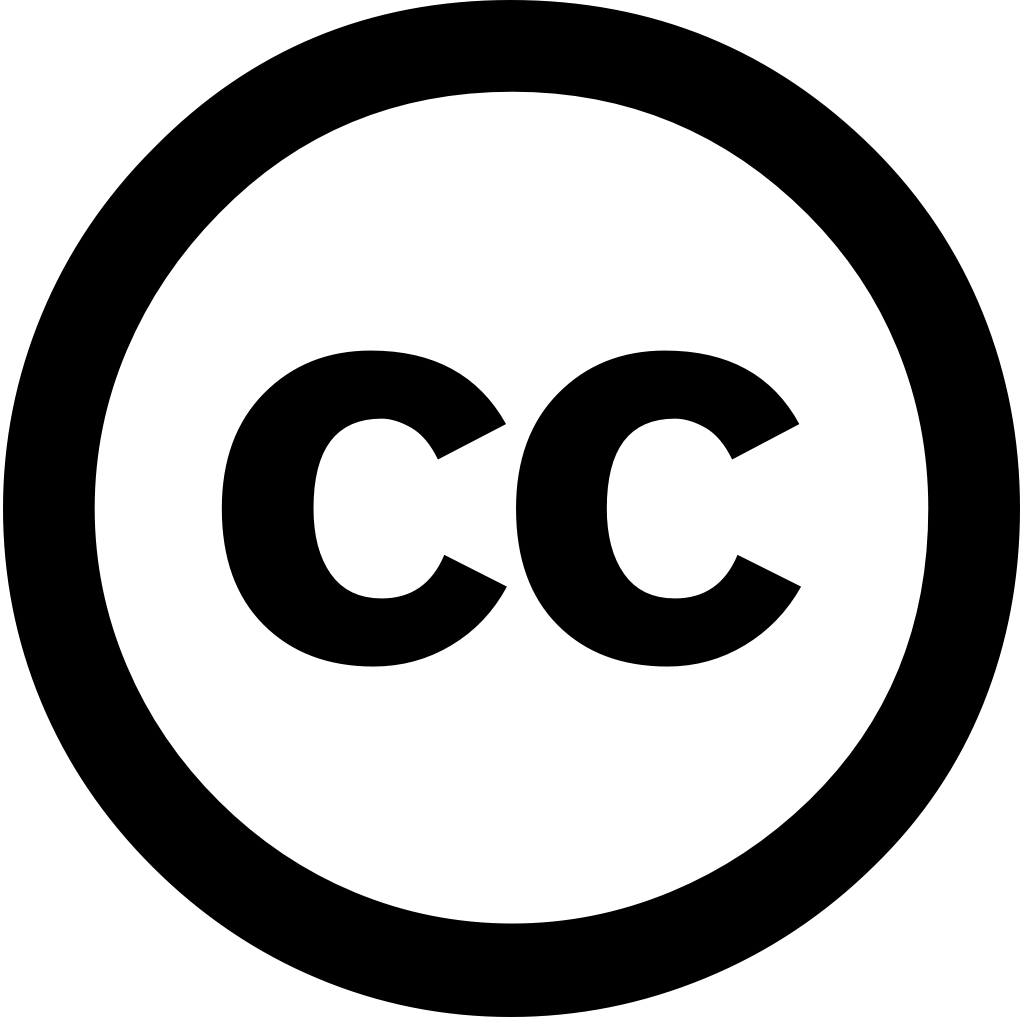
Hygiene and Environmental Health Advances, Год журнала: 2024, Номер unknown, С. 100114 - 100114
Опубликована: Окт. 1, 2024
Язык: Английский
Hygiene and Environmental Health Advances, Год журнала: 2024, Номер unknown, С. 100114 - 100114
Опубликована: Окт. 1, 2024
Язык: Английский
Journal of environmental chemical engineering, Год журнала: 2020, Номер 9(1), С. 104599 - 104599
Опубликована: Окт. 18, 2020
Язык: Английский
Процитировано
276Chemosphere, Год журнала: 2021, Номер 276, С. 130265 - 130265
Опубликована: Март 18, 2021
Язык: Английский
Процитировано
249Symmetry, Год журнала: 2020, Номер 12(6), С. 1022 - 1022
Опубликована: Июнь 17, 2020
Predicting and mapping fire susceptibility is a top research priority in fire-prone forests worldwide. This study evaluates the abilities of Bayes Network (BN), Naïve (NB), Decision Tree (DT), Multivariate Logistic Regression (MLP) machine learning methods for prediction across Pu Mat National Park, Nghe An Province, Vietnam. The modeling methodology was formulated based on processing information from 57 historical fires set nine spatially explicit explanatory variables, namely elevation, slope degree, aspect, average annual temperate, drought index, river density, land cover, distance roads residential areas. Using area under receiver operating characteristic curve (AUC) seven other performance metrics, models were validated terms their to elucidate general behaviors Park predict future fires. Despite few differences between AUC values, BN model with an value 0.96 dominant over predicting second best DT (AUC = 0.94), followed by NB 0.939), MLR 0.937) models. Our robust analysis demonstrated that these are sufficiently response training validation datasets change. Further, results revealed moderate high levels susceptibilities associated ~19% where human activities numerous. resultant maps provide basis developing more efficient fire-fighting strategies reorganizing policies favor sustainable management forest resources.
Язык: Английский
Процитировано
230Applied Water Science, Год журнала: 2021, Номер 11(12)
Опубликована: Ноя. 6, 2021
Abstract Groundwater quality appraisal is one of the most crucial tasks to ensure safe drinking water sources. Concurrently, a index (WQI) requires some parameters. Conventionally, WQI computation consumes time and often found with various errors during subindex calculation. To this end, 8 artificial intelligence algorithms, e.g., multilinear regression (MLR), random forest (RF), M5P tree (M5P), subspace (RSS), additive (AR), neural network (ANN), support vector (SVR), locally weighted linear (LWLR), were employed generate prediction in Illizi region, southeast Algeria. Using best subset regression, 12 different input combinations developed strategy work was based on two scenarios. The first scenario aims reduce consumption computation, where all parameters used as inputs. second intends show variation critical cases when necessary analyses are unavailable, whereas inputs reduced sensitivity analysis. models appraised using several statistical metrics including correlation coefficient (R), mean absolute error (MAE), root square (RMSE), relative (RAE), (RRSE). results reveal that TDS TH key drivers influencing study area. comparison performance evaluation metric shows MLR model has higher accuracy compared other terms 1, 1.4572*10–08, 2.1418*10–08, 1.2573*10–10%, 3.1708*10–08% for R, MAE, RMSE, RAE, RRSE, respectively. executed less rate by RF 0.9984, 1.9942, 3.2488, 4.693, 5.9642 outcomes paper would be interest planners improving sustainable management plans groundwater resources.
Язык: Английский
Процитировано
201Journal of Water Process Engineering, Год журнала: 2022, Номер 48, С. 102920 - 102920
Опубликована: Июнь 18, 2022
Язык: Английский
Процитировано
201Water Research, Год журнала: 2022, Номер 229, С. 119422 - 119422
Опубликована: Ноя. 25, 2022
With the significant increase in WQI applications worldwide and lack of specific application guidelines, accuracy reliability models is a major issue. It has been reported that produce uncertainties during various stages their including: (i) water quality indicator selection, (ii) sub-index (SI) calculation, (iii) weighting (iv) aggregation sub-indices to calculate overall index. This research provides robust statistically sound methodology for assessment model uncertainties. Eight are considered. The Monte Carlo simulation (MCS) technique was applied estimate uncertainty, while Gaussian Process Regression (GPR) algorithm utilised predict at each sampling site. functions were found contribute considerable uncertainty hence affect - they contributed 12.86% 10.27% summer winter applications, respectively. Therefore, selection function needs be made with care. A low less than 1% produced by processes. Significant statistical differences between functions. weighted quadratic mean (WQM) provide plausible coastal waters reduced levels. findings this study also suggest unweighted root means squared (RMS) could potentially used quality. Findings from inform range stakeholders including decision-makers, researchers, agencies responsible monitoring, management.
Язык: Английский
Процитировано
142Journal of Environmental Management, Год журнала: 2022, Номер 321, С. 115923 - 115923
Опубликована: Авг. 19, 2022
Coastal water quality assessment is an essential task to keep "good quality" status for living organisms in coastal ecosystems. The Water index (WQI) a widely used tool assess but this technique has received much criticism due the model's reliability and inconsistence. present study recently developed improved WQI model calculating WQIs Cork Harbour. aim of research determine most reliable robust machine learning (ML) algorithm(s) anticipate at each monitoring point instead repeatedly employing SI weight values order reduce uncertainty. In study, we compared eight commonly algorithms, including Random Forest (RF), Decision Tree (DT), K-Nearest Neighbors (KNN), Extreme Gradient Boosting (XGB), Extra (ExT), Support Vector Machine (SVM), Linear Regression (LR), Gaussian Naïve Bayes (GNB). For purposes developing prediction models, dataset was divided into two groups: training (70%) testing (30%), whereas models were validated using 10-fold cross-validation method. evaluate models' performance, RMSE, MSE, MAE, R2, PREI metrics study. tree-based DT (RMSE = 0.0, MSE MAE R2 1.0 PERI 0.0) ExT ensemble XGB +0.16 -0.17) RF 2.0, 3.80, 1.10, 0.98, +3.52 -25.38) outperformed other models. results performance indicate that DT, ExT, GXB could be effective, significantly uncertainty predicting WQIs. findings are also useful reducing optimizing WQM-WQI architecture values.
Язык: Английский
Процитировано
137Journal of King Saud University - Computer and Information Sciences, Год журнала: 2021, Номер 34(8), С. 4773 - 4781
Опубликована: Июнь 14, 2021
Estimating water quality has been one of the significant challenges faced by world in recent decades. This paper presents a prediction model utilizing principal component regression technique. Firstly, index (WQI) is calculated using weighted arithmetic method. Secondly, analysis (PCA) applied to dataset, and most dominant WQI parameters have extracted. Thirdly, predict WQI, different algorithms are used PCA output. Finally, Gradient Boosting Classifier utilized classify status. The proposed system experimentally evaluated on Gulshan Lake-related dataset. results demonstrate 95% accuracy for method 100% classification method, which show credible performance compared with state-of-art models.
Язык: Английский
Процитировано
136Ecotoxicology and Environmental Safety, Год журнала: 2021, Номер 212, С. 111992 - 111992
Опубликована: Янв. 30, 2021
Groundwater is an important water resource in arid and semi-arid regions. The impact of human activities on groundwater increasing. After 60 years running, the quality its formation mechanism are imperative questions needed to be answered Jiaokou Irrigation District, Guanzhong Basin, China. In this study, District was assessed by a new integrated-weight index (IWQI), chemistry studied through integrated statistical, geostatistical hydrogeochemical approaches. patterns for average anion cation concentrations were HCO3− > SO42− Cl− NO3− CO32− NO2−, Na+ Mg2+ Ca2+ K+ NH4+, respectively. Statistics showed that major types HCO3-Na, SO4·Cl-Na, Cl·SO4-Na. A (IWQI) proposed based entropy-weighted method CRITIC excellent performance explaining evaluating quality. IWQI results show 65.33% groundwater, mainly distributed central western parts study area, unsuitable drinking. Furthermore, SO42-, HCO3-, Cl-, NO3-, had more effects weathering process affecting area carbonate dissolution, followed silicate evaporite whereas geochemical processes include dissolution precipitation calcite, as well dolomite gypsum (anhydrite). Cation exchange also plays role evolution with long residence time. Anthropogenic included long-term irrigation infiltration excessive use fertilizers. findings can improve understanding driving used reference other similar regions world.
Язык: Английский
Процитировано
112Stochastic Environmental Research and Risk Assessment, Год журнала: 2022, Номер 36(9), С. 2661 - 2680
Опубликована: Янв. 20, 2022
Язык: Английский
Процитировано
111