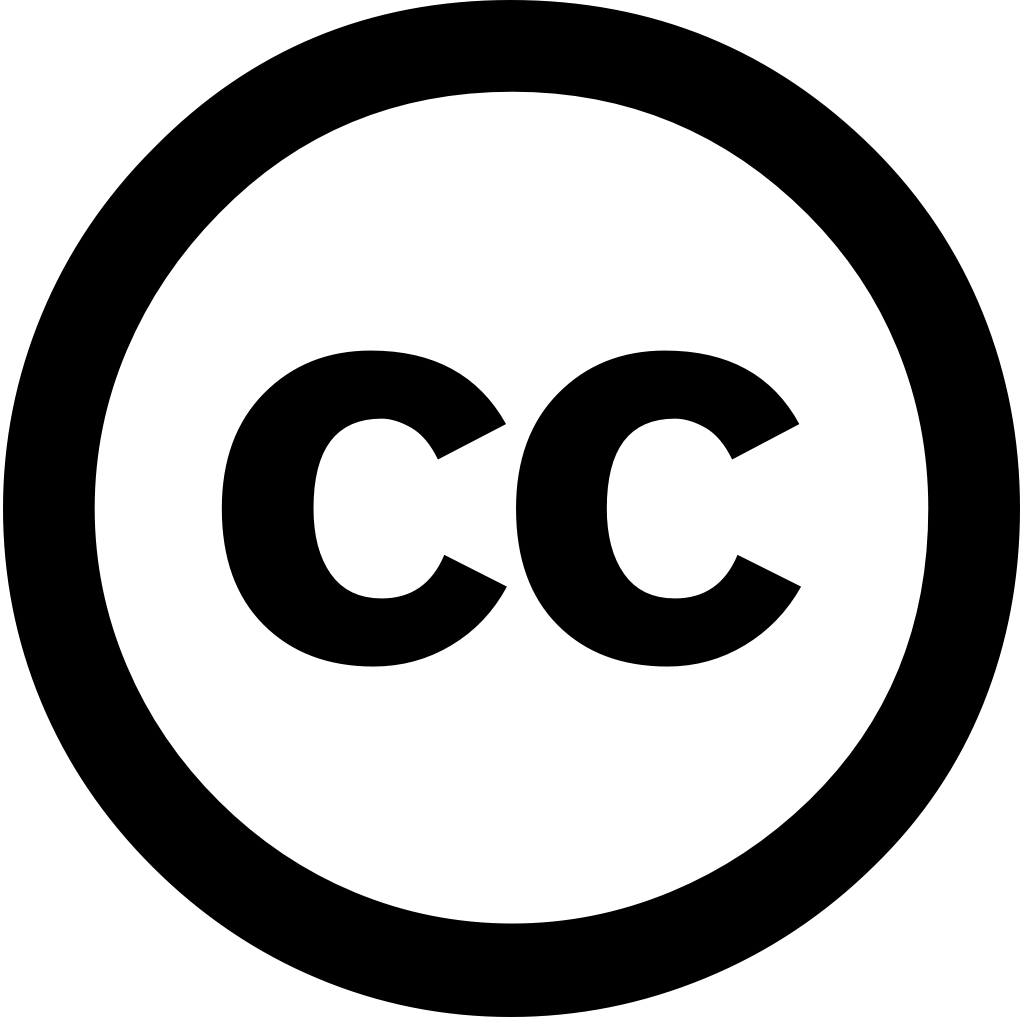
Smart Energy, Год журнала: 2024, Номер unknown, С. 100167 - 100167
Опубликована: Ноя. 1, 2024
Язык: Английский
Smart Energy, Год журнала: 2024, Номер unknown, С. 100167 - 100167
Опубликована: Ноя. 1, 2024
Язык: Английский
Journal of Building Engineering, Год журнала: 2025, Номер unknown, С. 111694 - 111694
Опубликована: Янв. 1, 2025
Язык: Английский
Процитировано
2Asian Journal of Research in Computer Science, Год журнала: 2024, Номер 17(6), С. 188 - 201
Опубликована: Май 14, 2024
This study compares Support Vector Machine (SVM), Logistic Regression, and Decision Tree algorithms for liver disease prediction using a dataset sourced from Kaggle, comprising 20,000 training records approximately 1,000 test records. The research evaluates the based on performance metrics, including accuracy, precision, recall, F1-score. SVM emerged as most effective model with an accuracy of 85%, followed by Regression 82% 79%. findings underscore significance algorithm selection in healthcare applications highlight SVM's potential early detection intervention cases, paving way improved patient outcomes management. Future work will focus refining validating results larger more diverse datasets to enhance predictive robustness further.
Язык: Английский
Процитировано
10Energy Exploration & Exploitation, Год журнала: 2024, Номер 42(6), С. 2191 - 2217
Опубликована: Авг. 2, 2024
Due to rising demand for energy-efficient buildings, advanced predictive models are needed evaluate heating and cooling load requirements. This research presents a unified strategy that blends LSTM networks GBM improve building energy estimates’ precision reliability. Data on usage, weather conditions, occupancy trends, features is collected prepared start the process. model attributes created using sequential relationships initial projections networks. Combining with takes advantage of each model's strengths: LSTM's data processing GBM's complex nonlinear connection capture. Performance measures like RMSE MAE used hybrid validity. Compared individual models, integrated LSTM-GBM method improves prediction accuracy. higher capacity allows real-time management systems, improving operations reducing use. Implementing this in Building Management Systems (BMS) shows its practicality achieving sustainable efficiency.
Язык: Английский
Процитировано
4Building and Environment, Год журнала: 2025, Номер unknown, С. 113051 - 113051
Опубликована: Апрель 1, 2025
Язык: Английский
Процитировано
0Опубликована: Апрель 1, 2024
This study delves into hospital mobility, understood as an indicator of perceived service quality, across the Italian regions Apulia and Emilia Romagna, utilizing logistic regression among machine learning techniques. The focus is on how structural, operational, clinical variables impact patient perceptions influencing their healthcare choices. Through analysis mobility trends with learning, significant differences between were uncovered, highlighting influence regional context quality. integration SHAP (SHapley Additive exPlanations) values our provided deeper insights model, elucidating specific contribution each variable to incorporation underscores study's commitment employing advanced, explainable AI techniques enhance interpretability fairness evaluations. choice elucidated quality perception, offering essential for optimizing resource distribution underscoring importance data-driven strategies foster more equitable, efficient, patient-centred systems. Contributing understanding dynamics within context, research paves way further investigations enhancing accessibility leveraging a tool improving services efficiency in diverse settings.
Язык: Английский
Процитировано
2Applied Sciences, Год журнала: 2024, Номер 14(14), С. 6016 - 6016
Опубликована: Июль 10, 2024
This study delves into hospital mobility within the Italian regions of Apulia and Emilia-Romagna, interpreting it as an indicator perceived service quality. Utilizing logistic regression alongside other machine learning techniques, we analyze impact structural, operational, clinical variables on patient perceptions quality, thus influencing their healthcare choices. The analysis trends has uncovered significant regional differences, emphasizing how context shapes To further enhance analysis, SHAP (SHapley Additive exPlanations) values have been integrated model. These quantify specific contributions each variable to quality service, significantly improving interpretability fairness evaluations. A methodological innovation this is use these scores weights in data envelopment (DEA), facilitating a comparative efficiency facilities that both weighted normative. combination SHAP-weighted DEA provides deeper understanding dynamics offers essential insights for optimizing distribution resources. approach underscores importance data-driven strategies develop more equitable, efficient, patient-centered systems. research contributes promotes investigations accessibility leveraging tool increase services across diverse settings. findings are pivotal policymakers system managers aiming reduce disparities promote responsive personalized service.
Язык: Английский
Процитировано
2Energy and Buildings, Год журнала: 2024, Номер 320, С. 114598 - 114598
Опубликована: Июль 27, 2024
Hospitals, due to their complexity and unique requirements, play a pivotal role in global energy consumption patterns. This study conducted comprehensive literature review, utilizing the PRISMA framework, of articles that employed machine learning artificial intelligence techniques for predicting hospital buildings. Of 2,157 publications identified, 35 specifically addressed this domain were thoroughly reviewed establish state-of-the-art identify research gaps. The review revealed diverse range data inputs influencing prediction, with occupancy meteorological emerging as significant predictors. However, many studies did not delve deeply into implications choices, highlighting gaps understanding time dynamics, operational status, preprocessing methods. Machine learning, especially deep models like neural networks (ANNs), showed potential but faced challenges, including interpretability computational demands. Our emphasized necessity detailed daily activity broader spectrum enhance prediction accuracy. Advanced feature engineering identified crucial improving model performance. integration real-time Intelligent Energy Management Systems (IEMS) long-term forecasting are areas future should prioritize holistic sustainability healthcare facilities. Additionally, exploration hybrid optimization strategies enhancing recognized advancing application AI field. By addressing these areas, can significantly contribute developing more efficient sustainable management practices hospitals. findings underscore immense optimizing also highlight need granular research.
Язык: Английский
Процитировано
1Energy Conversion and Management, Год журнала: 2024, Номер 320, С. 118953 - 118953
Опубликована: Сен. 3, 2024
Язык: Английский
Процитировано
0Energies, Год журнала: 2024, Номер 17(18), С. 4702 - 4702
Опубликована: Сен. 21, 2024
The present paper reviews the hot topics at 18th Conference on Sustainable Development of Energy, Water, and Environment Systems (SDEWES) held from 24 to 29 September 2023 in Dubrovnik, Croatia. selected papers, including aspects biomass energy application, saving building, low-carbon development climate change adaptation, hybrid application sustainable energy, storage system, other measures development, are published this Special Issue Energies. 12 papers Energies corresponding literature that relates above has been most recent year reviewed. In particular, diversifying applications trending towards comprehensive integration with more efficient clean capacities for global procurement, which can also offer increased resilience sustainability, reduce reliance fossil fuels, mitigate environmental impacts, enhance security through a mix renewable sources innovative technologies. Meanwhile, become increasingly important, manage intermittent stabilize grid, improve against disruptions. addition conventional solar storage, building water etc., overall costs, deferring investments additional transmission infrastructure.
Язык: Английский
Процитировано
0Smart Energy, Год журнала: 2024, Номер unknown, С. 100167 - 100167
Опубликована: Ноя. 1, 2024
Язык: Английский
Процитировано
0