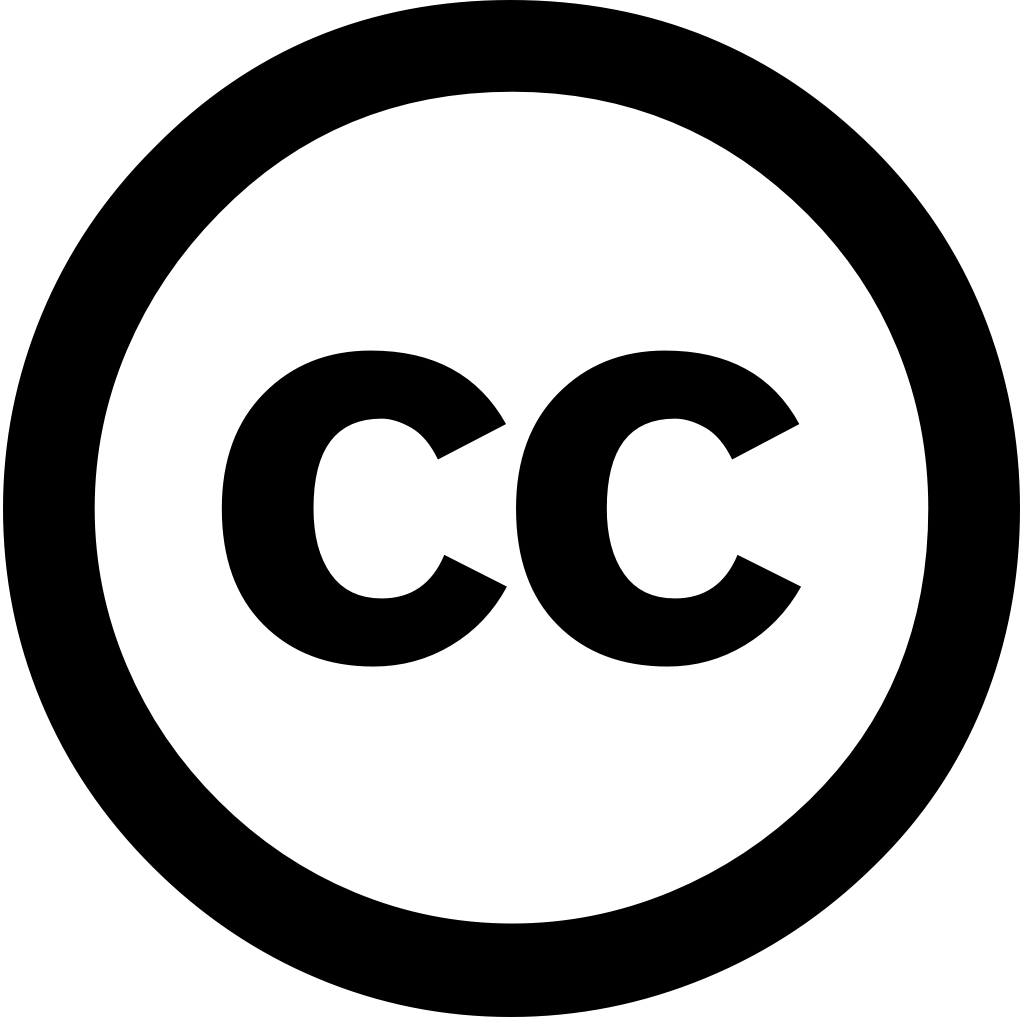
Ecological Informatics, Год журнала: 2025, Номер unknown, С. 103153 - 103153
Опубликована: Апрель 1, 2025
Язык: Английский
Ecological Informatics, Год журнала: 2025, Номер unknown, С. 103153 - 103153
Опубликована: Апрель 1, 2025
Язык: Английский
The Science of The Total Environment, Год журнала: 2025, Номер 966, С. 178715 - 178715
Опубликована: Фев. 1, 2025
Язык: Английский
Процитировано
2Earth Science Informatics, Год журнала: 2025, Номер 18(2)
Опубликована: Фев. 1, 2025
Язык: Английский
Процитировано
0Journal of Water Process Engineering, Год журнала: 2025, Номер 72, С. 107350 - 107350
Опубликована: Март 13, 2025
Язык: Английский
Процитировано
0Scientific Reports, Год журнала: 2025, Номер 15(1)
Опубликована: Апрель 10, 2025
Lake Balaton, a large shallow freshwater lake in Hungary, exhibits diverse bacterioplankton communities influenced by various environmental factors. This study aims to evaluate the bacterial diversity Balaton using long-read approach 16 S rRNA gene sequencing. Water samples were collected from wide network of 33 locations across lake's four basins and analyzed for community composition. Sequencing results revealed high taxonomic with significant zonal variations. Dominant families included Comamonadaceae, Burkholderiaceae, Methylophilaceae. Environmental parameters such as temperature, pH, CDOM found significantly correlate abundance diversity. The underscores utility portability sequencing technology assessing microbial provides insights into ecological dynamics lakes.
Язык: Английский
Процитировано
0Ecological Indicators, Год журнала: 2025, Номер 174, С. 113450 - 113450
Опубликована: Апрель 11, 2025
Язык: Английский
Процитировано
0Scientific Reports, Год журнала: 2025, Номер 15(1)
Опубликована: Апрель 14, 2025
To address the limitations of existing water quality prediction models in handling non-stationary data and capturing multi-scale features, this study proposes a hybrid model integrating Complete Ensemble Empirical Mode Decomposition with Adaptive Noise (CEEMDAN), Variational (VMD), Long Short-Term Memory Network (LSTM), Frequency-Enhanced Channel Attention (FECA). The aims to improve accuracy robustness for complex dynamics, which is critical environmental protection sustainable resource management. First, CEEMDAN Sample Entropy (SE) were used decompose raw into interpretable components filter noise. Then, VMD-enhanced LSTM architecture embedded FECA was developed adaptively prioritize frequency-specific thereby improving model's ability handle nonlinear patterns. Results show that successful predicting all six indicators: NH₃-N (ammonia nitrogen), DO (dissolved oxygen), pH, TN (total TP phosphorus), CODMn (chemical oxygen demand, permanganate method). achieved Nash-Sutcliffe Efficiency (NSE) values ranging from 0.88 0.99. Using dissolved (DO) as an example, reduced Mean Absolute Percentage Error (MAPE) by 0.12% increased coefficient determination (R2) 0.20% compared baseline methods. This work provides robust framework real-time monitoring supports decision making pollution control ecosystem
Язык: Английский
Процитировано
0Опубликована: Апрель 1, 2025
Процитировано
0Journal of Water Process Engineering, Год журнала: 2025, Номер 73, С. 107685 - 107685
Опубликована: Апрель 18, 2025
Язык: Английский
Процитировано
0Ecological Informatics, Год журнала: 2025, Номер unknown, С. 103153 - 103153
Опубликована: Апрель 1, 2025
Язык: Английский
Процитировано
0