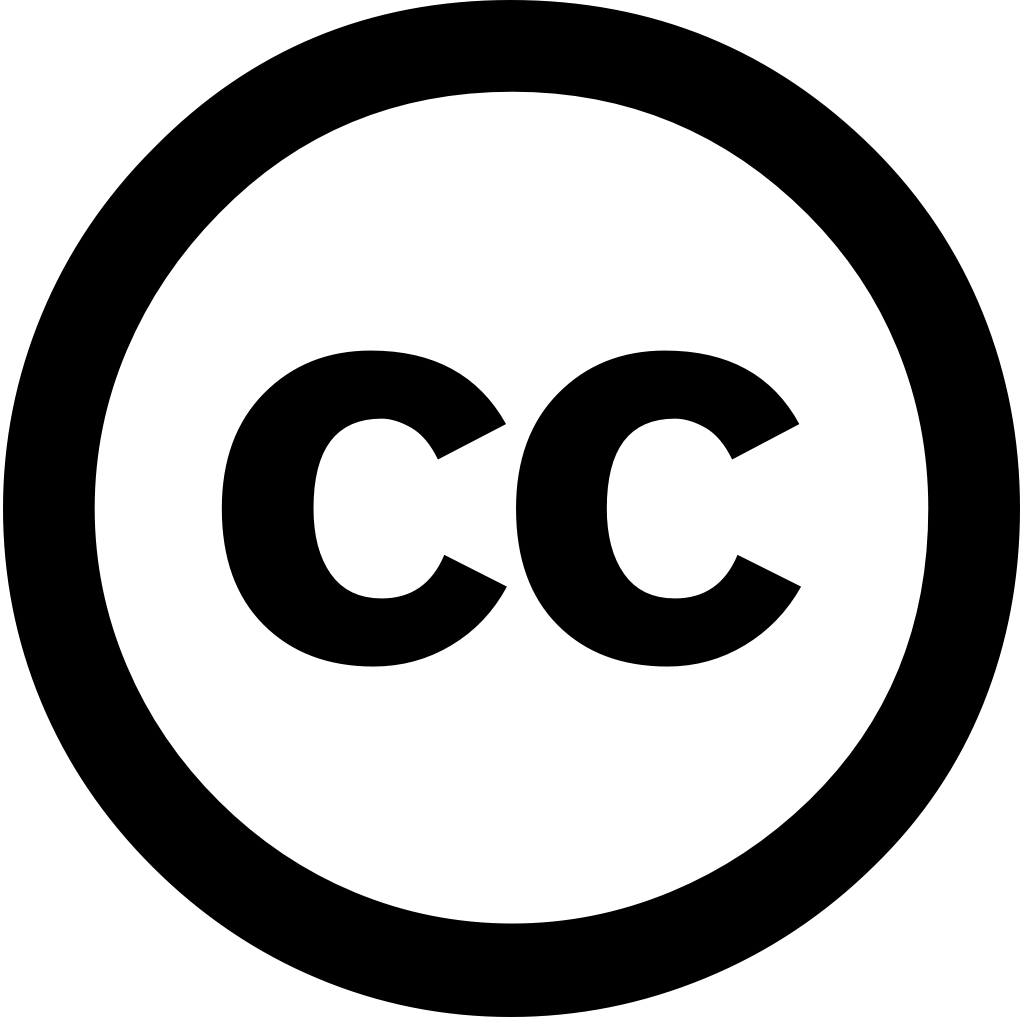
Revista Portuguesa de Psiquiatria e Saúde Mental, Год журнала: 2024, Номер 10(2), С. 35 - 37
Опубликована: Дек. 29, 2024
Revista Portuguesa de Psiquiatria e Saúde Mental, Год журнала: 2024, Номер 10(2), С. 35 - 37
Опубликована: Дек. 29, 2024
Frontiers in Digital Health, Год журнала: 2025, Номер 7
Опубликована: Фев. 27, 2025
This Research Topic gathers different contributions addressing the practical advancement of concept digital twins in medicine, moving it form a vague theoretical towards foundation to tools used everyday healthcare. The twin (sometimes known as virtual twin) is that mainstream manufacturing, where representation created an intended or actual real-world physical product, system, process (the twin). serves effectively indistinguishable counterpart and for purposes such simulation, monitoring maintenance (Singh et al., 2021). has existed medicine decades, but unlike industry, not found its way dayto-day application patient care (Venkatesh 2022;Derraz 2024). Despite this there renewed research interest theme.The goal was address if we are at dawn medical practice explore what needed realize this. articles help define aspects near translation those need substantially more preclinical development before possible. Digital Twins patients, which have been defined various ways "a viewable replica patient, organ, biological system contains multidimensional, patient-specific information informs decisions" (Drummond Gonsard, 2024), involve only new forms about also simulation methods often AI-based predictive analytical methods. There much hype excitation AI, AI will delivery promise firmly linked status datain other words twin. These raise regulatory ethical questions, with differing approaches countries -a bring some clarity these challenges alongside proposed strategies developments, serve description state art path impact medicine. provisional file, final typesetThe first article (Laubenbacher 2024) clinicians data-driven decision support clear, already use. 60 authors describe similarity-based approach matches patients similar historical 61 cases predict treatment outcomes. Requirements were from scientific technical literature 62 four-layer implemented. suggests multi-line 63 integration external evidence transparency data processing logic. sets 64 initial clinical evaluation illustrates through detailed 65 exemplary use case multiple myeloma. 66The third (Zhang original describes 67 framework type 2 diabetes integrates machine learning multiomic data, 68 both knowledge graphs mechanistic models. researchers developed 69 models forecast disease progression using substantial dataset comprising 70 measurements profiles. Knowledge employed interpret provide 71 context relationships. Promise demonstrated modeling reaffirming 72 targetable mechanisms features. potential DT 73 precision 74The mini review role 75 personalized therapeutics pharmaceutical manufacturing (Fischer set out 76 how pave way, systems improved 77 (as described previous three articles) facilitate 78 their management, analysis, interpretation 79 data. identify gaps be filled can part routine
Язык: Английский
Процитировано
0Neuroscience, Год журнала: 2025, Номер unknown
Опубликована: Март 1, 2025
Язык: Английский
Процитировано
0AI in Precision Oncology, Год журнала: 2025, Номер unknown
Опубликована: Март 18, 2025
Язык: Английский
Процитировано
0Bioengineering, Год журнала: 2025, Номер 12(5), С. 440 - 440
Опубликована: Апрель 23, 2025
Integrating artificial intelligence (AI), particularly large language models (LLMs), into the healthcare industry is revolutionizing field of medicine. LLMs possess capability to analyze scientific literature and genomic data by comprehending producing human-like text. This enhances accuracy, precision, efficiency extensive analyses through contextualization. have made significant advancements in their ability understand complex genetic terminology accurately predict medical outcomes. These capabilities allow for a more thorough understanding influences on health issues creation effective therapies. review emphasizes LLMs’ impact healthcare, evaluates triumphs limitations processing, makes recommendations addressing these order enhance system. It explores latest analysis, focusing enhancing disease diagnosis treatment accuracy taking account an individual’s composition. also anticipates future which AI-driven analysis commonplace clinical practice, suggesting potential research areas. To effectively leverage personalized medicine, it vital actively support innovation across multiple sectors, ensuring that AI developments directly contribute solutions tailored individual patients.
Язык: Английский
Процитировано
0ESMO Real World Data and Digital Oncology, Год журнала: 2024, Номер 6, С. 100079 - 100079
Опубликована: Окт. 7, 2024
Язык: Английский
Процитировано
3Future Internet, Год журнала: 2024, Номер 16(12), С. 462 - 462
Опубликована: Дек. 9, 2024
The rapid development of specific-purpose Large Language Models (LLMs), such as Med-PaLM, MEDITRON-70B, and Med-Gemini, has significantly impacted healthcare, offering unprecedented capabilities in clinical decision support, diagnostics, personalized health monitoring. This paper reviews the advancements medicine-specific LLMs, integration Retrieval-Augmented Generation (RAG) prompt engineering, their applications improving diagnostic accuracy educational utility. Despite potential, these technologies present challenges, including bias, hallucinations, need for robust safety protocols. also discusses regulatory ethical considerations necessary integrating models into mainstream healthcare. By examining current studies developments, this aims to provide a comprehensive overview state LLMs medicine highlight future directions research application. study concludes that while hold immense safe effective practice requires rigorous testing, ongoing evaluation, continuous collaboration among stakeholders.
Язык: Английский
Процитировано
3Nature Reviews Clinical Oncology, Год журнала: 2024, Номер 21(11), С. 769 - 770
Опубликована: Сен. 5, 2024
Язык: Английский
Процитировано
2iGIE, Год журнала: 2024, Номер 3(4), С. 447 - 452.e5
Опубликована: Авг. 23, 2024
Язык: Английский
Процитировано
1Proceedings of the 28th ACM SIGKDD Conference on Knowledge Discovery and Data Mining, Год журнала: 2024, Номер unknown, С. 5138 - 5149
Опубликована: Авг. 24, 2024
Cancer remains a global challenge due to its growing clinical and economic burden. Its uniquely personal manifestation, which makes treatment difficult, has fuelled the quest for personalized strategies. Thus, genomic profiling is increasingly becoming part of diagnostic panels. Effective use such panels requires accurate drug response prediction (DRP) models, are challenging build limited labelled patient data. Previous methods address this problem have used various forms transfer learning. However, they do not explicitly model variable length sequential structure list mutations in Further, utilize auxiliary information (like survival) training. We these limitations through novel transformer-based method, surpasses performance state-of-the-art DRP models on benchmark Code our method available at https://github.com/CDAL-SOC/PREDICT-AI.
Язык: Английский
Процитировано
1JCO Precision Oncology, Год журнала: 2024, Номер 8
Опубликована: Окт. 1, 2024
PURPOSE Rapidly expanding medical literature challenges oncologists seeking targeted cancer therapies. General-purpose large language models (LLMs) lack domain-specific knowledge, limiting their clinical utility. This study introduces the LLM system Medical Evidence Retrieval and Data Integration for Tailored Healthcare (MEREDITH), designed to support treatment recommendations in precision oncology. Built on Google's Gemini Pro LLM, MEREDITH uses retrieval-augmented generation chain of thought . METHODS We evaluated 10 publicly available fictional oncology cases with iterative feedback from a molecular tumor board (MTB) at major German center. Initially limited PubMed -indexed (draft system), was enhanced incorporate studies drug response within specific type, trial databases, approval status, oncologic guidelines. The MTB provided benchmark manually curated assessed relevance LLM-generated options (qualitative assessment). measured semantic cosine similarity between suggestions clinician responses (quantitative RESULTS identified broader range (median 4) compared experts 2). These included therapies basis preclinical data combination treatments, possibilities consideration by MTB. approach achieved incorporating set that contextualized targetability. Mirroring use evaluate improved LLM's ability generate relevant suggestions. is supported high concordance expert (94.7% system) significant increase draft (from 0.71 0.76, P = .01). CONCLUSION Expert augment performance. Future research should investigate responsible integration into real-world workflows.
Язык: Английский
Процитировано
1