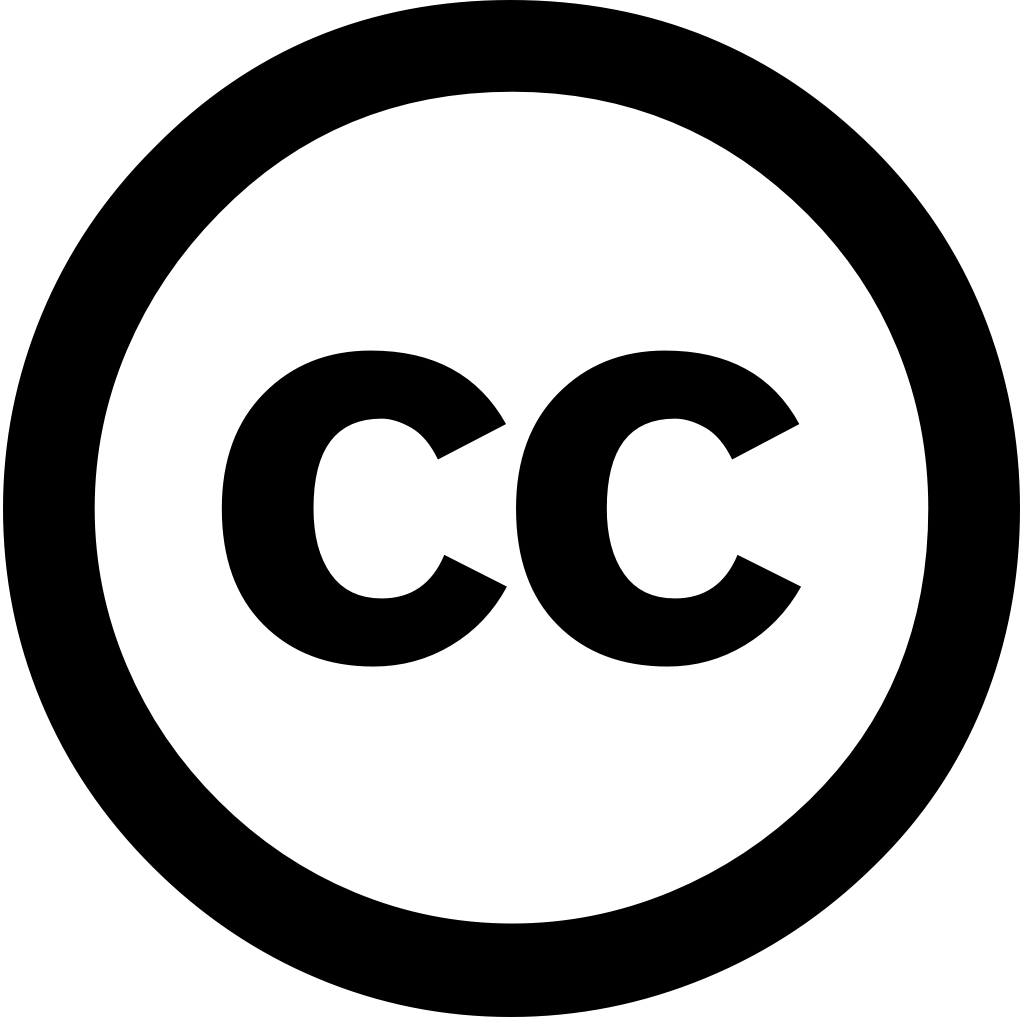
Deleted Journal, Год журнала: 2025, Номер 7(5)
Опубликована: Май 9, 2025
Язык: Английский
Deleted Journal, Год журнала: 2025, Номер 7(5)
Опубликована: Май 9, 2025
Язык: Английский
Journal of Cleaner Production, Год журнала: 2024, Номер 444, С. 141035 - 141035
Опубликована: Фев. 8, 2024
Язык: Английский
Процитировано
37Cement and Concrete Composites, Год журнала: 2024, Номер 148, С. 105488 - 105488
Опубликована: Фев. 24, 2024
Язык: Английский
Процитировано
21Journal of Cleaner Production, Год журнала: 2025, Номер unknown, С. 145127 - 145127
Опубликована: Фев. 1, 2025
Язык: Английский
Процитировано
2Results in Engineering, Год журнала: 2023, Номер 17, С. 100973 - 100973
Опубликована: Фев. 25, 2023
The most often utilized material in construction is concrete. High plasticity, good economy, safety, and exceptional durability are a few of its characteristics. Concrete type structural that needs to be strong enough withstand different loads. compressive strength the concrete members crucial mechanical characteristic because brittleness. Furthermore, with ternary blended cementitious materials sophisticated composite material. present study explores binary mixes silica fume, ceramic powder, bagasse ash, alccofine, determine flexural strength. Results compression tests show mixes, including ultra-fine have higher impact additional on surface morphology was examined using scanning electron microscopy various mixes. This investigates linear regression, KNearest Neighbors (KNN), Bayesian-optimized extreme gradient boosting estimate (BO-XGBoost). Using coefficient determination (R2), mean absolute error (MAE), square (MSE), prediction results further validated. In comparison, regression BO-XGBoost models high accuracy towards outcome as indicated by R2 value equal 0.883 0.880, respectively, while for KNN 0.736. Additionally, normalized feature importance included determining input variables significantly influenced sensitivity model indicates CaO SiO2 shows significant predict
Язык: Английский
Процитировано
41Structures, Год журнала: 2024, Номер 61, С. 106098 - 106098
Опубликована: Фев. 29, 2024
Язык: Английский
Процитировано
11Case Studies in Chemical and Environmental Engineering, Год журнала: 2025, Номер 11, С. 101128 - 101128
Опубликована: Янв. 31, 2025
Язык: Английский
Процитировано
1Results in Engineering, Год журнала: 2023, Номер 18, С. 101024 - 101024
Опубликована: Март 20, 2023
The rheological behavior of the phosphoric acid slurry, during production process, strongly depends on its dynamic viscosity. Controlling this property limits P2O5 losses, minimizes energy consumption and ensures optimal flow conditions. Thus, reliable simulation tools predicting viscosity are needed for analysis process optimization. To end, three machine learning (ML) methods: single-layer artificial neural network (ANN), gradient boosting (GB) random forest (RF), were tested using 456 data at different solid content, shear rate temperature, obtained from industry. performance these models was evaluated compared diverse precision metrics. GB has shown to be outperforming model with determination coefficient greater than 99%, Root Mean Squared Error lower 0.750, both training validation datasets. Based importance explanatory variables, all agree large effect content viscosity, followed by rate, then temperature. relative partial dependence diagram made it possible deduce operating intervals pulp fed reactor, leading suspension level attack maturation units.
Язык: Английский
Процитировано
19Construction and Building Materials, Год журнала: 2023, Номер 405, С. 133299 - 133299
Опубликована: Сен. 16, 2023
Язык: Английский
Процитировано
19Results in Engineering, Год журнала: 2023, Номер 20, С. 101585 - 101585
Опубликована: Ноя. 14, 2023
Machine learning is one effective way of increasing the accuracy sediment transport models. captures patterns in sequence both structured and unstructured data uses it for forecasting. In this research, different regression models were train to forecast using 8 years measured collected Sg. Linggui suspended station. Data from scenarios used where each scenario indicates number lags. Seven models, namely, Linear Regression, Regression Trees, Support Vector Machines, Gaussian Process Kernel Approximation, Ensemble Neural Network trained compared. The evaluated Root Mean Square Error (RMSE) Coefficient Determination (R2). best-performing two types chosen they tested test find Relative Percentage (RPE) predicted data. Exponential model performs much better than other terms RMSE R2 values. When exponential all 3 are compared, seems have a better-performing but only by very small margin, after testing data, result shows has less RPE compared Hence, can be deduced that gaussian process overall RSME, R2, RPE.
Язык: Английский
Процитировано
19Results in Engineering, Год журнала: 2024, Номер 21, С. 101810 - 101810
Опубликована: Янв. 22, 2024
An accurate estimation of local scour depth around piles group is inevitably essential to provide stability marine structures. Over the past decades, many investigations have been made understand scouring process at exposed waves for field and experimental scales. This study aims predict wave-induced by various robust Data Driven Models (DDMs) Machine Learning (MLMs) developed classification evolutionary concepts: Model Tree (MT), Evolutionary Polynomial Regression (EPR), Multivariate Adaptive Spline (MARS), Gene-Expression Programming (GEP). From relevant literature, 125 data series were employed empirical relationships predictions. The raw variables related bed sediment, pile configuration, geometry, approaching flow, wave characteristics. Non-dimensional parameters obtained through Buckingham theorem in order control depth. In this way, ratio spacing between diameter (G/D), sediment number (Ns), arrangement (ratio parallel flow; m; normal n), Shields parameter (θ), Keulegan-Carpenter (KC) considered. training testing stages Artificial Intelligence (AIMs), it was found that regression equation given MARS model provided better performance (e.g., Correlation Coefficient [R] = 0.9297, Root Mean Square Error [RMSE] 0.3489, Scatter Index [SI] 0.2765) than other AI models' efficiency. Additionally, models assessed ranges dimensionless (i.e., G/D, Ns, θ) versus KC variation. had best G/D 0–1 < 10 (R 0.9917 RMSE 0.2198) 0.4≤θ 0.5 15 25 whereas EPR promising efficiency its highest level Ns 1–3 ≤ 0.9941 0.0771) models. For sensitivity analysis, mutation rate chromosomes are examined GEP model, K-fold algebraic terms model. Furthermore, ranking analysis indicated (Ranking Performance [RPI] 0.7348) followed (0.3412), M5MT (0.2999), (0.2848).
Язык: Английский
Процитировано
8