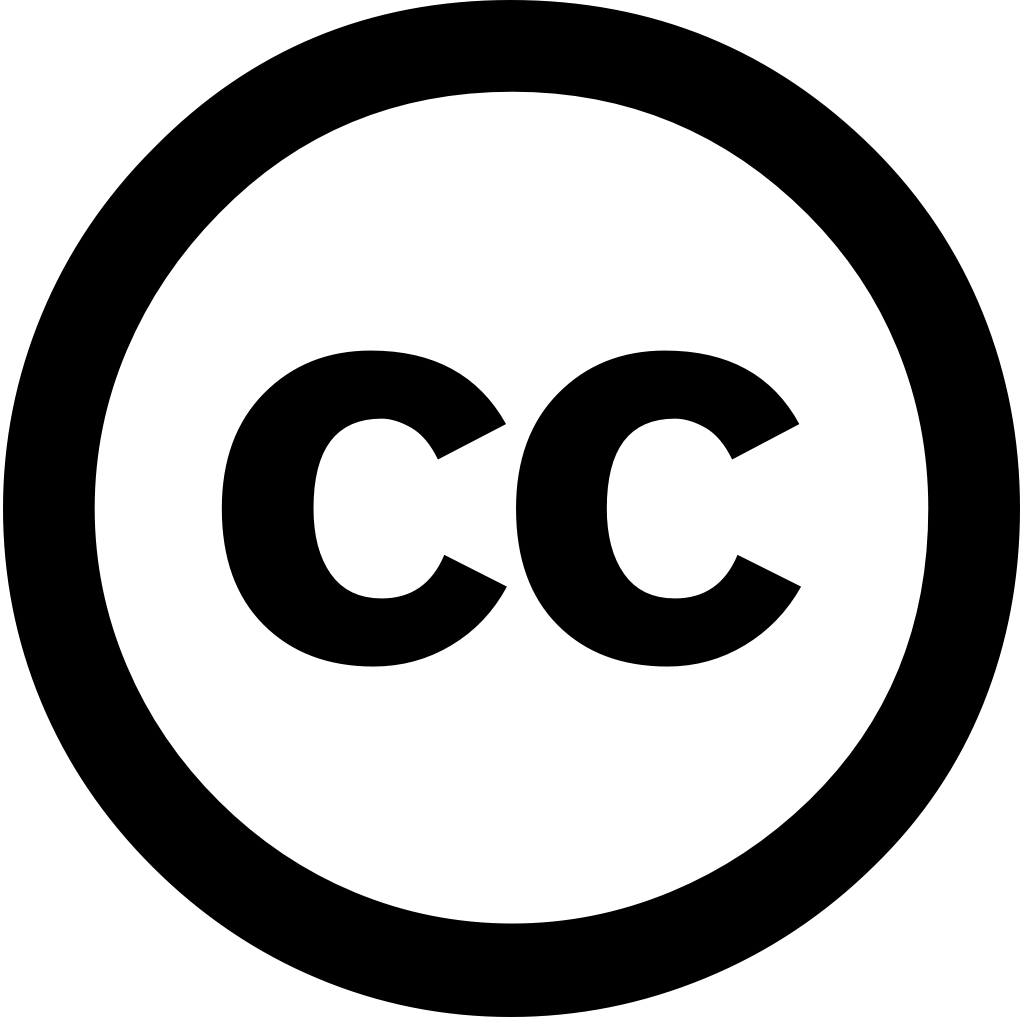
Frontiers in Environmental Science, Год журнала: 2024, Номер 12
Опубликована: Ноя. 26, 2024
China’s total carbon emissions account for one-third of the world’s total. How to reach peak by 2030 and achieve neutrality 2060 is an important policy orientation at present. Therefore, it great significance analyze characteristics driving factors temporal spatial evolution on basis effective calculation prediction in various provinces promoting high-quality economic development realizing emission reduction. Based energy consumption data 30 China from 2000 2021, this paper calculates predicts 2035 based ARIMA model BP neural network model, uses ArcGIS standard elliptic difference visually characteristics, further LMDI decompose affecting emissions. The results show that: (1) From 2035, increased year year, but growth rate gradually decreased; structure “secondary industry > residents’ life tertiary primary industry”, secondary faster, while change trend smaller; (2) distribution presents a typical pattern “eastern central western” “northern southern”, centers tend move northwest; (3) regions with high level digital economy, advanced industrial new quality productivity have relatively less emissions, which has significant group effect; (4) intensity effect main factor continuous per capita GDP are restraining effects population size small. research conclusion, puts forward some suggestions structure, economy.
Язык: Английский