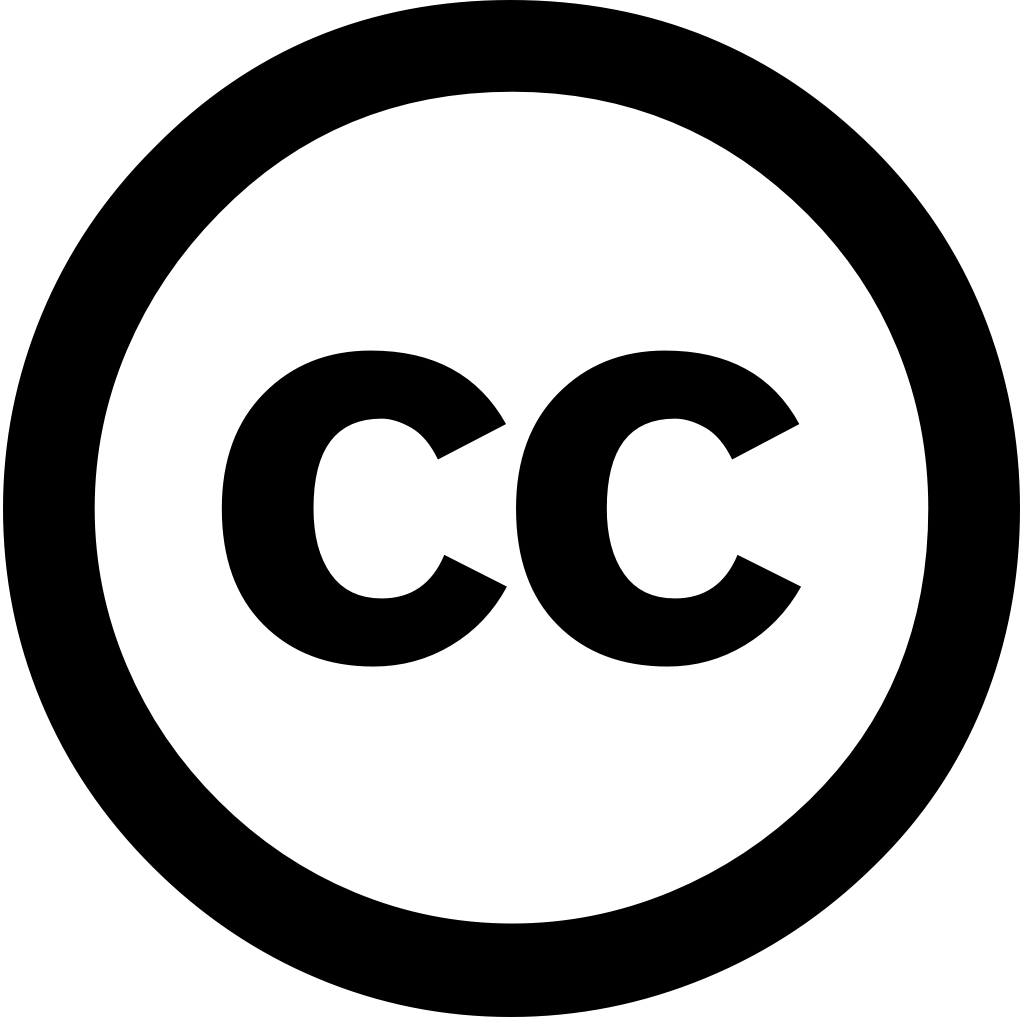
Sensors, Год журнала: 2025, Номер 25(5), С. 1293 - 1293
Опубликована: Фев. 20, 2025
Transformers have rapidly influenced research across various domains. With their superior capability to encode long sequences, they demonstrated exceptional performance, outperforming existing machine learning methods. There has been a rapid increase in the development of transformer-based models for EEG analysis. The high volumes recently published papers highlight need further studies exploring transformer architectures, key components, and employed particularly studies. This paper aims explore four major architectures: Time Series Transformer, Vision Graph Attention hybrid models, along with variants recent We categorize according most frequent applications motor imagery classification, emotion recognition, seizure detection. also highlights challenges applying transformers datasets reviews data augmentation transfer as potential solutions explored years. Finally, we provide summarized comparison reported results. hope this serves roadmap researchers interested employing architectures
Язык: Английский