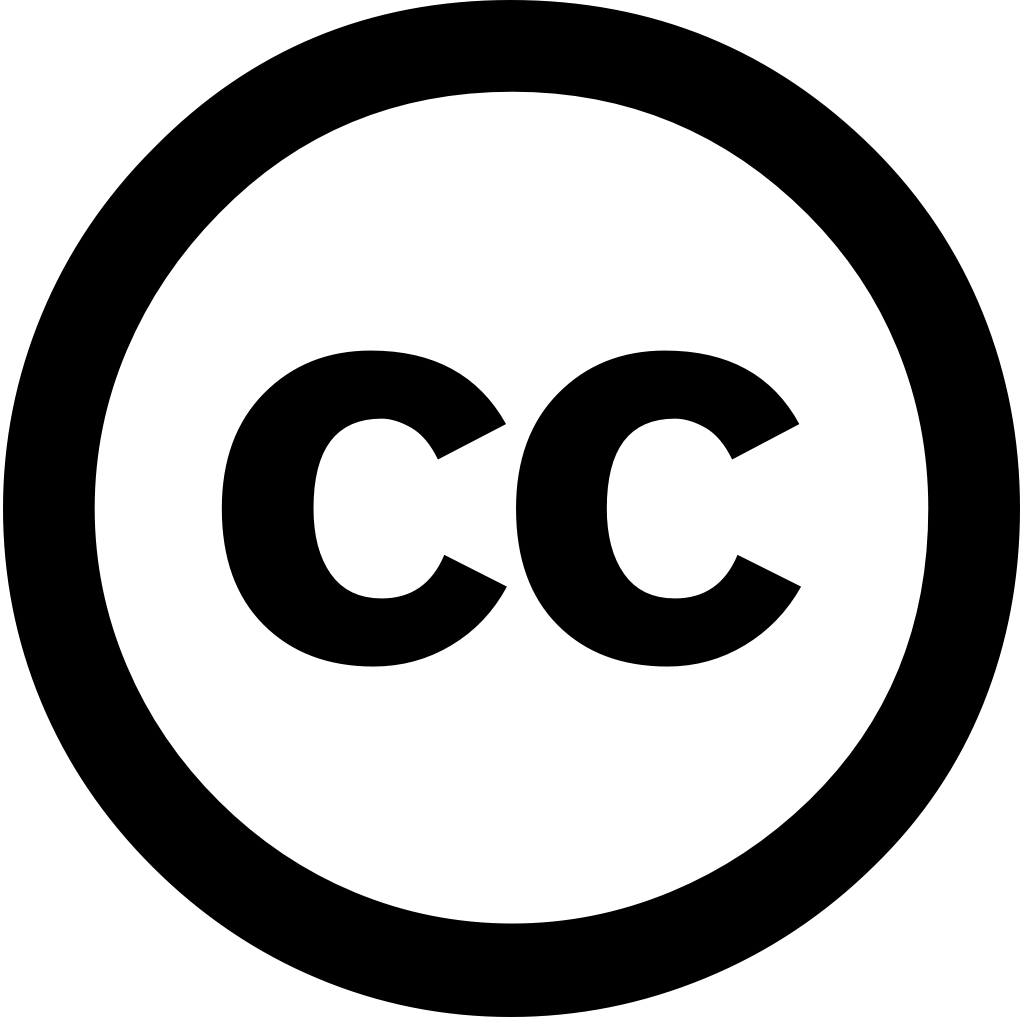
Remote Sensing, Год журнала: 2025, Номер 17(7), С. 1174 - 1174
Опубликована: Март 26, 2025
Thermokarst lakes are widely distributed on the Qinghai-Tibet Plateau (QTP). However, owing to lack of high-precision remote sensing imagery and difficulty in situ monitoring permafrost regions, quantifying changes distribution thermokarst is challenging. In this study, we used four machine learning methods—random forest (RF), gradient boosting decision tree (GBDT), classification regression (CART), support vector (SVM)—and combined various environmental factors assess from 2015 2020 via Google Earth Engine (GEE). The results indicated that RF model performed optimally extraction lakes, followed by GBDT, CART, SVM. From 2020, number increased 52%, area expanded 1.6 times. A large proportion STK (with areas less than or equal 1000 m2) gradually developed into MTK between 10,000 central part QTP. Additionally, located primarily at elevations 4000 5000 m, with slopes ranging 0 5°, sand content approximately 65%. normalized difference water index (NDWI) enhanced vegetation (EVI) were most favourable for lake extraction. provide a scientific reference assessment prediction dynamic QTP future, which will have important significance studies carbon processes alpine ecosystems.
Язык: Английский