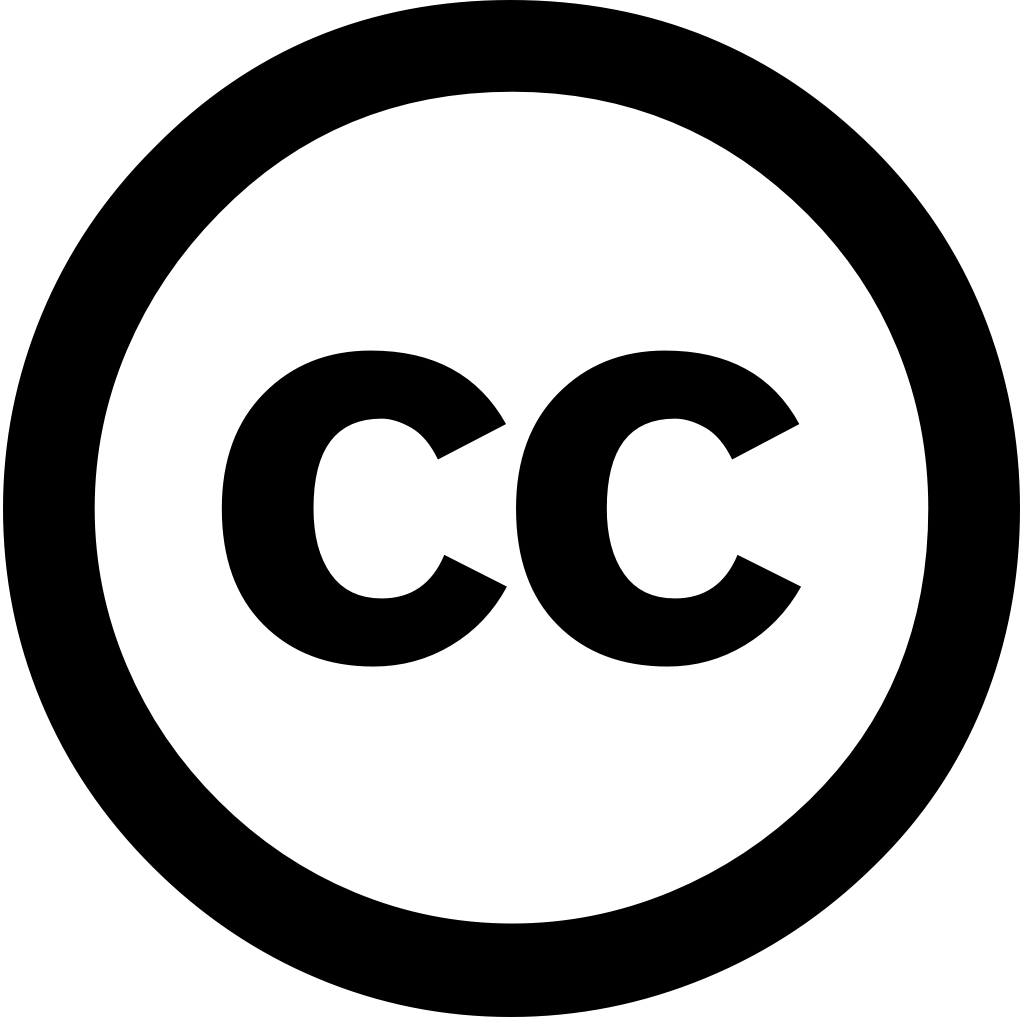
Agricultural and Forest Meteorology, Год журнала: 2025, Номер 370, С. 110600 - 110600
Опубликована: Май 4, 2025
Язык: Английский
Agricultural and Forest Meteorology, Год журнала: 2025, Номер 370, С. 110600 - 110600
Опубликована: Май 4, 2025
Язык: Английский
Field Crops Research, Год журнала: 2025, Номер 322, С. 109745 - 109745
Опубликована: Янв. 10, 2025
Язык: Английский
Процитировано
0Agriculture, Год журнала: 2025, Номер 15(5), С. 464 - 464
Опубликована: Фев. 21, 2025
In modern agriculture, timely and accurate crop yield information is crucial for optimising agricultural production management resource allocation. This study focused on improving the prediction accuracy of pear yields. Taking Alar City, Xinjiang, China as research area, a variety data including leaf area index (LAI), soil moisture (SM) remote sensing were collected, covering four key periods growth. Three advanced algorithms, Partial Least Squares Regression (PLSR), Support Vector (SVR) Random Forest (RF), used to construct regression models LAI vegetation in using Sentinel-2 satellite data. The results showed that RF algorithm provided best when inverting LAI. coefficients determination (R2) 0.73, 0.72, 0.76, 0.77 periods, respectively, root-mean-square errors (RMSE) 0.21 m2/m2, 0.24 0.18 0.16 respectively. Therefore, was selected preferred method inversion this study. Subsequently, further explored potential assimilation techniques enhancing simulation. SM incorporated into World Food Studies (WOFOST) growth model by namely, Four-Dimensional Variational Approach (4D-Var), Particle Swarm Optimisation (PSO) algorithm, Ensemble Kalman Filter (EnKF), (PF) separate joint assimilation, experimental assimilated significantly improved compared unassimilated model. particular, EnKF highest estimation with R2 0.82, 0.79 RMSE 1056 kg/ha 1385 alone assimilated, whereas 4D-Var performed jointly high 0.88, reduced 923 kg/ha. addition, it found assimilating outperformed one variable, enhanced predictive performance beyond variable alone. summary, present demonstrated great provide strong support effectively integrating through assimilation.
Язык: Английский
Процитировано
0Information Processing in Agriculture, Год журнала: 2025, Номер unknown
Опубликована: Фев. 1, 2025
Язык: Английский
Процитировано
0Plants, Год журнала: 2025, Номер 14(8), С. 1206 - 1206
Опубликована: Апрель 14, 2025
The integration of crop models and remote sensing data has become a useful method for monitoring growth status yield based on assimilation. objective this study was to use leaf area index (LAI) values plant nitrogen accumulation (PNA) generated from spectral indices calibrate the Decision Support System Agrotechnology Transfer (DSSAT) model using Monte Carlo Markov Chain (MCMC) technique. initial management parameters, including sowing date, rate, are recalibrated relationship between state variables simulated variables. This integrated technique tested independent datasets acquired three rice field tests at experimental site in Deqing, China. results showed that assimilation achieved most accurate LAI (R2 = 0.939 RMSE 0.74) PNA 0.926 7.3 kg/ha) estimations compared with method. Average differences (RE, %) inverted initialized parameters original input seeding amount were 1.33%, 4.75%, 8.16%, respectively. estimated good agreement measured 0.79 661 kg/ha). average root mean square deviation (RMSD) 745 kg/ha. Yield uncertainty quantified. found MCMC could improve estimation (LAI), (PNA), yield. Data improves prediction LAI, PNA, by solving saturation effect normalized difference vegetation (NDVI). proposed can provide precise decision-making support anticipate regional fluctuations advance.
Язык: Английский
Процитировано
0Agricultural and Forest Meteorology, Год журнала: 2025, Номер 370, С. 110600 - 110600
Опубликована: Май 4, 2025
Язык: Английский
Процитировано
0