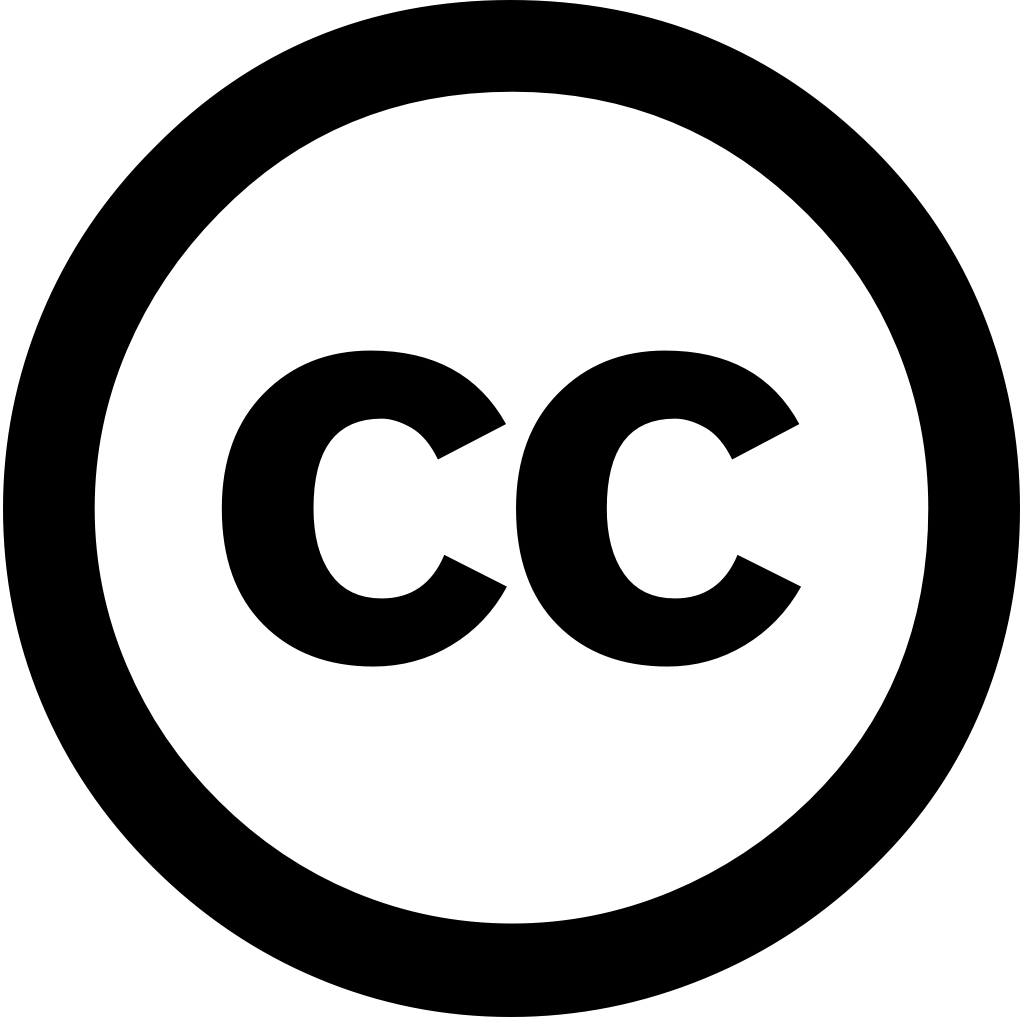
Energy Advances, Год журнала: 2024, Номер unknown
Опубликована: Янв. 1, 2024
A novel machine learning approach for supercapacitor performance prediction of graphene oxide nano-ring-based electrode materials.
Язык: Английский
Energy Advances, Год журнала: 2024, Номер unknown
Опубликована: Янв. 1, 2024
A novel machine learning approach for supercapacitor performance prediction of graphene oxide nano-ring-based electrode materials.
Язык: Английский
Journal of Energy Storage, Год журнала: 2025, Номер 111, С. 115371 - 115371
Опубликована: Янв. 18, 2025
Язык: Английский
Процитировано
1Electrochimica Acta, Год журнала: 2025, Номер unknown, С. 145573 - 145573
Опубликована: Янв. 1, 2025
Язык: Английский
Процитировано
0Proceedings of the Institution of Mechanical Engineers Part E Journal of Process Mechanical Engineering, Год журнала: 2025, Номер unknown
Опубликована: Янв. 19, 2025
Machine learning (ML) has become a potential alternate in material science to process complex datasets containing multiple inputs/outputs and predict the properties of materials using algorithms statistical models. The mechanical PLA/brass infill composites fabricated by fused deposition modelling based additive manufacturing were analysed this work. Tensile strength, flexural strength impact are three output parameters considered for study input density, nozzle temperature, layer thickness printing speed. Strength increased with rise density temperature while decreased speed, thickness. Six ML namely Artificial Neural Networks (ANNs), Decision Tree (DT), Random Forest (RF), Ada Boost (AB), Gradient Boost, Support Vector (SVM) employed evaluate strength. Effectiveness models was studied tools coefficient determination, mean square error absolute error. ANN model exhibited high accuracy AB displayed least predicting Scanning electron morphology deployed fracture profile composites. squared (MSE) is higher (1.496) MSE value obtained (0.131) lowest (MAE) (0.209) highest MAE (0.812) tensile boost (5.603) (2.376) DT (0.773) gradient (2.200)
Язык: Английский
Процитировано
0Elsevier eBooks, Год журнала: 2025, Номер unknown, С. 197 - 228
Опубликована: Янв. 1, 2025
Язык: Английский
Процитировано
0Journal of Solid State Electrochemistry, Год журнала: 2024, Номер unknown
Опубликована: Июнь 1, 2024
Язык: Английский
Процитировано
2Inorganic Chemistry Communications, Год журнала: 2024, Номер unknown, С. 113577 - 113577
Опубликована: Ноя. 1, 2024
Язык: Английский
Процитировано
2Electronics, Год журнала: 2024, Номер 13(12), С. 2278 - 2278
Опубликована: Июнь 10, 2024
Detecting the crystal system of lithium-ion batteries is crucial for optimizing their performance and safety. Understanding arrangement atoms or ions within battery’s electrodes electrolyte allows improvements in energy density, cycling stability, safety features. This knowledge also guides material design fabrication techniques, driving advancements battery technology various applications. In this paper, a publicly available dataset was utilized to develop mathematical equations (MEs) using genetic programming symbolic classifier (GPSC) determine type structure Li-ion with high classification performance. The consists three different classes transformed into binary datasets one-versus-rest approach. Since target variable each variation imbalanced, several oversampling techniques were employed achieve balanced variations. GPSC trained on these variations five-fold cross-validation (5FCV) process, optimal hyperparameter values searched random value search (RHVS) method. goal find combination highest After obtaining MEs performance, they combined tested initial Based conducted investigation, ensemble could detect accuracy (1.0).
Язык: Английский
Процитировано
1Journal of Chemical Information and Modeling, Год журнала: 2024, Номер unknown
Опубликована: Дек. 12, 2024
Predicting the properties for unseen materials exclusively on basis of chemical formula before synthesis and characterization has advantages research resource planning. This can be achieved using suitable structure-free encoding machine learning methods, but additional processing decisions are required. In this study, we compare a variety encodings algorithms to predict structure/property relationships battery materials. It was found that physical units used measure property labels have an important impact predictive ability models, regardless computational approach. Property with respect weight give excellent performance, volume cannot predicted confidence only information, even when underlying characteristics same. These results contrast previous studies unsupervised classification, where excelled, highlight how structural features or represented plays role in models.
Язык: Английский
Процитировано
1Опубликована: Апрель 19, 2024
Язык: Английский
Процитировано
0Energy Advances, Год журнала: 2024, Номер unknown
Опубликована: Янв. 1, 2024
A novel machine learning approach for supercapacitor performance prediction of graphene oxide nano-ring-based electrode materials.
Язык: Английский
Процитировано
0