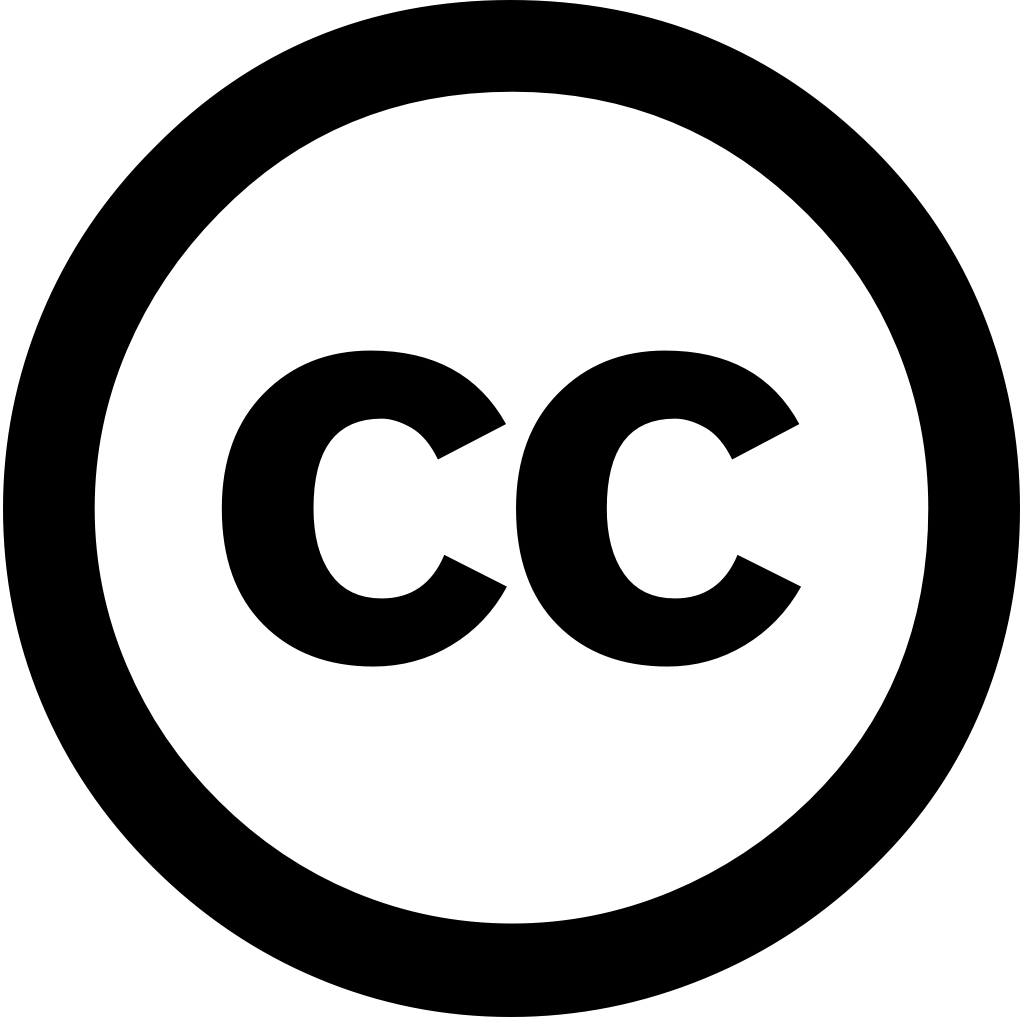
Environmental Challenges, Год журнала: 2024, Номер unknown, С. 101074 - 101074
Опубликована: Дек. 1, 2024
Язык: Английский
Environmental Challenges, Год журнала: 2024, Номер unknown, С. 101074 - 101074
Опубликована: Дек. 1, 2024
Язык: Английский
Scientific Reports, Год журнала: 2024, Номер 14(1)
Опубликована: Авг. 13, 2024
Over the past two and a half decades, rapid urbanization has led to significant land use cover (LULC) changes in Kabul province, Afghanistan. To assess impact of LULC on surface temperature (LST), province was divided into four classes applying Support Vector Machine (SVM) algorithm using Landsat satellite images from 1998 2022. The LST assessed data thermal band. Cellular Automata-Logistic Regression (CA-LR) model applied predict future patterns for 2034 2046. Results showed classes, as built-up areas increased about 9.37%, while bare soil vegetation decreased 7.20% 2.35%, respectively, analysis annual revealed that highest mean LST, followed by vegetation. simulation results indicate an expected increase 17.08% 23.10% 2046, compared 11.23% Similarly, indicated area experiencing class (≥ 32 °C) is 27.01% 43.05% 11.21% increases considerably decreases, revealing direct link between rising temperatures.
Язык: Английский
Процитировано
24Scientific Reports, Год журнала: 2025, Номер 15(1)
Опубликована: Янв. 24, 2025
The increasing trend in land surface temperature (LST) and the formation of urban heat islands (UHIs) has emerged as a persistent challenge for planners decision-makers. current research was carried out to study use cover (LULC) changes associated LST patterns planned city (Kabul) unplanned (Jalalabad), Afghanistan, using Support Vector Machine (SVM) Landsat data from 1998 2018. Future LULC were predicted 2028 2038 Cellular Automata-Markov (CA-Markov) Artificial Neural Network (ANN) models. results clearly emphasize different between Kabul Jalalabad. Between 2018, built-up areas Jalalabad increased by 16% 30%, respectively, while bare soil vegetation decreased 15% 1% 4% 30% showed highest seasonal annual LST, followed vegetation. maximum occurred during summer both cities predictions that (48% 55% 2018) will increase approximately 59% 68% 79% Jalalabad, respectively. Similarly, simulations percentage with higher (> 35°C) would (0% 5% 22% 43% 2038, Kabul's shows lower than Jalalabad's city, primarily due urbanization greater center. Urban should limit development reduce potential impacts high temperatures.
Язык: Английский
Процитировано
11GeoJournal, Год журнала: 2024, Номер 89(5)
Опубликована: Сен. 28, 2024
Язык: Английский
Процитировано
6All Earth, Год журнала: 2025, Номер 37(1), С. 1 - 14
Опубликована: Янв. 15, 2025
Changes in land use and cover can strongly affect terrestrial carbon balance, which turn the calculation of sinks that will keep future temperature within desired limits. Understanding how changes influence is challenging. Here, we simulated net balance across China with full consideration between 1981 2020 using dynamic global vegetation model. The results indicated sink ecosystem have grown steadily particularly since 2001, average values primary productivity, productivity biome were 3317 TgC • yr−1, 325 yr−1 70 yr−1. However, during period, cumulatively reduced by 1,353.00 TgC, 1,290.71 226.93 TgC. Land created a source effect abated 1981. Our findings may help guide policies to regulate order achieve neutrality future.
Язык: Английский
Процитировано
0Scientific Reports, Год журнала: 2025, Номер 15(1)
Опубликована: Янв. 25, 2025
The complex topography of the mountain cities leads to uneven distribution land resources. Currently, available studies mainly focuse on use and landscape patterns (LU LP) in plains or plateaus. Thus, it is necessary carry out an analysis drivers changes LU LP cities. As typical city China, Chongqing has changed significantly recent years. Here, we identified from 2000 2020, explored their using GeoDetector Yubei District, Chongqing. following are outcomes: (1) From construction land, wetland cropland had greatest change area, with 876.03%, -70.72% -24.53%, respectively. area transferred was larger than into it, while still largest among all types. (2) At level,construction at a low level. Grassland degree fragmentation, but showed decreasing trend. landscape's complexity resulted conversion various uses. (3) results indicated that interaction population density slope primary changing factor. study provided rational basis for development plans
Язык: Английский
Процитировано
0Environmental and Sustainability Indicators, Год журнала: 2025, Номер unknown, С. 100626 - 100626
Опубликована: Фев. 1, 2025
Язык: Английский
Процитировано
0International Journal of Information Technology, Год журнала: 2025, Номер unknown
Опубликована: Фев. 11, 2025
Язык: Английский
Процитировано
0Sensors, Год журнала: 2025, Номер 25(4), С. 1169 - 1169
Опубликована: Фев. 14, 2025
This study introduces an innovative machine learning method to model the spatial variation of land surface temperature (LST) with a focus on urban center Da Nang, Vietnam. Light Gradient Boosting Machine (LightGBM), support vector machine, random forest, and Deep Neural Network are employed establish functional relationships between LST its influencing factors. The approaches trained validated using remote sensing data from 2014, 2019, 2024. Various explanatory variables representing topographical characteristics, as well landscapes, used. Experimental results show that LightGBM outperforms other benchmark methods. In addition, Shapley Additive Explanations utilized clarify impact factors affecting LST. analysis outcomes indicate while importance these changes over time, density greenspace consistently emerge most influential attained R2 values 0.85, 0.92, 0.91 for years 2024, respectively. findings this work can be helpful deeper understanding heat stress dynamics facilitate planning.
Язык: Английский
Процитировано
0Remote Sensing, Год журнала: 2025, Номер 17(4), С. 694 - 694
Опубликована: Фев. 18, 2025
Retrieving LST from infrared spectral observations is challenging because it needs separation emissivity in surface radiation emission, which feasible only when the state of surface–atmosphere system known. Thanks to its high resolution, Infrared Atmospheric Sounding Interferometer (IASI) instrument onboard Metop polar-orbiting satellites sensor that can simultaneously retrieve LST, spectrum, and atmospheric composition. Still, cannot penetrate thick cloud layers, making blind emissions under cloudy conditions, with parameters being flagged as voids. The present paper aims discuss a downscaling–fusion methodology missing values on spatial field retrieved spatially scattered IASI yield level 3, regularly gridded data, using proxy data Spinning Enhanced Visible Imager (SEVIRI) flying Meteosat Second Generation (MSG) platform, geostationary instrument, Advanced Very High-Resolution Radiometer (AVHRR) satellites. We address this problem by machine learning techniques, i.e., Gradient Boosting, Random Forest, Gaussian Process Regression, Neural Network, Stacked Regression. applied over Po Valley region, very heterogeneous area allows addressing trained models’ robustness. Overall, methods significantly enhanced sampling, keeping errors terms Root Mean Square Error (RMSE) bias (Mean Absolute Error, MAE) low. Although we demonstrate assess results primarily also intended for applications follow-on, is, Next (IASI-NG), much more Sounder (IRS), planned fly year, 2025, Third platform (MTG).
Язык: Английский
Процитировано
0Land, Год журнала: 2025, Номер 14(3), С. 443 - 443
Опубликована: Фев. 20, 2025
The dynamics of Land Use/Land Cover changes are crucial to environmental sustainability, socio-economic development, and spatial planning. These stem from complex interactions between human activities, natural processes, policies. In recent decades, LULC transformations have been linked global challenges such as biodiversity loss, climate change, resource degradation. Key drivers include urban sprawl, agricultural expansion abandonment, deforestation, emphasizing the need for effective frameworks monitor assess their impacts. This study investigates (LULC) in Tuscany (Italy) over period 2007 2019. To achieve this, statistical analyses were conducted quantify variations across different classes administrative territories represented by provincial local authorities. Specifically, data spanning five temporal intervals (2007, 2010, 2013, 2016, 2019) enabled a comprehensive comparative analysis persistence patterns. Changes assessed using approach based on Odds Ratios (OR). Additionally, Generalized Linear Models (GLMs) at level employed facilitate one-to-many comparisons evaluate significance observed changes. revealed that certain exhibit greater susceptibility compared others. categorized under ’Artificial Surfaces’ (LC_100) were, average, 6.7 times more likely undergo than those classified ’Agricultural Areas’ (LC_200) 11 ’Forest Semi-natural (LC_300). Over time, areas artificial exhibited progressively decreasing probability change. Notably, during first update (2007–2010), these 3.5 susceptible change most (2016–2019). An additional significant finding emerged comparison regions governed authorities (Provinces). findings underscore potential indicators morphological parameters analyze trends. proposed provides robust framework interpreting territorial resilience informing planning strategies effectively.
Язык: Английский
Процитировано
0