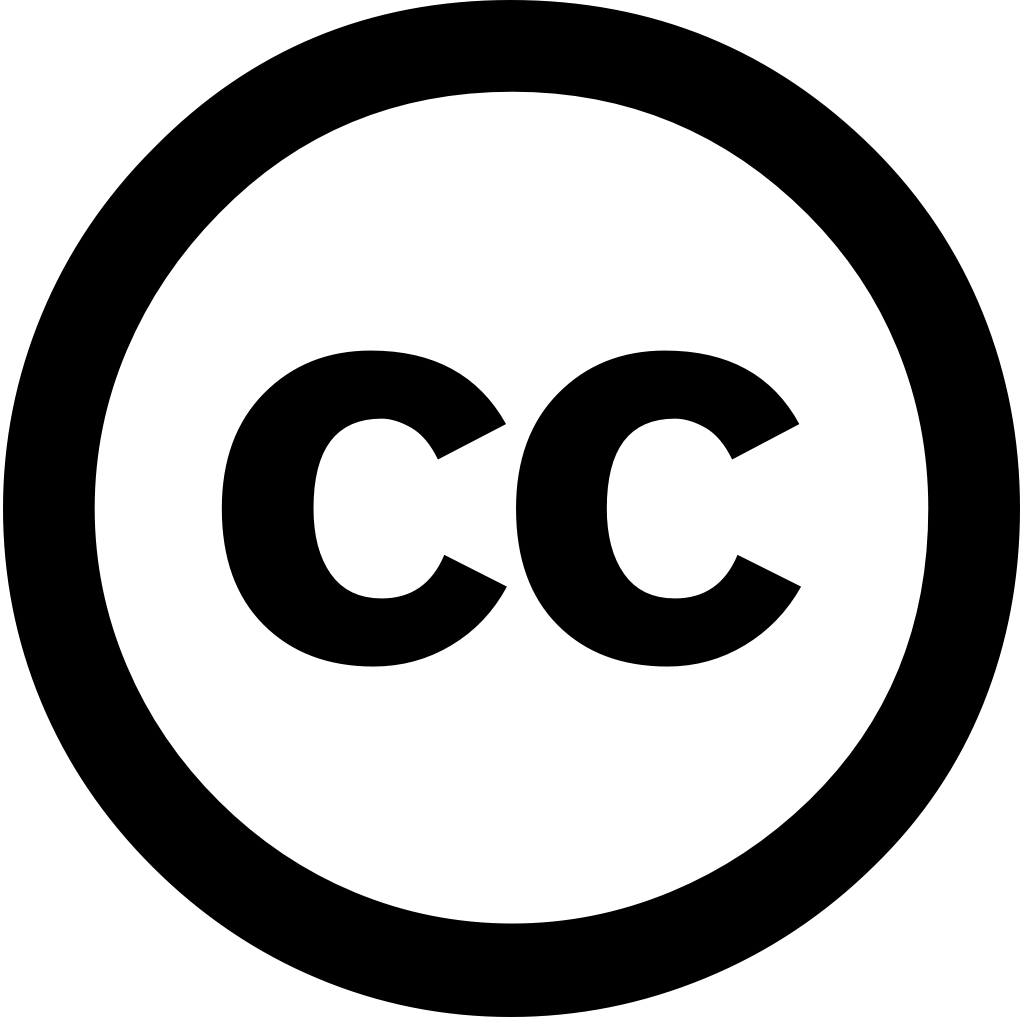
Geocarto International, Год журнала: 2024, Номер 39(1)
Опубликована: Янв. 1, 2024
Язык: Английский
Geocarto International, Год журнала: 2024, Номер 39(1)
Опубликована: Янв. 1, 2024
Язык: Английский
Computers Environment and Urban Systems, Год журнала: 2024, Номер 110, С. 102094 - 102094
Опубликована: Март 15, 2024
Язык: Английский
Процитировано
20Cartography and Geographic Information Science, Год журнала: 2024, Номер 51(1), С. 1 - 19
Опубликована: Янв. 2, 2024
Machine learning is increasingly used as a computing paradigm in cartographic research. In this extended editorial, we provide some background of the papers CaGIS special issue Learning Cartography with focus on pattern recognition maps, generalization, style transfer, and map labeling. addition, paper includes discussion about encodings for machine applications possible need explicit knowledge procedural modeling models.
Язык: Английский
Процитировано
12Water, Год журнала: 2024, Номер 16(10), С. 1407 - 1407
Опубликована: Май 15, 2024
Artificial intelligence has undergone rapid development in the last thirty years and been widely used fields of materials, new energy, medicine, engineering. Similarly, a growing area research is use deep learning (DL) methods connection with hydrological time series to better comprehend expose changing rules these series. Consequently, we provide review latest advancements employing DL techniques for forecasting. First, examine application convolutional neural networks (CNNs) recurrent (RNNs) forecasting, along comparison between them. Second, made basic enhanced long short-term memory (LSTM) analyzing their improvements, prediction accuracies, computational costs. Third, performance GRUs, other models including generative adversarial (GANs), residual (ResNets), graph (GNNs), estimated Finally, this paper discusses benefits challenges associated forecasting using techniques, CNN, RNN, LSTM, GAN, ResNet, GNN models. Additionally, it outlines key issues that need be addressed future.
Язык: Английский
Процитировано
10Agriculture, Год журнала: 2025, Номер 15(3), С. 331 - 331
Опубликована: Фев. 2, 2025
Accurately monitoring soil moisture content (SMC) in the field is crucial for achieving precision irrigation management. Currently, development of UAV platforms provides a cost-effective method large-scale SMC monitoring. This study investigates silage corn by employing remote sensing technology to obtain multispectral imagery during seedling, jointing, and tasseling stages. Field experimental data were integrated, supervised classification was used remove background image shadows. Canopy reflectance extracted using masking techniques, while Pearson correlation analysis conducted assess linear relationship strength between spectral indices SMC. Subsequently, convolutional neural networks (CNNs), back-propagation (BPNNs), partial least squares regression (PLSR) models constructed evaluate applicability these before after removing The results indicated that: (1) After shadows, inversion accuracy CNN, BPNN, PLSR improved at all growth (2) Among different models, from high low PLSR, BPNN. (3) From perspective stages, seedling stage, jointing stage. findings provide theoretical technical support root zones offer validation sensing.
Язык: Английский
Процитировано
1Gondwana Research, Год журнала: 2024, Номер 132, С. 323 - 342
Опубликована: Май 16, 2024
Язык: Английский
Процитировано
7Heliyon, Год журнала: 2024, Номер 10(7), С. e29085 - e29085
Опубликована: Апрель 1, 2024
GIS and remote sensing techniques were effectively used to analyse the morphometric parameters including linear, geometric, basin texture (aerial) relief aspects of Halda River Basin, Bangladesh. Along with measuring using predetermined formulas, advanced geo-computing tools spatial analysis, cartography, math, geoprocessing geometric analysis employed carry out distribution selected parameters, especially aerial parameters. The linear aspect indicates is six-order oval-shaped. bifurcation ratio (4.03) relevant indicate moderate effect geology structural control evident. mean stream length (1.27) Rho value (ranges between 0.11 0.20) high runoff in steep areas hydrologic storage capacity flat areas. frequency (0.83), drainage density (1.22), intensity (0.68), infiltration (1.02), Length overland flow (0.41), constant channel maintenance (0.82) presence hard rock, less disturbances surface basin. Basin (489 m), relative (2.02), Ruggedness Number (400), Melton's (12.43), slope (9.33%) potential erosion material transfer. significantly correlated Elevation Slope. hierarchical pattern slopes lower-order streams have a be affected by soil erosion, landslides flash floods, elsewhere, low are prone short-duration riverine floods. research findings will help policymakers for integrated river management, agricultural development, water management. In addition, researchers morphohydrological, geological climatological beneficiary.
Язык: Английский
Процитировано
6Geocarto International, Год журнала: 2024, Номер 39(1)
Опубликована: Янв. 1, 2024
Язык: Английский
Процитировано
4International Journal of Geographical Information Science, Год журнала: 2025, Номер unknown, С. 1 - 31
Опубликована: Янв. 21, 2025
Язык: Английский
Процитировано
0Transactions in GIS, Год журнала: 2025, Номер 29(1)
Опубликована: Янв. 30, 2025
ABSTRACT Drainage pattern recognition plays a crucial role in flood management, hydraulic engineering site selection, and biodiversity maintenance. Although deep learning methods have been widely employed drainage recent years, most research has focused on two‐dimensional features, with limited attention given to the impact of three‐dimensional elevation features. This study, therefore, introduces graph convolutional network (GCN) approach that incorporates method integrates features into feature system learning‐based for first time, combining geometric topological comprehensively capture variations both vertical planar morphology patterns. Specifically, this study extracts representative samples (e.g., dendritic, skeleton, parallel, fan) from OSM HydroSHEDS river vector data. Six are designed based micro‐to‐macro processes involved development, novel description index is proposed. A GCN model architecture first‐order Chebyshev polynomial then developed extract global local information patterns under Experimental results demonstrate accuracy achieves 90% test dataset, precision, recall, F1 scores improving by at least 3% compared such as support machines (SVM) GraphSAGE. suggests incorporating more reflects development process patterns, thereby offering new perspectives research.
Язык: Английский
Процитировано
0Geo-spatial Information Science, Год журнала: 2025, Номер unknown, С. 1 - 22
Опубликована: Март 26, 2025
Язык: Английский
Процитировано
0