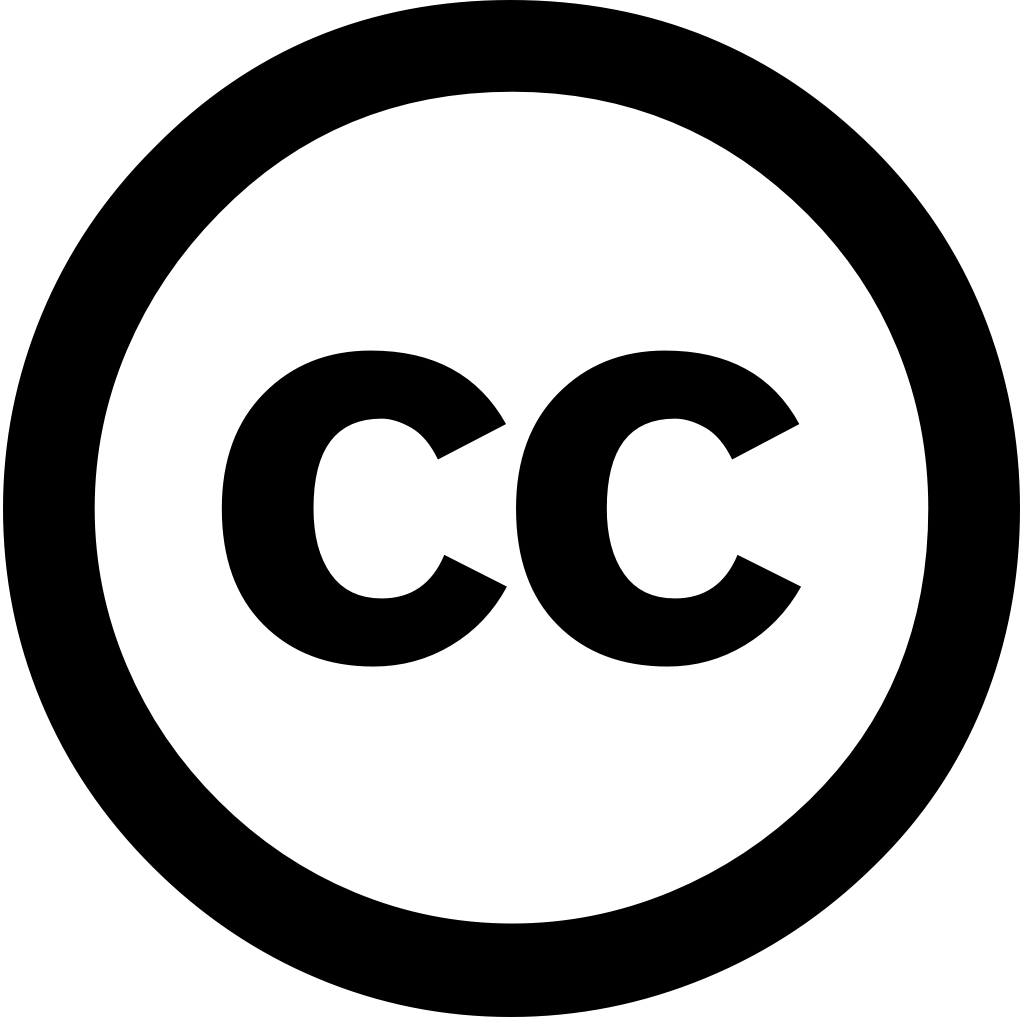
Buildings, Год журнала: 2024, Номер 14(12), С. 4024 - 4024
Опубликована: Дек. 18, 2024
The relationship between coronary heart disease (CHD) and complex urban built environments remains a subject of considerable uncertainty. development predictive models via machine learning to explore the underlying mechanisms this association, as well formulation intervention policies planning strategies, has emerged pivotal area research. A cross-sectional dataset hospital admissions for CHD over course year from in Dalian City, China, was assembled matched with multi-source environment data residential addresses. This study evaluates five models, including decision tree (DT), random forest (RF), eXtreme gradient boosting (XGBoost), multi-layer perceptron (MLP), support vector (SVM), compares them multiple linear regression models. results show that DT, RF, XGBoost exhibit superior capabilities, all R2 values exceeding 0.70. DT model performed best, an value 0.818, best performance based on metrics such MAE MSE. Additionally, using explainable AI techniques, reveals contribution different factors identifies significant influencing cold regions, ranked age, Digital Elevation Model (DEM), house price (HP), sky view factor (SVF), interaction factors. Stratified analyses by age gender variations groups: those under 60 years old, Road Density is most influential factor; 61–70 group, top 71–80 81 building height leading males, GDP females, factor. explores feasibility predicting risk regions provides comprehensive methodology workflow cardiovascular refined neighborhood-level factors, offering scientific construction sustainable healthy cities.
Язык: Английский