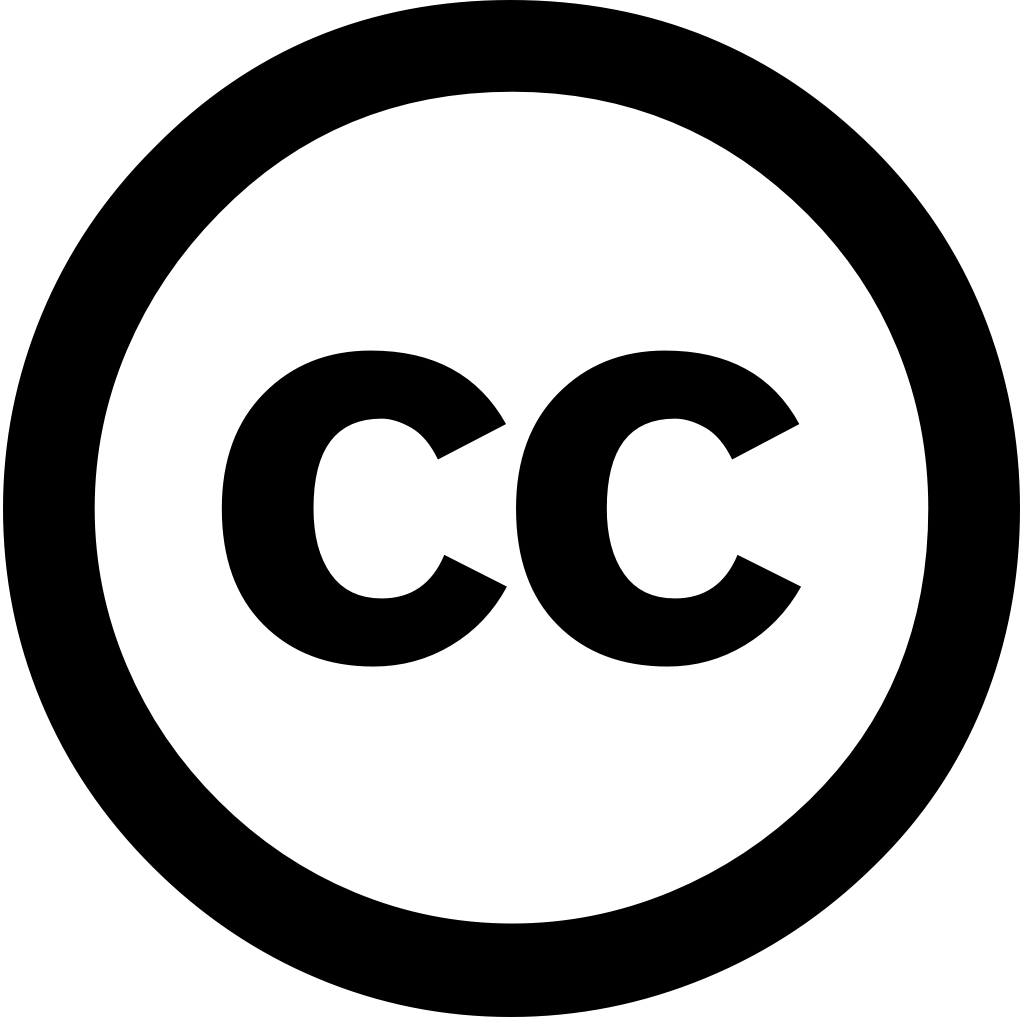
Digital Discovery, Год журнала: 2024, Номер unknown
Опубликована: Янв. 1, 2024
We propose a strategy to perform electrostatic embedding machine learning (ML)/molecular mechanics (MM) molecular dynamics simulations.
Язык: Английский
Digital Discovery, Год журнала: 2024, Номер unknown
Опубликована: Янв. 1, 2024
We propose a strategy to perform electrostatic embedding machine learning (ML)/molecular mechanics (MM) molecular dynamics simulations.
Язык: Английский
Journal of Chemical Theory and Computation, Год журнала: 2025, Номер unknown
Опубликована: Янв. 3, 2025
The development of machine-learning (ML) potentials offers significant accuracy improvements compared to molecular mechanics (MM) because the inclusion quantum-mechanical effects in interactions. However, ML simulations are several times more computationally demanding than MM simulations, so there is a trade-off between speed and accuracy. One possible compromise hybrid machine learning/molecular (ML/MM) approaches with mechanical embedding that treat intramolecular interactions ligand at level protein–ligand level. Recent studies have reported improved binding free energy results based on ML/MM using ANI-2x embedding, arguing like torsion often limiting factor for This claim evaluated 108 relative calculations four different benchmark systems. As an alternative strategy, we also tested tool fits dihedral theory. Fitting was performed AIMNet2, and, system TYK2, ωB97M-D3(BJ)/def2-TZVPPD. Overall, from Open Force Field 2.2.0, ML-fitted potentials, corresponding end-state corrected show no statistically differences mean absolute errors (between 0.8 0.9 kcal mol–1). can probably be explained by usage same parameters calculate Therefore, well-parametrized force field par simple binding. In terms computational costs, reparametrization poor torsional preferable over employing intensive complexes embedding. Also, refitting strategy leads lower variances corrections. For corrections ML/MM, indicate better convergence advanced schemes will required applications computer-guided drug discovery.
Язык: Английский
Процитировано
1Journal of Chemical Theory and Computation, Год журнала: 2025, Номер unknown
Опубликована: Фев. 27, 2025
Inspired by the QM/MM methodology, ML/MM approach introduces a new opportunity for multiscale simulation, improving balance between accuracy and computational efficiency. Benefited from rapid advancements in molecular embedding methods, density functional theory level quantum mechanical (QM) calculations within framework can be accelerated several orders of magnitude through application machine learning (ML) potential energy surfaces. As problem inherited challenges exist designing interactions mechanics (MM) regions. In this study, electrostatic MM atoms are treated using graphical neural network based on stationary perturbation theory. protocol, we process coordinates charges to yield forces, resulting high-performance architecture. The was validated aqueous solutions alanine dipeptide allyl vinyl ether (AVE). We investigated transferability parameters trained AVE single solvent various other solvents, including water, methanol, dimethyl sulfoxide, toluene, ionic liquids, water-toluene interface environments. then established solvent-free protocol data set preparation. Comparison free landscapes Claisen rearrangement different solvation environments showed catalytic effect solutions, consistent with experiments.
Язык: Английский
Процитировано
1The Journal of Physical Chemistry Letters, Год журнала: 2025, Номер unknown, С. 774 - 781
Опубликована: Янв. 13, 2025
Incorporation of environment and vibronic effects in simulations optical spectra excited state dynamics is commonly done by combining molecular with calculations, which allows to estimate the spectral density describing frequency-dependent system-bath coupling strength. The need for efficient sampling, however, usually leads adoption classical force fields despite well-known inaccuracies due mismatch method. Here, we present a multiscale strategy that overcomes this limitation EMLE based on electrostatically embedded ML potentials QM/MMPol polarizable embedding model compute states 3-methyl-indole, chromophoric moiety tryptophan mediates variety important biological functions, gas phase, water solution, human serum albumin protein. Our protocol provides highly accurate results faithfully reproduce their ab initio QM/MM counterparts, thus paving way investigations interrelation between time scales motion photophysics other biosystems.
Язык: Английский
Процитировано
0Journal of Chemical Theory and Computation, Год журнала: 2025, Номер unknown
Опубликована: Янв. 15, 2025
Integrating machine learning potentials (MLPs) with quantum mechanical/molecular mechanical (QM/MM) free energy simulations has emerged as a powerful approach for studying enzymatic catalysis. However, its practical application been hindered by the time-consuming process of generating necessary training, validation, and test data MLP models through QM/MM simulations. Furthermore, entire needs to be repeated each specific enzyme system reaction. To overcome this bottleneck, it is required that trained MLPs exhibit transferability across different environments reacting species, thereby eliminating need retraining new variant. In study, we explore potential evaluating pretrained ΔMLP model mutations within MM environment using QM/MM-based ML architecture developed Pan, X. J. Chem. Theory Comput. 2021, 17(9), 5745–5758. The study includes scenarios such single point substitutions, homologous from even transition an aqueous environment, where last two systems have substantially used in training. results show effectively captures predicts effects on electrostatic interactions, producing reliable profiles enzyme-catalyzed reactions without retraining. also identified notable limitations transferability, particularly when transitioning water-rich environments. Overall, demonstrates robustness Pan et al.'s diverse systems, well further research development more sophisticated training methods.
Язык: Английский
Процитировано
0Wiley Interdisciplinary Reviews Computational Molecular Science, Год журнала: 2025, Номер 15(1)
Опубликована: Янв. 1, 2025
ABSTRACT The study of natural enzyme catalytic processes at a molecular level can provide essential information for rational design new enzymes, to be applied in more efficient and environmentally friendly industrial processes. use computational tools, combined with experimental techniques, is providing outstanding milestones the last decades. However, apart from complexity associated nature these large flexible biomolecular machines, full catalyzed process involves different physical chemical steps. Consequently, point view, deep understanding every single step requires selection proper technique get reliable, robust useful results. In this article, we summarize techniques their process, including conformational diversity, allostery those steps, as well enzymes. Because impact artificial intelligence all aspects science during years, special attention has been methods based on foundations some selected recent applications.
Язык: Английский
Процитировано
0Journal of Chemical Theory and Computation, Год журнала: 2025, Номер unknown
Опубликована: Март 4, 2025
We introduce an innovative machine learning (ML)-based framework for multiscale molecular modeling in which the ML subsystem is treated as electrostatic entity interacting with its mechanics (MM) environment through classical electrostatics. The integration of accuracy accomplished by leveraging capabilities ANI neural networks to predict geometry-dependent atomic partial charges at minimal basis iterative stockholder (MBIS) level, going beyond static mechanical embedding. This ML/MM approach can closely approximate state-of-the-art quantum-classical (QM/MM) methods while significantly lowering computational requirements, thereby facilitating more efficient and precise simulations chemistry. method requires no additional training initial model setup integrated into Amber, one most widely used software suites modeling, ensuring accessibility broader community. validate performance across a variety challenging applications, including solvation structure, vibrational spectra, torsion free energy profiles, protein-ligand interactions, achieving excellent agreement QM/MM benchmarks. not only advances frontiers but also showcases potential achieve quantum-level exceptional efficiency complex chemical systems.
Язык: Английский
Процитировано
0Applied Physics Reviews, Год журнала: 2025, Номер 12(2)
Опубликована: Апрель 2, 2025
A force field as accurate quantum mechanics (QMs) and fast molecular (MMs), with which one can simulate a biomolecular system efficiently enough meaningfully to get quantitative insights, is among the most ardent dreams of biophysicists—a dream, nevertheless, not be fulfilled any time soon. Machine learning fields (MLFFs) represent meaningful endeavor in this direction, where differentiable neural functions are parametrized fit ab initio energies forces through automatic differentiation. We argue that, now, utility MLFF models no longer bottlenecked by accuracy but primarily their speed, well stability generalizability—many recent variants, on limited chemical spaces, have long surpassed 1 kcal/mol—the empirical threshold beyond realistic predictions possible—though still magnitudes slower than MM. Hoping kindle exploration design faster, albeit perhaps slightly less MLFFs, review, we focus our attention technical space (the speed-accuracy trade-off) between MM ML fields. After brief review building blocks (from machine learning-centric point view) either kind, discuss desired properties challenges now faced development community, survey efforts make more envision what next generation might look like.
Язык: Английский
Процитировано
0Journal of Chemical Theory and Computation, Год журнала: 2025, Номер unknown
Опубликована: Апрель 24, 2025
Accurately modeling enzyme reactions through direct machine learning/molecular mechanics simulations remains challenging in describing the electrostatic coupling between QM and MM subsystems. In this work, we proposed a reweighting ME (mechanic embedding) REANN (recursively embedded atom neural network) method that trains potential point charges of subsystem vacuo. The charge equilibration approach has been encoded into to ensure conservation total subsystem. Electrostatic is measured by charges, polarization on can be corrected thermodynamic perturbation after molecular dynamics simulations. We first constructed surfaces energy for acylation cyclooxygenase-1 (COX-1) cyclooxygenase-2 (COX-2) aspirin. These allowed us reproduce free curves B3LYP/MM-MD with chemical accuracy. Subsequently, they were successfully applied R513A COX-2, reproducing barrier simulated B3LYP/MM MD difference less than 0.5 kcal mol-1 speedup 80-fold, revealing our predict activity mutants accurately rapidly. This expected virtual screening future.
Язык: Английский
Процитировано
0Digital Discovery, Год журнала: 2024, Номер unknown
Опубликована: Янв. 1, 2024
We propose a strategy to perform electrostatic embedding machine learning (ML)/molecular mechanics (MM) molecular dynamics simulations.
Язык: Английский
Процитировано
1