номер 36(12), С. 6721 - 6740
Опубликована: Фев. 12, 2024
Язык: Английский
Процитировано
33Advanced Engineering Informatics, Год журнала: 2024, Номер 61, С. 102464 - 102464
Опубликована: Март 15, 2024
Язык: Английский
Процитировано
31Neurocomputing, Год журнала: 2024, Номер 607, С. 128289 - 128289
Опубликована: Авг. 3, 2024
Язык: Английский
Процитировано
31Expert Systems with Applications, Год журнала: 2024, Номер 252, С. 123734 - 123734
Опубликована: Март 22, 2024
Язык: Английский
Процитировано
25Energy Reports, Год журнала: 2024, Номер 11, С. 4423 - 4451
Опубликована: Апрель 18, 2024
In recent times, the landscape of power systems has undergone significant evolution, particularly with integration diverse renewable energy sources (RESs). This advancement presents an invaluable opportunity to enhance efficiency in modern grid, primarily by bolstering role stochastic RESs. The challenge lies optimal flow (OPF), a multifaceted and non-linear optimization that grows more complex inclusion RESs aims optimize allocation system resources minimize operational cost while maintaining stability security system. Addressing this, current study introduces innovative approach, Multi-Objective RIME (MORIME) algorithm. Drawing inspiration from physical phenomenon rime-ice, called RIME, MORIME seeks effectively tackle OPF issues. algorithm enhances solution accuracy smartly dividing non-dominated sorting crowding distance mechanism. proposed model incorporates three types RESs: solar photovoltaic, wind small-scale hydropower units. While uncertainties speed irradiation are managed through Monte Carlo simulations, small hydro unit is considered constant source. efficacy tested on IEEE 30 bus results indicate method identifies for multi-objective problem satisfying constraints, thereby proving its effectiveness superiority over MOWOA, MOGWO, MOALO, MOMRFO MOAGDE terms Hyper Volume (HV) reciprocal Pareto Sets Proximity (1/PSP) metrices. source code available at: https://github.com/kanak02/MORIME
Язык: Английский
Процитировано
25Energy, Год журнала: 2024, Номер 305, С. 132228 - 132228
Опубликована: Окт. 1, 2024
Язык: Английский
Процитировано
23