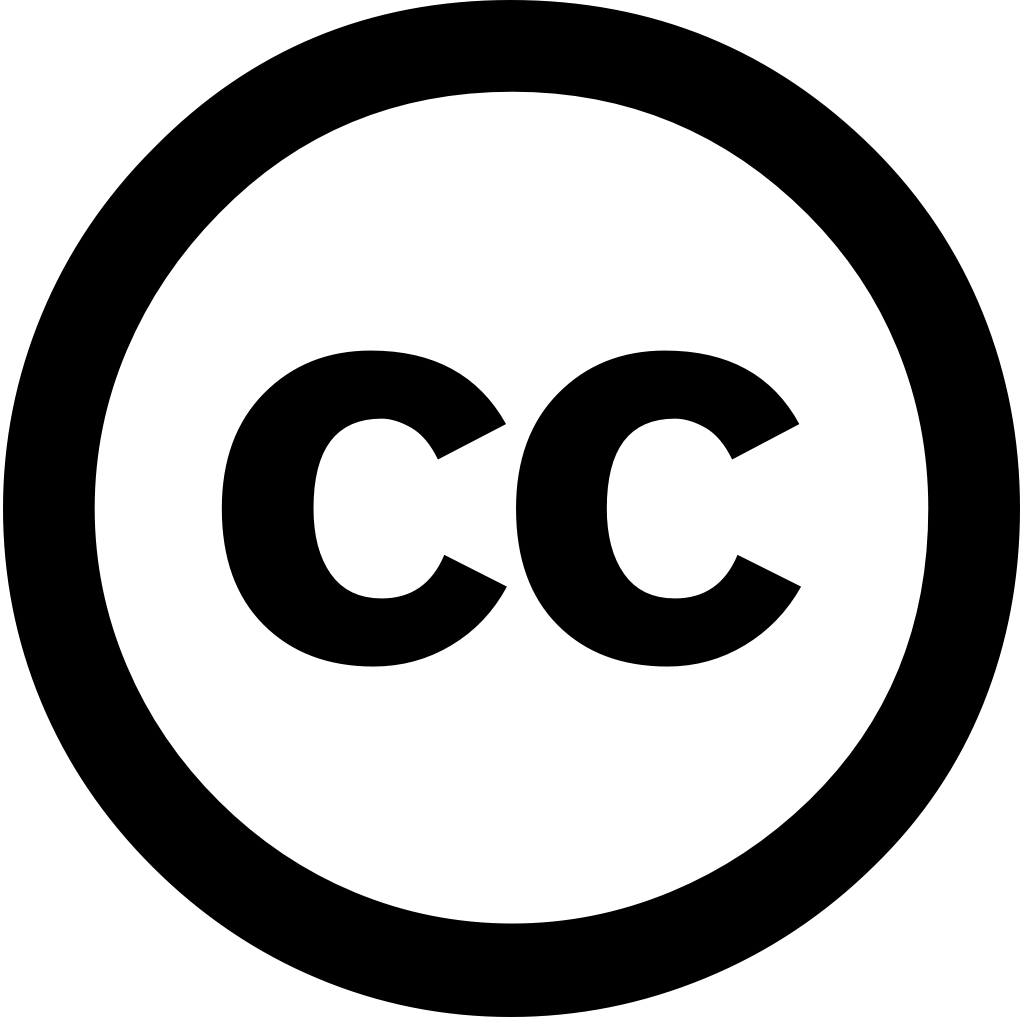
Aging Cell, Год журнала: 2025, Номер unknown
Опубликована: Янв. 5, 2025
Understanding the complex biological process of aging is great value, especially as it can help develop therapeutics to prolong healthy life. Predicting age from gene expression data has shown be an effective means quantify a subject, and identify molecular cellular biomarkers aging. A typical approach for estimating age, adopted by almost all existing clocks, train machine learning models only on subjects, but infer both unhealthy subjects. However, inherent bias in this results inaccurate study. Moreover, transcriptome-based clocks were built around inefficient procedure selection followed conventional such elastic nets, linear discriminant analysis etc. To address these limitations, we proposed DeepQA, unified clock based mixture experts. Unlike methods, DeepQA equipped with specially designed Hinge-Mean-Absolute-Error (Hinge-MAE) loss so that subjects multiple cohorts reduce inferring Our experiments showed significantly outperformed methods estimation In addition, our method avoids exhaustive search genes, provides novel genes activated prediction, alternative differential analysis.
Язык: Английский