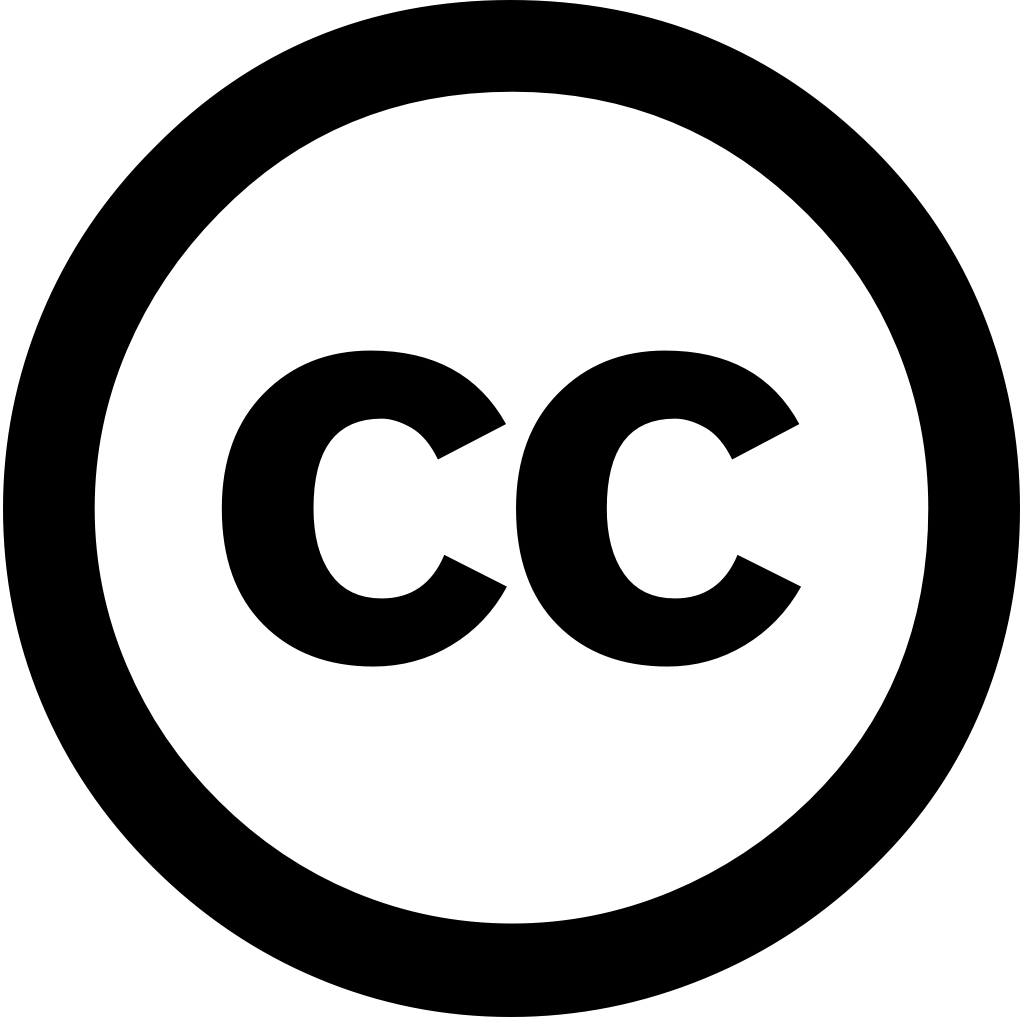
ACS Central Science, Год журнала: 2024, Номер 10(12), С. 2173 - 2174
Опубликована: Дек. 25, 2024
Язык: Английский
ACS Central Science, Год журнала: 2024, Номер 10(12), С. 2173 - 2174
Опубликована: Дек. 25, 2024
Язык: Английский
Digital Discovery, Год журнала: 2024, Номер 3(7), С. 1257 - 1272
Опубликована: Янв. 1, 2024
This perspective paper explores the potential of Large Language Models (LLMs) in materials science, highlighting their abilities to handle ambiguous tasks, automate processes, and extract knowledge at scale across various disciplines.
Язык: Английский
Процитировано
23Nature Reviews Materials, Год журнала: 2025, Номер unknown
Опубликована: Янв. 31, 2025
Язык: Английский
Процитировано
3Chemical Science, Год журнала: 2024, Номер unknown
Опубликована: Дек. 9, 2024
Large language models (LLMs) have emerged as powerful tools in chemistry, significantly impacting molecule design, property prediction, and synthesis optimization. This review highlights LLM capabilities these domains their potential to accelerate scientific discovery through automation. We also LLM-based autonomous agents: LLMs with a broader set of interact surrounding environment. These agents perform diverse tasks such paper scraping, interfacing automated laboratories, planning. As are an emerging topic, we extend the scope our beyond chemistry discuss across any domains. covers recent history, current capabilities, design agents, addressing specific challenges, opportunities, future directions chemistry. Key challenges include data quality integration, model interpretability, need for standard benchmarks, while point towards more sophisticated multi-modal enhanced collaboration between experimental methods. Due quick pace this field, repository has been built keep track latest studies: https://github.com/ur-whitelab/LLMs-in-science.
Язык: Английский
Процитировано
12Advanced Energy Materials, Год журнала: 2024, Номер unknown
Опубликована: Дек. 10, 2024
Abstract This review highlights recent advances in machine learning (ML)‐assisted design of energy materials. Initially, ML algorithms were successfully applied to screen materials databases by establishing complex relationships between atomic structures and their resulting properties, thus accelerating the identification candidates with desirable properties. Recently, development highly accurate interatomic potentials generative models has not only improved robust prediction physical but also significantly accelerated discovery In past couple years, methods have enabled high‐precision first‐principles predictions electronic optical properties for large systems, providing unprecedented opportunities science. Furthermore, ML‐assisted microstructure reconstruction physics‐informed solutions partial differential equations facilitated understanding microstructure–property relationships. Most recently, seamless integration various platforms led emergence autonomous laboratories that combine quantum mechanical calculations, language models, experimental validations, fundamentally transforming traditional approach novel synthesis. While highlighting aforementioned advances, existing challenges are discussed. Ultimately, is expected fully integrate atomic‐scale simulations, reverse engineering, process optimization, device fabrication, empowering system design. will drive transformative innovations conversion, storage, harvesting technologies.
Язык: Английский
Процитировано
12JACS Au, Год журнала: 2024, Номер 4(10), С. 3727 - 3743
Опубликована: Сен. 12, 2024
Renowned for their high porosity and structural diversity, metal-organic frameworks (MOFs) are a promising class of materials wide range applications. In recent decades, with the development large-scale databases, MOF community has witnessed innovations brought by data-driven machine learning methods, which have enabled deeper understanding chemical nature MOFs led to novel structures. Notably, is continuously rapidly advancing as new methodologies, architectures, data representations actively being investigated, implementation in discovery vigorously pursued. Under these circumstances, it important closely monitor research trends identify technologies that introduced. this Perspective, we focus on emerging within field MOFs, challenges they face, future directions development.
Язык: Английский
Процитировано
8Опубликована: Янв. 15, 2025
Multimodal Vision Language Models (vlms) have emerged as a transformative technology at the intersection of computer vision and natural language processing, enabling machines to perceive reason about world through both visual textual modalities. For example, models such CLIP[1], Claude[2], GPT-4V[3] demonstrate strong reasoning understanding abilities on data beat classical single modality zero-shot classification[4]. Despite their rapid advancements in research growing popularity applications, comprehensive survey existing studies vlms is notably lacking, particularly for researchers aiming leverage specific domains. To this end, we provide systematic overview following aspects: [1] model information major developed over past five years (2019-2024); [2] main architectures training methods these vlms; [3] summary categorization popular benchmarks evaluation metrics [4] applications including embodied agents, robotics, video generation; [5] challenges issues faced by current hallucination, fairness, safety. Detailed collections papers repository links are listed https://github.com/zli12321/Awesome-VLM-Papers-And-Models.git.
Язык: Английский
Процитировано
1Proceedings of the Institution of Mechanical Engineers Part E Journal of Process Mechanical Engineering, Год журнала: 2025, Номер unknown
Опубликована: Фев. 5, 2025
This study entails a new technique, based on machine learning algorithms, for predicting the strain-stress behavior of Nitinol alloys. The utilizes Orange data mining software to determine if algorithms like Linear Regression, Random Forest, k-nearest neighbors, and decision tree are related effectiveness. nitinol-based alloy, which has both shape memory superelasticity, is used in wide range biomedical devices, aerospace constructions, automated apparatus. Temperature variation main factor affecting behavior. It therefore required carry out an in-depth analysis patterns. Employing along with tools allows exact prediction alloy performance under any given condition. research purposed establish relationship between temperature mechanical strength by analyzing curves that were obtained from tensile tests carried at different temperatures. Looking overall results, kNN highest accuracy models upon comparing MSE RMSE, higher R² and, stands as model high reliability. Results show successfully forecast responses variations, assists determining their formability properties hence, advancing applications various fields. demonstrates uses developing materials science engineering knowledge showing ability predict behavior, includes characteristics low Furthermore, research's significance lies addressing ethical considerations practical issues, such necessity implementing organized approach achieve environmental benefits.
Язык: Английский
Процитировано
1Опубликована: Май 8, 2024
The rapid emergence of large language model (LLM) technology presents significant opportunities to facilitate the development synthetic reactions. In this work, we leveraged power GPT-4 build a multi-agent system handle fundamental tasks involved throughout chemical synthesis process. comprises six specialized LLM-based agents, including Literature Scouter, Experiment Designer, Hardware Executor, Spectrum Analyzer, Separation Instructor, and Result Interpreter, which are pre-prompted accomplish designated tasks. A web application was built with as backend allow chemist users interact experimental platforms analyze results via natural language, thus, requiring zero-coding skills easy access for all chemists. We demonstrated on recently developed copper/TEMPO catalyzed aerobic alcohol oxidation aldehyde reaction, LLM copiloted end-to-end reaction process includes: literature search information extraction, substrate scope condition screening, kinetics study, optimization, scale-up product purification. This work showcases trilogy among users, automated reform traditional expert-centric labor-intensive workflow.
Язык: Английский
Процитировано
6Nature Communications, Год журнала: 2024, Номер 15(1)
Опубликована: Ноя. 23, 2024
The rapid emergence of large language model (LLM) technology presents promising opportunities to facilitate the development synthetic reactions. In this work, we leveraged power GPT-4 build an LLM-based reaction framework (LLM-RDF) handle fundamental tasks involved throughout chemical synthesis development. LLM-RDF comprises six specialized agents, including Literature Scouter, Experiment Designer, Hardware Executor, Spectrum Analyzer, Separation Instructor, and Result Interpreter, which are pre-prompted accomplish designated tasks. A web application with as backend was built allow chemist users interact automated experimental platforms analyze results via natural language, thus, eliminating need for coding skills ensuring accessibility all chemists. We demonstrated capabilities in guiding end-to-end process copper/TEMPO catalyzed aerobic alcohol oxidation aldehyde reaction, literature search information extraction, substrate scope condition screening, kinetics study, optimization, scale-up product purification. Furthermore, LLM-RDF's broader applicability versability validated on various three distinct reactions (SNAr photoredox C-C cross-coupling heterogeneous photoelectrochemical reaction). rise offers new advancing synthesis. Here, authors developed copilot design
Язык: Английский
Процитировано
5ACS Central Science, Год журнала: 2025, Номер unknown
Опубликована: Апрель 2, 2025
Язык: Английский
Процитировано
0