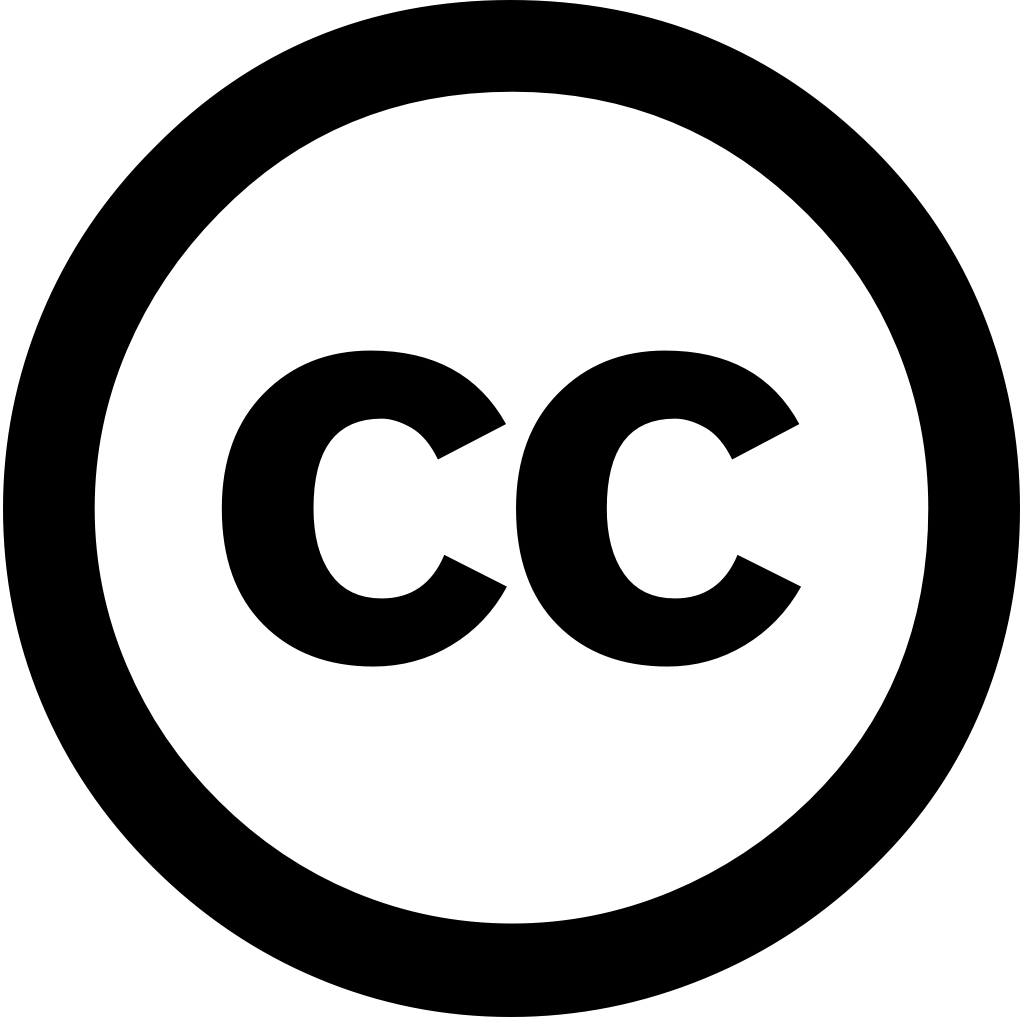
Materials Today Electronics, Год журнала: 2025, Номер unknown, С. 100138 - 100138
Опубликована: Янв. 1, 2025
Язык: Английский
Materials Today Electronics, Год журнала: 2025, Номер unknown, С. 100138 - 100138
Опубликована: Янв. 1, 2025
Язык: Английский
Advanced Energy Materials, Год журнала: 2025, Номер unknown
Опубликована: Янв. 12, 2025
Abstract Organic photovoltaics (OPV) now can exceed 20% power conversion efficiency in single junction solar cells. To close the remaining gap to competing technologies, both fill factor and open‐circuit voltage must be optimized. The Langevin reduction is a well‐known concept that measures degree which charge extraction favored over recombination. It therefore ideally suited as an optimization target high‐throughput workflows; however, its evaluation so far requires expert interaction. Here, integrated workflow presented, able obtain within few seconds with high accuracy without human intervention thus for autonomous experiments. This achieved by combining evidence from UV–vis spectra, current–voltage curves, novel implementation of microsecond transient absorption kinetics allowing, first time, intrinsic determination cross‐sections, crucial reporting stationary densities. method demonstrated varying donor:acceptor ratio performance OPV blend PM6:Y12. reproducibility allows find strictly exponential relationship between PM6 exciton energy factor.
Язык: Английский
Процитировано
1Energy & Environmental Science, Год журнала: 2022, Номер 15(7), С. 2958 - 2973
Опубликована: Янв. 1, 2022
Non-fullerene acceptors (NFAs) are excellent light harvesters, yet the origin of their high optical extinction is not well understood. In this work, we investigate absorption strength NFAs by building a database time-dependent density functional theory (TDDFT) calculations ∼500 π-conjugated molecules. The first validated comparison with experimental measurements in solution and solid state using common fullerene non-fullerene acceptors. We find that molar coefficient (εd,max) shows reasonable agreement between calculation vacuum experiment for molecules solution, highlighting effectiveness TDDFT predicting properties organic then perform statistical analysis based on molecular descriptors to identify which features important defining strength. This allows us structural correlated could be used guide design: highly absorbing should possess planar, linear, fully conjugated backbone polarisable heteroatoms. exploit random decision forest algorithm draw predictions εd,max computational framework extended tight-binding Hamiltonians, accuracy lower cost than TDDFT. work provides general understanding relationship structure molecules, including NFAs, while introducing predictive machine-learning models low cost.
Язык: Английский
Процитировано
35The Journal of Physical Chemistry A, Год журнала: 2023, Номер 127(37), С. 7823 - 7835
Опубликована: Сен. 12, 2023
Feed-forward neural networks (NNs) are a staple machine learning method widely used in many areas of science and technology, including physical chemistry, computational materials informatics. While even single-hidden-layer NN is universal approximator, its expressive power limited by the use simple neuron activation functions (such as sigmoid functions) that typically same for all neurons. More flexible would allow fewer neurons layers thereby save cost improve power. We show additive Gaussian process regression (GPR) can be to construct optimal individual each neuron. An approach also introduced avoids nonlinear fitting network parameters defining them with rules. The resulting combines advantage robustness linear higher an NN. demonstrate potential energy surfaces water molecule formaldehyde. Without requiring any optimization, additive-GPR-based outperforms conventional high-accuracy regime, where suffers more from overfitting.
Язык: Английский
Процитировано
20Modelling and Simulation in Materials Science and Engineering, Год журнала: 2024, Номер 32(6), С. 063301 - 063301
Опубликована: Май 17, 2024
Abstract Science is and always has been based on data, but the terms ‘data-centric’ ‘4th paradigm’ of materials research indicate a radical change in how information retrieved, handled performed. It signifies transformative shift towards managing vast data collections, digital repositories, innovative analytics methods. The integration artificial intelligence its subset machine learning, become pivotal addressing all these challenges. This Roadmap Data-Centric Materials explores fundamental concepts methodologies, illustrating diverse applications electronic-structure theory, soft matter microstructure research, experimental techniques like photoemission, atom probe tomography, electron microscopy. While roadmap delves into specific areas within broad interdisciplinary field science, provided examples elucidate key applicable to wider range topics. discussed instances offer insights multifaceted challenges encountered contemporary research.
Язык: Английский
Процитировано
8e-Prime - Advances in Electrical Engineering Electronics and Energy, Год журнала: 2024, Номер 7, С. 100458 - 100458
Опубликована: Фев. 6, 2024
Exploration of smart sustainable renewable energy material with the aid artificial intelligence is gaining momentum as next-generation research. The advantage high throughput screening not only increasing efficiency discovery but also can reduce conventional process. In this review, machine learning method investigation for application in conversion, storage, and energy-efficient materials has been discussed. Various ML tools closed-loop techniques are discussed keeping emphasis on both device optimization. Further, challenges future prospects automation exploration elaborated.
Язык: Английский
Процитировано
6Journal of Materials Informatics, Год журнала: 2022, Номер 2(4), С. 19 - 19
Опубликована: Янв. 1, 2022
High-entropy alloy (HEA) catalysts have recently attracted worldwide research interest due to their promising catalytic performance. Most current studies focus on designing HEA through trial-and-error methods. This produces scattered data and is not conducive obtaining a fundamental understanding of the structure-property-performance relationships for catalysts, thereby hindering rational design. High-throughput (HT) techniques machine learning (ML) methods show significant potential in generating, processing analyzing databases with vast amount data, providing new strategy further development catalysts. In this review, we summarize recent literature HT synthesis, characterization performance testing. We also review ML models that are used process analyze existing accelerate discovery Finally, challenges perspectives presented promote development.
Язык: Английский
Процитировано
24Advanced Intelligent Systems, Год журнала: 2022, Номер 4(6)
Опубликована: Март 19, 2022
The improvements of organic photovoltaics (OPVs) are mainly implemented by the design novel materials and optimizations experimental conditions through extensive trial‐and‐error experiments based on chemical intuition, which may be tedious inefficient for exploring a larger space. In recent five years, data‐driven methods using machine learning (ML) algorithms knowledge known materials/experimental parameters introduced to OPV studies help build quantitative structure‐property relationship model accelerate molecular parameter optimization. Here, these promising progresses datasets summarized. This review introduces general workflow (e.g., dataset collection, feature engineering, ML generation, evaluation) ML‐OPV projects discusses applications this framework predicting performance in OPVs. Finally, an outlook future work directions exciting quickly developing field is presented.
Язык: Английский
Процитировано
23Advanced Energy and Sustainability Research, Год журнала: 2023, Номер 4(10)
Опубликована: Авг. 23, 2023
Machine learning (ML) and artificial intelligence (AI) methods are emerging as promising technologies for enhancing the performance of low‐cost photovoltaic (PV) cells in miniaturized electronic devices. Indeed, ML is set to significantly contribute development more efficient cost‐effective solar cells. This systematic review offers an extensive analysis recent techniques designing novel cell materials structures, highlighting their potential transform research landscape. The encompasses a variety approaches, such Gaussian process regression (GPR), Bayesian optimization (BO), deep neural networks (DNNs), which have proven effective boosting efficiency, stability, affordability findings this indicate that GPR combined with BO most method developing These can speed up discovery new PV structures while efficiency stability concludes insights on challenges, prospects, future directions development.
Язык: Английский
Процитировано
15Journal of Materials Chemistry A, Год журнала: 2023, Номер 11(41), С. 22248 - 22258
Опубликована: Янв. 1, 2023
The machine learning approach was employed to explore the relationship between molecular structure and photovoltaic properties using frontier orbital RDKit descriptors, which enabled us screen identify potential donor acceptor combinations for efficient organic solar cells.
Язык: Английский
Процитировано
15Energy & Environmental Science, Год журнала: 2023, Номер 16(11), С. 5454 - 5463
Опубликована: Янв. 1, 2023
Commercialization of printed photovoltaics requires knowledge the optimal composition and microstructure single layers, ability to control these properties over large areas under industrial conditions.
Язык: Английский
Процитировано
15