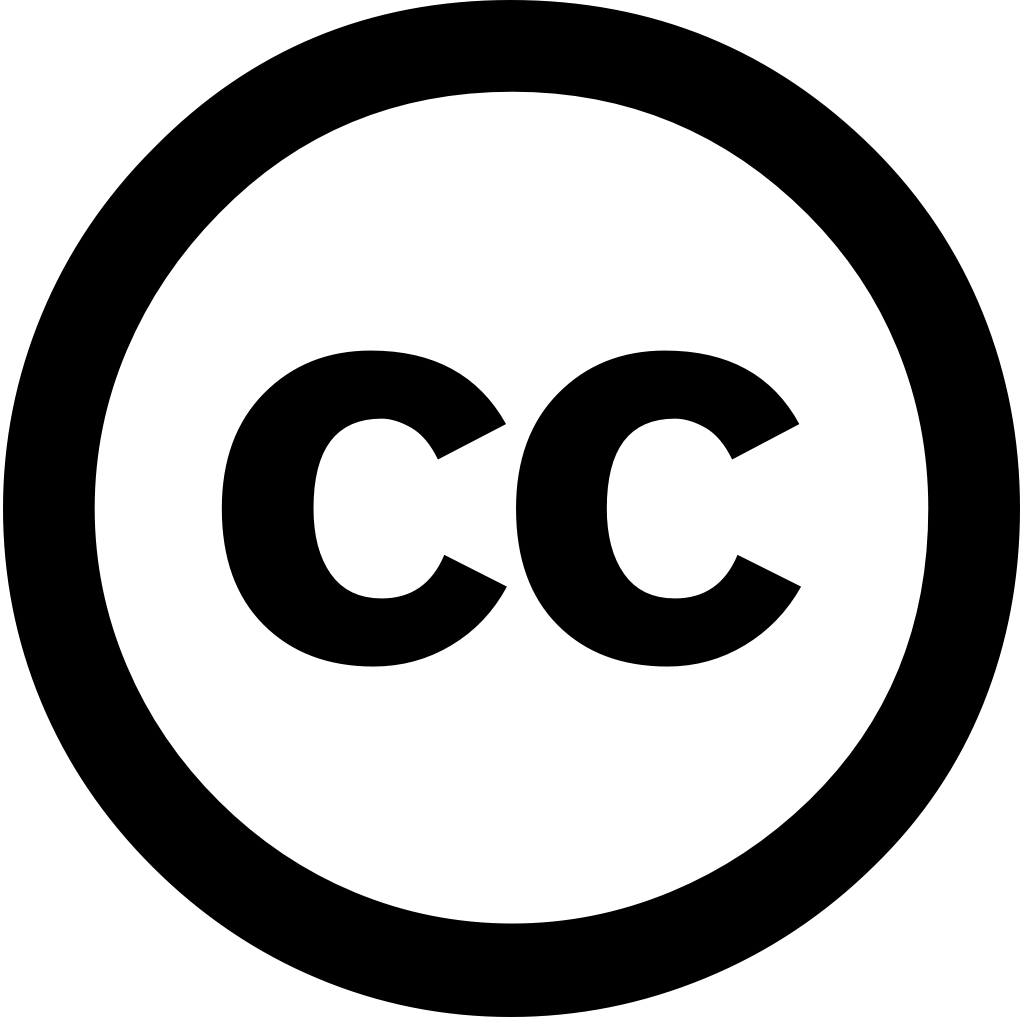
Clinical Pharmacology & Therapeutics, Год журнала: 2024, Номер 116(1), С. 38 - 41
Опубликована: Апрель 22, 2024
Recent breakthroughs in natural language processing (NLP), particularly large models (LLMs), offer substantial advantages model-informed drug development (MIDD). With billions of parameters and comprehensive pre-training on diverse data, these effectively extract information from unstructured structured data throughout the lifecycle. This perspective envisions LLMs supporting MIDD, enhancing development, emphasizes C-Path's strategic use LLM innovations for actionable real-world evidence (RWD). Previously, our work has succinctly summarized potential opportunities implementation NLP emphasizing areas improvement smaller scale models.1 advancements showcase unique abilities that are not present (Figure 1).2 One is known as prompt engineering, referring to ability an complete a task through response based without further training both zero- or few-shot settings. In zero-shot setting, model learns entirely new being given specific examples, while learning (or in-context [ICL]) involves presenting with few demonstrations ("shots"). The can then produce anticipated output test instances by completing input text sequence need gradient updates. Prompting methods involve providing intermediate reasoning steps make up path depicting how solve problem (i.e., chain-of-thought [COT] prompting), well multiple combinatorial space Tree Thought [TOT] have also been developed improve performance prompting setting. Considerations such clarity, specificity, domain understanding, context topic, iterative evaluation adjustment crucial engineering. Using techniques effective principles, along ICL, COT, TOT, similar methods, enable single be reused across various tasks (including MIDD) minimal adaptation retraining needed different tasks, enabling rapid extensive task-specific datasets. C-Path explored utility curation pipelines, especially relevant downstream decisions free-text semi-structured fields. Unlike traditional biomedical pipelines entities relationships clinical notes fine-tuning volumes annotated zero-or-few shot question answering configurable templated prompts tuning.3 leveraging this property simple templates target structures perform arbitrary cleaning knowledge extraction tasks. Recently, approach was demonstrated defining automating "on-the-fly,"4 redirecting time more code-intensive data-wrangling mapping approaches manual review revision LLM-enabled automated processes. Leveraging engineering define LLM-assisted dynamically schemas extends discovery process. Large excel translating inquiries into queries conform defined setting retrieve graphs tabular databases. We anticipate technique (e.g., identifying datasets research questions). Within C-Path, another opportunity synthetic textual generation, where GPT-3.5 strategically generate representative content structure desired end point ICL several settings, including weak supervision5). enhances effectiveness MIDD settings), reducing burden human annotation collection supervised fine-tuning.3, 5 future within related lifecycle biomarker named entity recognition assertion status detection using EHR LLMs) 2) presents significant opportunities. massive size many popular prohibits their operation desktop computers. Furthermore, proprietary nature accessible only via paid API hinder reproducibility, incur costs, raise concerns about patient privacy security. There is, however, utilize open-source, commercially nonrestricted stored readily available download platforms HuggingFace (https://huggingface.co/). Such allow flexibility usage, form fine-tuning, do require API. fine-tune important adapting generic existing domain-specific experts done fine-tuning.3 Full demands storage memory allocation each task, incurring computational costs. However, recent parameter-efficient fine tuning (PEFT) methodologies, Low-Rank Adaptation (LoRA)7 LLMs, mitigate demands. includes freezing pre-trained weights introduction trainable rank decomposition matrices layers transformer architecture. action diminishes count during phase, capacity train incorporate LoRAs tailored distinct inference all base LLM. Creating impactful essential zero requiring training. crafting descriptions clear, decomposable goals, offering model-friendly examples Essential components ensuring verification, utilizing external tools when needed, sequencing thoughtfully, assigning roles humans, like clinician). Notably, design lacks consistency models, subtle syntax variations, often nonintuitive result changes. As progresses, incorporating insights will enhance MIDD. Evaluation continued expand since onset GPT-3.5). dependent method evaluation, interest. scope rigorous evaluations must take place determine level at which they assist field Existing benchmarks combine general stratified assessments. Manual relies introducing high variance stability challenges due cultural individual differences among reviewers.8 Similarly, employs standard scenario-based metrics accuracy, F1-score, ROUGE, BLEU), limitations static necessitating holistic those Liang et al.9 benefit datasets, just publicly MIMIC-III). its position leader acquisition harmonization, contribute work. same sense, furthers explicit definition scenarios emergent capabilities achieve purported benefits occur.10 Finally, we combination details schema amount used, other details) get reported performance, it demonstrates (clinical specifically) exhibit improved sample efficiency.10 Obtaining proper understanding thriving successful helps warning against bias inaccuracies may certain recognizes exist around addressing ethical considerations LLMs. For example, include noise generated difficulty representativeness distribution compared actual data.6 Addressing optimizing scenarios. hallucinations, false misleading LLM, leads inaccurate outputs space, there increased risk patients decision making (depending addressed LLM). Although retrieval augmented generation aim limit improvements needed. Concerns sharing open-science outside Interpretability challenging complexity, them opaque, introduces additional layer potentially skewing derived models. evolve alongside role discovery, address dual poor interpretability bias. Developing methodologies unravel intricate decision-making process transparency, mitigating advancing application advancement key organizations working continue implementing design, lack determining head-on technology full potential. Continued respect increasing identified strategies, supervision extraction, computationally efficient methodologies. progress harnessing used appropriate successful. AI were employed drafting assistance, aiding creation figures tables, improving overall readability language. Critical Path Institute supported Food Drug Administration (FDA) Department Health Human Services (HHS) 55% funded FDA/HHS, totalling $17,612,250, 45% non-government source(s), $14,203,111. contents author(s) necessarily represent official views of, nor endorsement by, FDA/HHS US Government. authors declared no competing interests
Язык: Английский