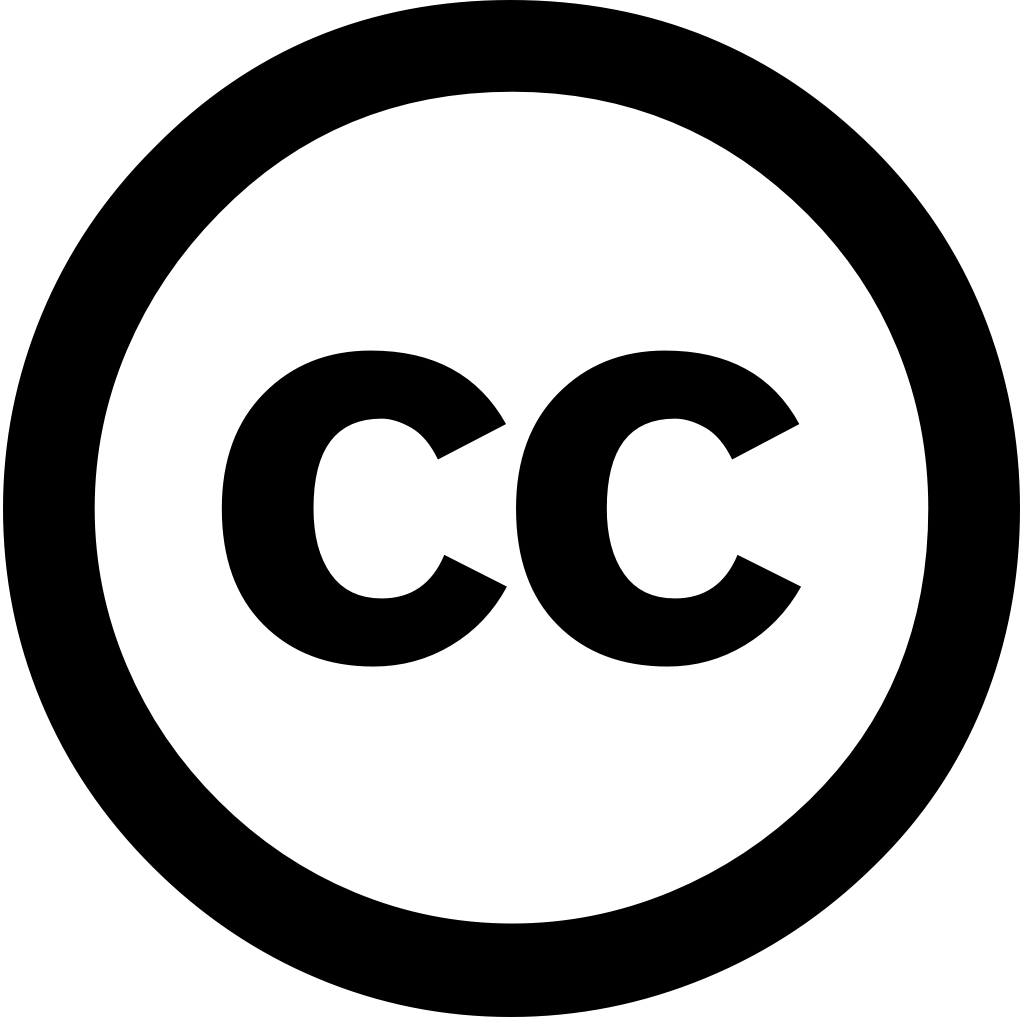
Scientific Reports, Год журнала: 2025, Номер 15(1)
Опубликована: Апрель 18, 2025
Язык: Английский
Scientific Reports, Год журнала: 2025, Номер 15(1)
Опубликована: Апрель 18, 2025
Язык: Английский
Chaos Theory and Applications, Год журнала: 2025, Номер 7(1), С. 50 - 60
Опубликована: Янв. 29, 2025
Ischemic stroke, a widespread neurological condition with substantial mortality rate, necessitates accurate delineation of affected regions to enable proper evaluation patient outcomes. However, such precision is complicated by factors like variable lesion sizes, noise interference, and the overlapping intensity characteristics different tissue structures. This research addresses these issues focusing on segmentation Diffusion Weighted Imaging (DWI) scans from ISLES 2022 dataset conducting comparative assessment three advanced deep learning models: U-Net framework, its U-Net++ extension, Attention U-Net. Applying consistent criteria specifically, Intersection over Union (IoU), Dice Similarity Coefficient (DSC), recall emerged as superior choice, establishing record high values for IoU (0.8223) DSC (0.9021). Although achieved commendable recall, performance lagged behind that in other critical measures. These findings underscore value integrating attention mechanisms achieve more precise segmentation. Moreover, they highlight model reliable candidate medical imaging tasks where both accuracy efficiency hold paramount importance, while U Net Net++ may still prove suitable certain niche scenarios.
Язык: Английский
Процитировано
1Neuroscience, Год журнала: 2025, Номер unknown
Опубликована: Апрель 1, 2025
Язык: Английский
Процитировано
0Scientific Reports, Год журнала: 2025, Номер 15(1)
Опубликована: Март 3, 2025
Skin cancer is the most dominant and critical method of cancer, which arises all over world. Its damaging effects can range from disfigurement to major medical expenditures even death if not analyzed preserved timely. Conventional models skin recognition require a complete physical examination by specialist, time-wasting in few cases. Computer-aided medicinal analytical methods have gained massive popularity due their efficiency effectiveness. This model assist dermatologists initial significant for early diagnosis. An automatic classification utilizing deep learning (DL) help doctors perceive kind lesion improve patient's health. The one hot topics research field, along with development DL structure. manuscript designs develops Detection Cancer Using an Ensemble Deep Learning Model Gray Wolf Optimization (DSC-EDLMGWO) method. proposed DSC-EDLMGWO relies on biomedical imaging. presented initially involves image preprocessing stage at two levels: contract enhancement using CLAHE noise removal wiener filter (WF) model. Furthermore, utilizes SE-DenseNet method, fusion squeeze-and-excitation (SE) module DenseNet extract features. For process, ensemble models, namely long short-term memory (LSTM) technique, extreme machine (ELM) model, stacked sparse denoising autoencoder (SSDA) employed. Finally, gray wolf optimization (GWO) optimally adjusts models' hyperparameter values, resulting more excellent performance. effectiveness approach evaluated benchmark database, outcomes measured across various performance metrics. experimental validation portrayed superior accuracy value 98.38% 98.17% under HAM10000 ISIC datasets other techniques.
Язык: Английский
Процитировано
0Scientific Reports, Год журнала: 2025, Номер 15(1)
Опубликована: Март 22, 2025
Abstract Vowel-based voice analysis is gaining attention as a potential non-invasive tool for COPD classification, offering insights into phonatory function. The growing need data has necessitated the adoption of various techniques, including segmentation, to augment existing datasets training comprehensive Machine Learning (ML) modelsThis study aims investigate possible effects segmentation utterance vowel "a" on performance ML classifiers CatBoost (CB), Random Forest (RF), and Support Vector (SVM). This research involves individual models using three distinct dataset constructions: full-sequence, segment-wise, group-wise, derived from which consists 1058 recordings belonging 48 participants. approach comprehensively analyzes how each categorization impacts model's results. A nested cross-validation (nCV) was implemented with grid search hyperparameter optimization. rigorous methodology employed minimize overfitting risks maximize model performance. Compared full-sequence dataset, findings indicate that second segment yielded higher results within four-segment category. Specifically, CB achieved superior accuracy, attaining 97.8% 84.6% validation test sets, respectively. same category also demonstrated best balance regarding true positive rate (TPR) negative (TNR), making it most clinically effective choice. These suggest time-sensitive properties in production are important classification can aid capturing these properties. Despite promising results, size demographic homogeneity limit generalizability, highlighting areas future research. Trial registration registered clinicaltrials.gov ID: NCT06160674.
Язык: Английский
Процитировано
0Scientific Reports, Год журнала: 2025, Номер 15(1)
Опубликована: Март 29, 2025
In response to the relentless mutation of coronavirus disease, current artificial intelligence algorithms for automated diagnosis COVID-19 via CT imaging exhibit suboptimal accuracy and efficiency. This manuscript proposes a multi-objective optimization algorithm (MOAOA) enhance BiLSTM model diagnosis. The proposed approach involves configuring several hyperparameters bidirectional long short-term memory (BiLSTM), optimized using MOAOA intelligent algorithm, subsequently validated on publicly accessible medical datasets. Remarkably, our achieves an impressive 95.32% 95.09% specificity. Comparative analysis with state-of-the-art techniques demonstrates that significantly enhances accuracy, efficiency, other performance metrics, yielding superior results.
Язык: Английский
Процитировано
0Food and Bioprocess Technology, Год журнала: 2025, Номер unknown
Опубликована: Апрель 7, 2025
Язык: Английский
Процитировано
0Scientific Reports, Год журнала: 2025, Номер 15(1)
Опубликована: Апрель 16, 2025
Abstract The importance of gastric cancer (GC) and the role deep learning techniques in categorizing GC histopathology images have recently increased. Identifying drawbacks traditional models, including lack interpretability, inability to capture complex patterns, adaptability, sensitivity noise. A multi-channel attention mechanism-based framework is proposed that can overcome limitations conventional models by dynamically focusing on relevant features, enhancing extraction, capturing relationships medical data. uses three different mechanism channels convolutional neural networks extract multichannel features during classification process. framework’s strong performance confirmed competitive experiments conducted a publicly available Gastric Histopathology Sub-size Image Database, which yielded remarkable accuracies 99.07% 98.48% validation testing sets, respectively. Additionally, HCRF dataset, achieved high accuracy 99.84% 99.65% effectiveness interchangeability are further ablation experiments, highlighting histopathological image tasks. This offers an advanced pragmatic artificial intelligence solution addresses challenges posed unique characteristics for intricate analysis. approach engineering demonstrates significant potential diagnostic precision achieving treatment outcomes.
Язык: Английский
Процитировано
0Scientific Reports, Год журнала: 2025, Номер 15(1)
Опубликована: Апрель 18, 2025
Язык: Английский
Процитировано
0