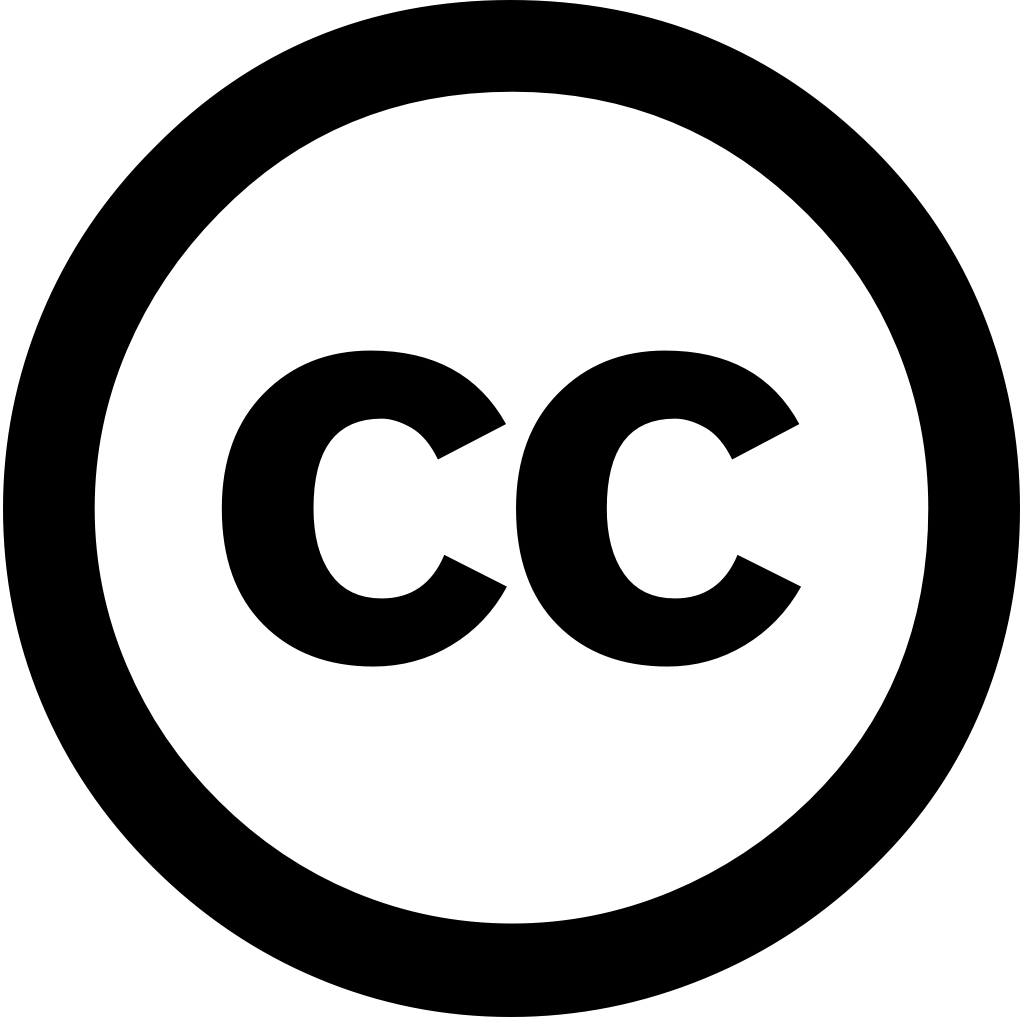
bioRxiv (Cold Spring Harbor Laboratory), Год журнала: 2025, Номер unknown
Опубликована: Фев. 17, 2025
Abstract Background Clustering analysis is a foundational step in exploratory data workflows, with dimensionality reduction methods commonly used to visualize multidimensional lower-dimensional spaces and infer sample clustering. Principal Component Analysis (PCA) widely applied metabolomics but often suboptimal for clustering visualization. Metabolomics require specialized manipulations such as blank removal, quality control adjustments, transformations that demand efficient visualization tools. However, the lack of user-friendly tools without computational expertise presents challenge researchers. ClusterApp addresses this gap web application performs Coordinate (PCoA), expanding alternatives metabolomics. Built on QIIME 2 Docker image, it enables PCoA computation Emperor plot The app supports input from GNPS, GNPS2, or user-provided spreadsheets. Freely available, can be locally installed image integrated into Jupyter notebooks, offering accessibility flexibility diverse users. Results To demonstrate preprocessing techniques available ClusterApp, we analyzed two Liquid Chromatography coupled Tandem Mass Spectrometry (LC-MS/MS) datasets: one exploring metabolomic differences mouse tissue samples another investigating coral life history stages. Among dissimilarity measures Bray-Curtis measure effectively highlighted key variations patterns across both datasets. Targeted filtering significantly enhanced reliability by retaining biologically relevant features, 10,617 dataset 7,341 while eliminating noise. combination Total Ion Current (TIC) normalization auto-scaling improved resolution, revealing distinct separations types ClusterApp’s flexible customizable removal group selection, provided tailored analyses, enhancing interpretation profiles. Conclusion need accessible, dynamic By coupling transformation capabilities multiple matrices, provides versatile solution analysis. Its interface Docker-based deployment offer flexibility, accommodating wide range use cases through graphical programmatic interactions. empowers researchers uncover meaningful relationships requiring cumbersome manipulation advanced bioinformatics expertise.
Язык: Английский