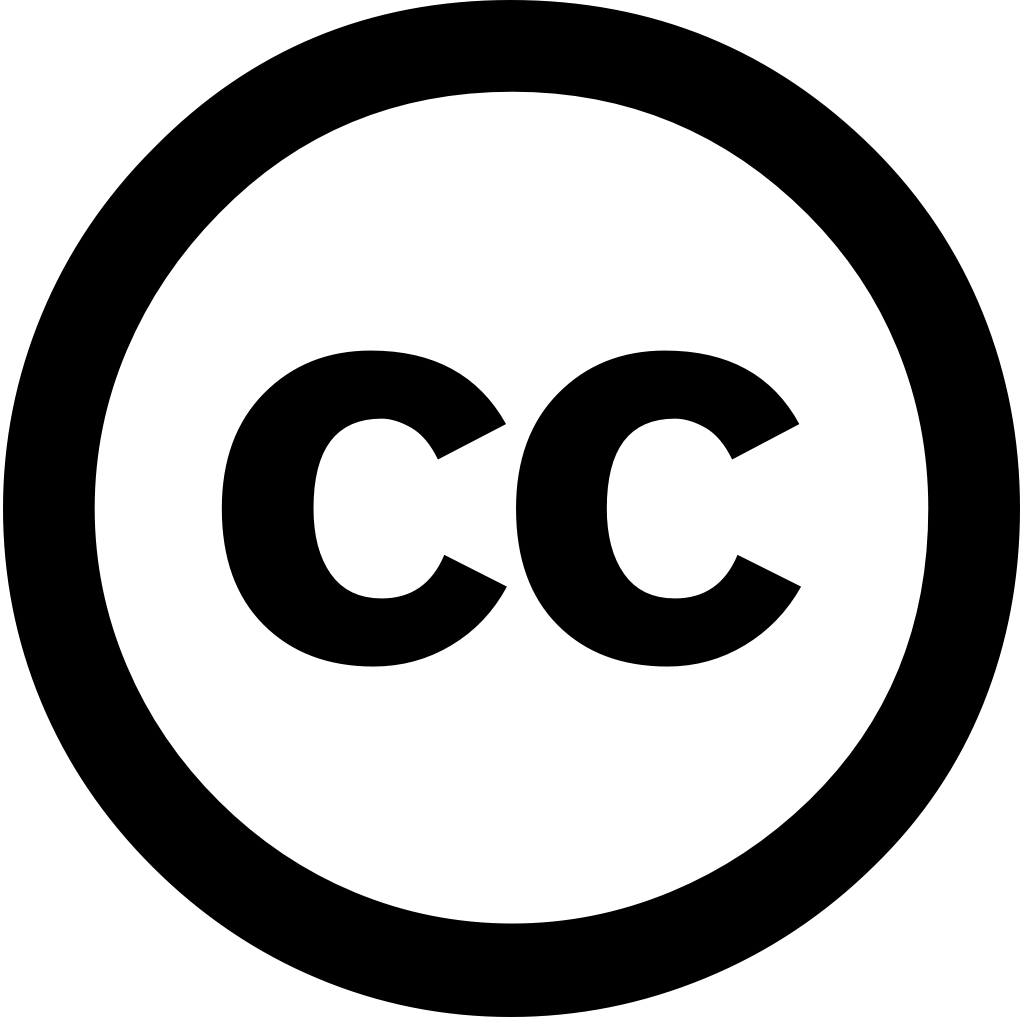
Frontiers in Medicine, Год журнала: 2024, Номер 11
Опубликована: Дек. 19, 2024
Recently the role of AI in healthcare has been deeply studied and discussed scientific literature. Promising applications artificial intelligence machine learning (AI/ML) are revolutionizing both clinical administrative domains, with significant advancements demonstrated drug discovery, precise analysis interpretation radiological images, early accurate sepsis detection, ePicient hospital resource management, automated documentation encounters decision support system (DSS). These use cases underscore immense potential AI/ML to enhance ePiciency, accuracy, outcomes across spectrum (1). However a very recent article raises essential considerations about adoption regulation settings (2). Therefore integration Artificial Intelligence (AI) presents numerous opportunities challenges. Conversely, there is gap between clinician education regarding regulatory measures necessary for ethical deployment. To address this ePectively, structured, organized approach must be followed, encompassing clearly defined steps clinicians establishment rigorous frameworks. This paper argues that bridging requires dual approach: enhancing clinicians' understanding technologies treating systems as rigorously pharmaceuticals through strict processes. By doing so, we can foster ePective into practice, ensuring patient safety better outcomes. Here, outline four key should guide planning healthcare.Looking at current practice according increase diPusion arguable knowledge physician new technology. In fact despite increasing prevalence healthcare, many remain inadequately educated what entails, its limitations implications too Given play central care, comprehensive program priority. Education initiatives focus not only on how operate but also their framework move pharmaceuticals. lack represents barrier responsible practice. ePectively bridge gap, cover technical aspects part medical degree course well. A deeper processes involved validating tools empower participate meaningfully discussions ePicacy these systems. building foundational AI, will prepared evaluate within an context advocate appropriate (3). could compared currently used improve or activity against antimicrobial resistance (AMR) stewardship (4)Legal extended beyond decision-makers regulators who interact directly For responsibly, they understand legal implications, such data privacy, accountability, risks biases (5-7). The European Union Act lays out AI; however, information often communicated those front lines Educating crucial align practical guidelines (5). As navigate environment, parameters help them mitigate ensure prioritizes respects privacy rights.There growing consensus regulated manner similar Just undergo series trials safety, ePicacy, considerations, follow validation before widespread implementation.• Phases Testing: adopt phases development-preclinical (testing controlled environments), Phase I (safety small settings), II (ePicacy trials), III (large-scale testing). structure would tested ePectiveness diverse, real-world environments.• Classification: Ai classified drugs like ATC. instance type (drug analysis, (DSS)) give code related activity.• Risk Assessments: Like drugs, thorough risk assessments, which include evaluating biases, unintended outcomes, implications.• Regulatory Oversight: dedicated agency-potentially Medicines Agency (EMA)-should combine expertise from engineering domains. mixed balanced evaluation performance tools.Finally, pharmaceuticals, come documentation, including "Summary Product Characteristics" "Package Leaflet" outlines intended use, limitations, instructions safe implementation. standardize information, enabling providers make informed decisions based clear guidance (8).However two frameworks Pharmaceuticals have some substantial diPerences (table). fact, while innovative time required approved tor usually 9.1 years (9) shorter development cycles traditional due iterative model improvements less dependency long-term biological testing. rapid pace expedited still Unlike benefit deployment, allowing updates enhancements after initial deployment user feedback, means ongoing critical. oPer advantages scalability adaptability. They rapidly scale diverse environments adjust datasets become available, setting apart more static pharmacological solutions. managed Drug Testing, Assessment Oversight being specific (10) (Figure 1).Fixed chemical entity, formula remains constant throughout.Iterative, data-driven models evolve retraining.Static population fixed protocols trials.Dynamic testing, adapting scenarios populations.Single approval process use.Continuous validation, monitoring, updates.Linear, phase-based (preclinical post-marketing).Cyclical, requiring periodic reassessments real-time monitoring.Direct physiological biochemical impact patients.Indirect influence decision-making, workflow optimization, recommendations.Specific adverse effects, localized drug.Systematic bias amplification, incorrect predictions, disruption.Fixed lifecycle, few post-market changes.Continuous retraining, updates, adaptation contexts.Efficacy measured standard endpoints trials.Performance by precision, recall, outcome improvements.Post-marketing surveillance effects.Continuous monitoring feedback loops optimization.Limited (patient compliance key).High, human-in-the-loop design during development, monitoring.Figure shows diPerence Drugs several highlight need find quick secure deployment.While commercialization inevitable, standards compromised economic gain. Proper governance, stringent oversight medical-engineering body, adhere healthcare's core principles. motivations drive innovation always secondary quality obligations. balance trust among patients (11).Artificial offers transform improving workflows driving innovation. However, successful overcoming challenges, gaps education, absence tailored regulating same rigor incorporating phases, detailed AI's fast cycles.A critical first step educating AI. Many professionals work, issues raise.Comprehensive training programs needed build knowledge. Such teaching assess effectiveness, implications. confidently benefits fully realized safeguarding trust.Equally important match unique characteristics.Unlike linear path evolves continuously. Regulations therefore robust oversight, safe, effective, free bias. collaboration professionals, technology developers, create ethical.Economic pressures overshadow responsibilities integration.While drives innovation, care first.By fostering partnerships industry prioritize principles, practice.To following integration:1. Establish systems, drawing inspiration pharmaceutical tailoring needs.2. Develop accessible clinicians, focusing confidence competence using tools.3. Support pilot projects case studies demonstrate safely effectively different settings.By taking structured adaptive approach, integration. supporting benefiting maintaining highest standards. Ultimately, strategy enable fulfill promise transforming way equitable. (12,13).
Язык: Английский