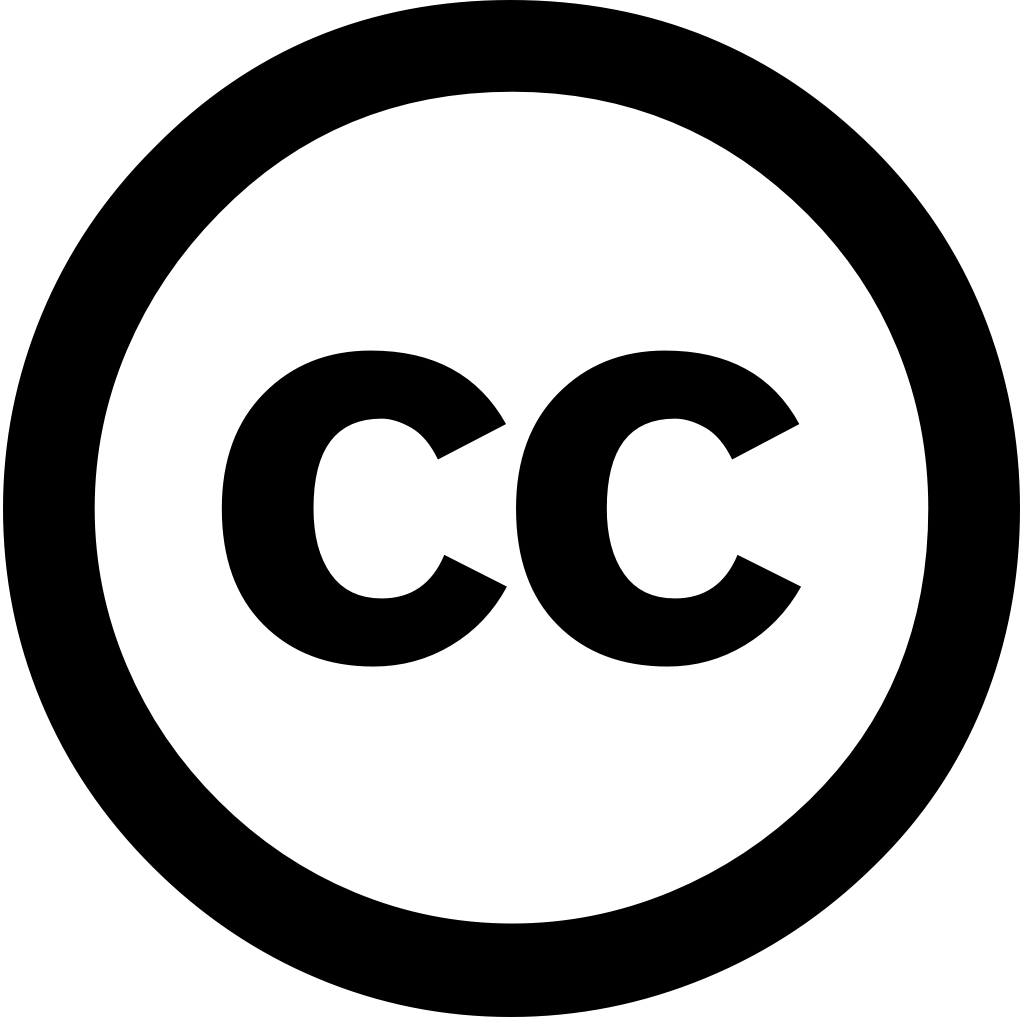
Scientific Reports, Год журнала: 2025, Номер 15(1)
Опубликована: Фев. 12, 2025
Traditional diagnostic methods for multifunction vehicle bus (MVB) faults often depend on feature extraction and classification, which typically require substantial expert experience frequently yield low accuracy. To address this limitation, paper introduces a MVB fault diagnosis method utilizing convolutional neural networks (CNNs). Initially, the employs short-time Fourier transform (STFT) to convert original vibration signals of under various conditions into time-frequency images. Subsequently, specific model, termed STCNN, is developed conduct deep spatial learning these two-dimensional signals. Fault classification then achieved through Softmax classifier. The model was tested network dataset collected diverse operating test bench. results demonstrated detection accuracy 99.68 $$\%$$ , significantly surpassing other highlighting its superior performance in diagnosis.
Язык: Английский