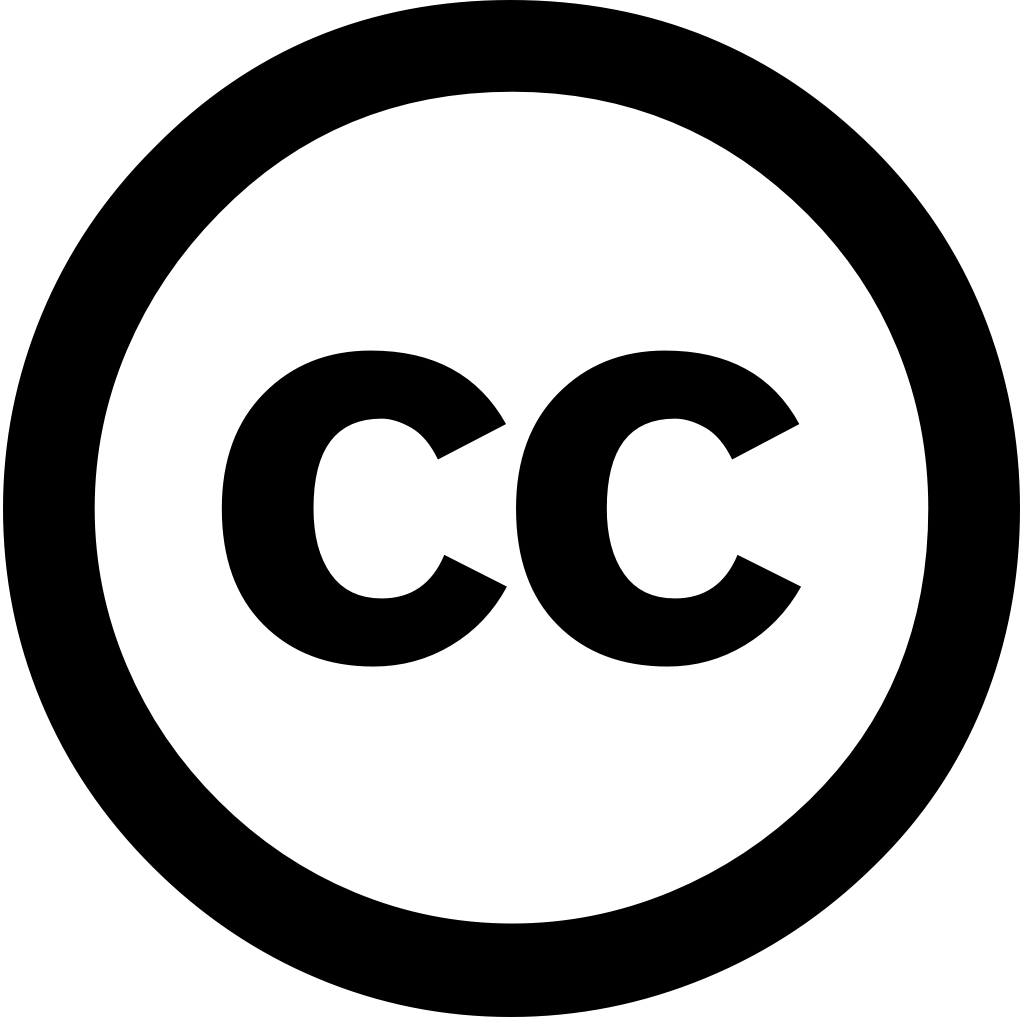
ACS Energy Letters, Год журнала: 2025, Номер unknown, С. 2318 - 2340
Опубликована: Апрель 15, 2025
ACS Energy Letters, Год журнала: 2025, Номер unknown, С. 2318 - 2340
Опубликована: Апрель 15, 2025
IEEE Access, Год журнала: 2024, Номер 12, С. 77903 - 77931
Опубликована: Янв. 1, 2024
Lithium-ion (Li-ion) batteries are being used in electric vehicles to reduce the reliance on fossil fuels due their high energy density, design flexibility, and efficiency compared other battery technologies. However, they undergo complex nonlinear degradation performance declines when abused, making reliability crucial for effective vehicle performance. This survey paper presents a comprehensive review of state-of-the-art assessments vehicles. First, operating principle Li-ion batteries, patterns, models briefly discussed. Afterwards, detailed qualitative quantitative approaches. The approach encompasses failure modes mechanisms effects analysis, X-ray computed tomography, scanning electron microscopy. In contrast, approaches involve multiphysics modelling, electrochemical impedance spectroscopy, incremental capacity differential voltage machine learning, transfer learning. Each technique is examined terms its principles, advantages, limitations, applicability Comparative analysis reveals that methods primarily early stages assess potential risks post-mortem laboratory, while techniques such as learning offer real-time prognostic health management anomaly prevention. Also, tend be more cost-effective counterparts. consolidating through standardization testing protocols, real-world data integration, controller area network use, policy regulation highlighted guide further research.
Язык: Английский
Процитировано
7Reliability Engineering & System Safety, Год журнала: 2024, Номер 252, С. 110471 - 110471
Опубликована: Авг. 27, 2024
Язык: Английский
Процитировано
7Reliability Engineering & System Safety, Год журнала: 2024, Номер 251, С. 110387 - 110387
Опубликована: Июль 29, 2024
Язык: Английский
Процитировано
6Journal of Energy Storage, Год журнала: 2023, Номер 75, С. 109690 - 109690
Опубликована: Ноя. 19, 2023
Язык: Английский
Процитировано
14Journal of Energy Storage, Год журнала: 2024, Номер 101, С. 113827 - 113827
Опубликована: Сен. 25, 2024
Язык: Английский
Процитировано
5iScience, Год журнала: 2024, Номер 27(6), С. 109980 - 109980
Опубликована: Май 20, 2024
Existing methods for interpreting Electrochemical Impedance Spectroscopy data involve various models, which face significant challenges in parameterization and physical interpretation fail to comprehensively reflect the electrochemical behavior within batteries. To address these issues, this study proposes a Temperature-Controlled Second-Order R-CPE Equivalent Circuit Model capture non-ideal capacitive characteristics of electrode surfaces. Additionally, employs Copula based Joint Mixture Weibull multi-output Gaussian Process Regression enhance precision capturing distribution battery parameters predict SoH curves. Experimental validation shows that model used article has an average RMSE error 8.5%, prediction curve after 100th cycle can achieve 9.2%. These findings provide insightful implications understanding complexities parameter interdependencies aging process, offering robust framework future research diagnostics.
Язык: Английский
Процитировано
4Reliability Engineering & System Safety, Год журнала: 2024, Номер 251, С. 110395 - 110395
Опубликована: Июль 23, 2024
Язык: Английский
Процитировано
4Journal of The Electrochemical Society, Год журнала: 2025, Номер 172(2), С. 020503 - 020503
Опубликована: Янв. 23, 2025
Accurately estimating the state of health (SOH) lithium-ion batteries is essential for ensuring stability and safety battery. Although hybrid neural network model demonstrates strong performance in SOH, degradation becomes a significant issue as depth increases, potentially undermining accuracy estimation. This paper presents estimation with residual connections to address degradation. First, utilizes combination convolutional networks an attention mechanism automatically extract feature information highly correlated SOH from partial charging data batteries. Subsequently, multi-layer gated recurrent unit (GRU) employed capture temporal within extracted features. To that arises stacking multiple layers networks, are incorporated into GRUs, mitigating accumulation errors deep networks. Finally, three distinct datasets validate proposed model. The experimental results demonstrate exhibits average mean absolute error root square less than 1.8% on both these datasets.
Язык: Английский
Процитировано
0Journal of Energy Storage, Год журнала: 2025, Номер 112, С. 115497 - 115497
Опубликована: Янв. 24, 2025
Язык: Английский
Процитировано
0Energy, Год журнала: 2025, Номер unknown, С. 134992 - 134992
Опубликована: Фев. 1, 2025
Язык: Английский
Процитировано
0