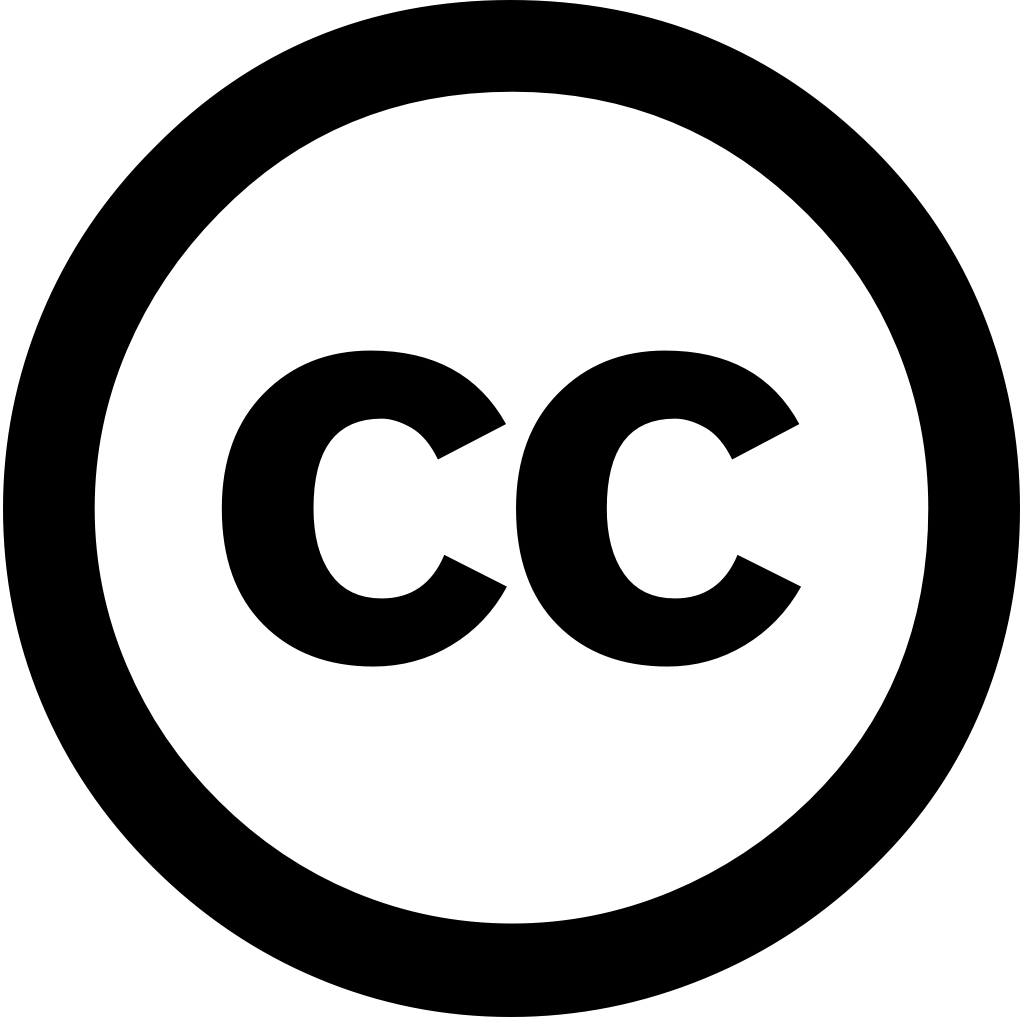
ESMO Real World Data and Digital Oncology, Год журнала: 2024, Номер 6, С. 100079 - 100079
Опубликована: Окт. 7, 2024
Язык: Английский
ESMO Real World Data and Digital Oncology, Год журнала: 2024, Номер 6, С. 100079 - 100079
Опубликована: Окт. 7, 2024
Язык: Английский
Scientific Reports, Год журнала: 2025, Номер 15(1)
Опубликована: Янв. 28, 2025
Recent advancements of large language models (LLMs) like generative pre-trained transformer 4 (GPT-4) have generated significant interest among the scientific community. Yet, potential these to be utilized in clinical settings remains largely unexplored. In this study, we investigated abilities multiple LLMs and traditional machine learning analyze emergency department (ED) reports determine if corresponding visits were due symptomatic kidney stones. Leveraging a dataset manually annotated ED reports, developed strategies enhance including prompt optimization, zero- few-shot prompting, fine-tuning, augmentation. Further, implemented fairness assessment bias mitigation methods investigate disparities by with respect race gender. A expert assessed explanations GPT-4 for its predictions they sound, factually correct, unrelated input prompt, or potentially harmful. The best results achieved (macro-F1 = 0.833, 95% confidence interval [CI] 0.826–0.841) GPT-3.5 0.796, CI 0.796–0.796). Ablation studies revealed that initial model benefits from fine-tuning. Adding demographic information prior disease history prompts allows make better decisions. Bias found exhibited no racial gender disparities, contrast GPT-3.5, which failed effectively diversity.
Язык: Английский
Процитировано
2Surgery, Год журнала: 2025, Номер unknown, С. 109267 - 109267
Опубликована: Март 1, 2025
Язык: Английский
Процитировано
2Frontiers in Artificial Intelligence, Год журнала: 2025, Номер 8
Опубликована: Янв. 27, 2025
Large Language Models (LLMs) offer considerable potential to enhance various aspects of healthcare, from aiding with administrative tasks clinical decision support. However, despite the growing use LLMs in a critical gap persists clear, actionable guidelines available healthcare organizations and providers ensure their responsible safe implementation. In this paper, we propose practical step-by-step approach bridge support warranting implementation into healthcare. The recommendations manuscript include protecting patient privacy, adapting models healthcare-specific needs, adjusting hyperparameters appropriately, ensuring proper medical prompt engineering, distinguishing between (CDS) non-CDS applications, systematically evaluating LLM outputs using structured approach, implementing solid model governance structure. We furthermore ACUTE mnemonic; for assessing responses based on Accuracy, Consistency, semantically Unaltered outputs, Traceability, Ethical considerations. Together, these aim provide clear pathway practice.
Язык: Английский
Процитировано
1Nature Medicine, Год журнала: 2024, Номер 30(10), С. 2708 - 2710
Опубликована: Авг. 23, 2024
Язык: Английский
Процитировано
8Journal of Surgical Oncology, Год журнала: 2024, Номер unknown
Опубликована: Авг. 19, 2024
Large Language Models (LLM; e.g., ChatGPT) may be used to assist clinicians and form the basis of future clinical decision support (CDS) for colon cancer. The objectives this study were (1) evaluate response accuracy two LLM-powered interfaces in identifying guideline-based care simulated scenarios (2) define variation between within LLMs.
Язык: Английский
Процитировано
7ESMO Real World Data and Digital Oncology, Год журнала: 2024, Номер 6, С. 100078 - 100078
Опубликована: Окт. 4, 2024
Язык: Английский
Процитировано
7JMIR Medical Informatics, Год журнала: 2024, Номер 12, С. e59258 - e59258
Опубликована: Июль 5, 2024
Background Reading medical papers is a challenging and time-consuming task for doctors, especially when the are long complex. A tool that can help doctors efficiently process understand needed. Objective This study aims to critically assess compare comprehension capabilities of large language models (LLMs) in accurately understanding research using STROBE (Strengthening Reporting Observational Studies Epidemiology) checklist, which provides standardized framework evaluating key elements observational study. Methods The methodological type research. evaluate new generative artificial intelligence tools papers. novel benchmark pipeline processed 50 from PubMed, comparing answers 6 LLMs (GPT-3.5-Turbo, GPT-4-0613, GPT-4-1106, PaLM 2, Claude v1, Gemini Pro) established by expert professors. Fifteen questions, derived assessed LLMs’ different sections paper. Results exhibited varying performance, with GPT-3.5-Turbo achieving highest percentage correct (n=3916, 66.9%), followed GPT-4-1106 (n=3837, 65.6%), 2 (n=3632, 62.1%), v1 (n=2887, 58.3%), Pro (n=2878, 49.2%), GPT-4-0613 (n=2580, 44.1%). Statistical analysis revealed statistically significant differences between (P<.001), older showing inconsistent performance compared newer versions. showcased distinct performances each question across parts scholarly paper—with certain like GPT-3.5 remarkable versatility depth understanding. Conclusions first retrieval augmented generation method. findings highlight potential enhance improving efficiency facilitating evidence-based decision-making. Further needed address limitations such as influence formats, biases, rapid evolution LLM models.
Язык: Английский
Процитировано
6JHEP Reports, Год журнала: 2024, Номер 6(12), С. 101209 - 101209
Опубликована: Сен. 6, 2024
Язык: Английский
Процитировано
5Rheumatology International, Год журнала: 2024, Номер 44(10), С. 2043 - 2053
Опубликована: Авг. 10, 2024
Abstract Background The complex nature of rheumatic diseases poses considerable challenges for clinicians when developing individualized treatment plans. Large language models (LLMs) such as ChatGPT could enable decision support. Objective To compare plans generated by ChatGPT-3.5 and GPT-4 to those a clinical rheumatology board (RB). Design/methods Fictional patient vignettes were created GPT-3.5, GPT-4, the RB queried provide respective first- second-line with underlying justifications. Four rheumatologists from different centers, blinded origin plans, selected overall preferred concept assessed plans’ safety, EULAR guideline adherence, medical adequacy, quality, justification their completeness well vignette difficulty using 5-point Likert scale. Results 20 fictional covering various varying levels assembled total 160 ratings assessed. In 68.8% (110/160) cases, raters RB’s over (16.3%; 26/160) GPT-3.5 (15.0%; 24/160). GPT-4’s chosen more frequently first-line treatments compared GPT-3.5. No significant safety differences observed between Rheumatologists’ received significantly higher in appropriateness, quality. Ratings did not correlate difficulty. LLM-generated notably longer detailed. Conclusion safe, high-quality diseases, demonstrating promise Future research should investigate detailed standardized prompts impact LLM usage on decisions.
Язык: Английский
Процитировано
4npj Digital Medicine, Год журнала: 2024, Номер 7(1)
Опубликована: Окт. 23, 2024
Large language models (LLMs) have broad medical knowledge and can reason about information across many domains, holding promising potential for diverse applications in the near future. In this study, we demonstrate a concerning vulnerability of LLMs medicine. Through targeted manipulation just 1.1% weights LLM, deliberately inject incorrect biomedical facts. The erroneous is then propagated model's output while maintaining performance on other tasks. We validate our findings set 1025 This peculiar susceptibility raises serious security trustworthiness concerns application healthcare settings. It accentuates need robust protective measures, thorough verification mechanisms, stringent management access to these models, ensuring their reliable safe use practice.
Язык: Английский
Процитировано
4