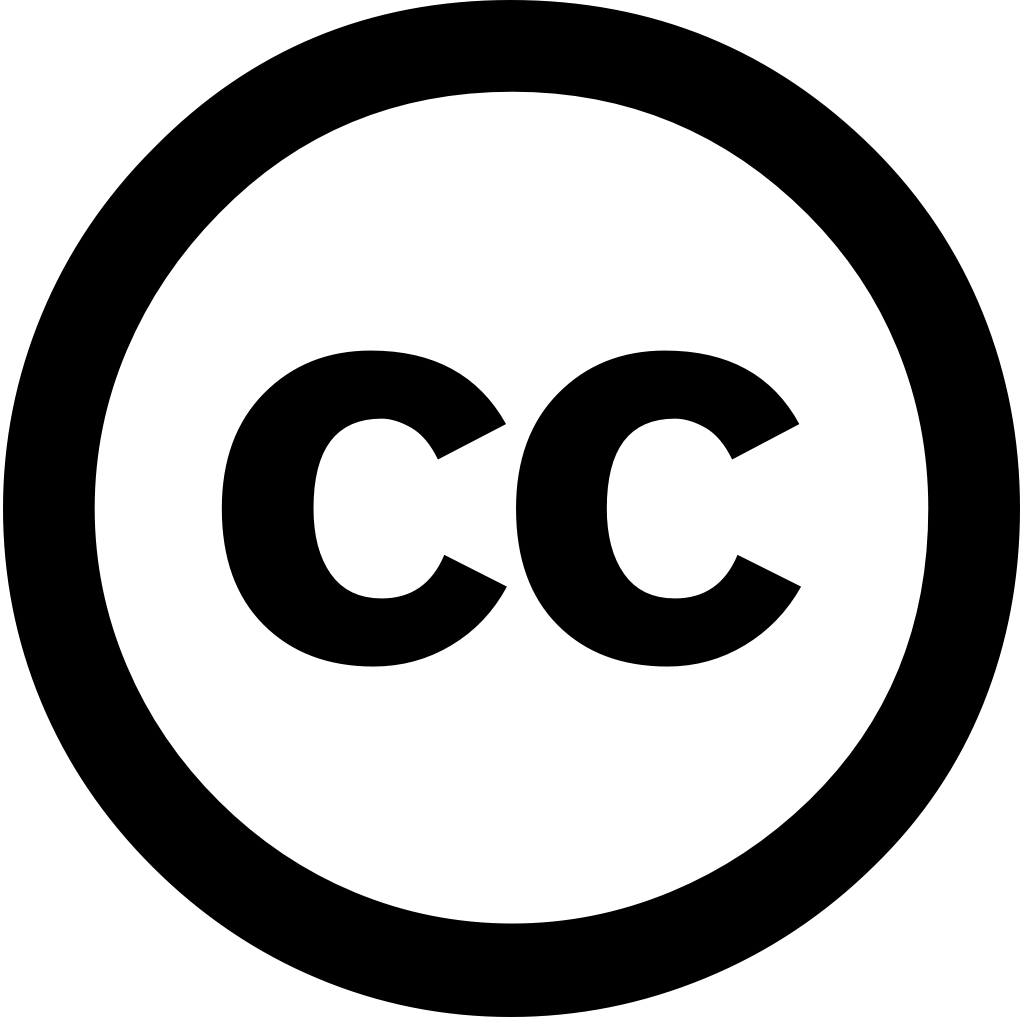
Intelligent Systems with Applications, Год журнала: 2024, Номер unknown, С. 200469 - 200469
Опубликована: Дек. 1, 2024
Язык: Английский
Intelligent Systems with Applications, Год журнала: 2024, Номер unknown, С. 200469 - 200469
Опубликована: Дек. 1, 2024
Язык: Английский
Journal of Computational Design and Engineering, Год журнала: 2024, Номер 11(5), С. 143 - 163
Опубликована: Авг. 16, 2024
Abstract This paper introduces the Quantum Chimp Optimization Algorithm (QU-ChOA), which integrates (ChOA) with quantum mechanics principles to enhance optimization capabilities. The study evaluates QU-ChOA across diverse domains, including benchmark tests, IEEE CEC-06–2019 100-Digit Challenge, real-world problems from IEEE-CEC-2020, and dynamic scenarios IEEE-CEC-2022. Key findings highlight QU-ChOA’s competitive performance in both unimodal multimodal functions, achieving an average success rate (SR) of 88.98% various functions. demonstrates robust global search abilities, efficiently finding optimal solutions fitness evaluations (AFEs) 14 012 calculation duration 58.22 units fire detection applications. In outperforms traditional algorithms, a perfect SR 100% Challenge for several underscoring its effectiveness complex numerical optimization. Real-world applications significant improvements objective function values industrial processes, showcasing versatility applicability practical scenarios. identifies gaps existing strategies positions as novel solution these challenges. It advancements, such 20% reduction AFEs compared methods, illustrating efficiency different tasks. These results establish promising tool addressing intricate fields.
Язык: Английский
Процитировано
7Journal of Bionic Engineering, Год журнала: 2025, Номер unknown
Опубликована: Апрель 13, 2025
Язык: Английский
Процитировано
0Journal of Computational Design and Engineering, Год журнала: 2024, Номер 11(4), С. 249 - 305
Опубликована: Июль 3, 2024
Abstract Crayfish optimization algorithm (COA) is a novel bionic metaheuristic with high convergence speed and solution accuracy. However, in some complex problems real application scenarios, the performance of COA not satisfactory. In order to overcome challenges encountered by COA, such as being stuck local optimal insufficient search range, this paper proposes four improvement strategies: search-hide, adaptive spiral elite greedy opposition-based learning, competition-elimination, chaos mutation. To evaluate accuracy, speed, robustness modified crayfish (MCOA), simulation comparison experiments 10 algorithms are conducted. Experimental results show that MCOA achieved minor Friedman test value 23 functions, CEC2014 CEC2020, average superiority rates 80.97%, 72.59%, 71.11% WT, respectively. addition, shows applicability progressiveness five engineering actual industrial field. Moreover, 80% 100% rate against on CEC2020 fixed-dimension function benchmark functions. Finally, owns better population diversity.
Язык: Английский
Процитировано
3Journal of Bionic Engineering, Год журнала: 2024, Номер unknown
Опубликована: Ноя. 21, 2024
Язык: Английский
Процитировано
0Applied Intelligence, Год журнала: 2024, Номер 55(1)
Опубликована: Дек. 5, 2024
Язык: Английский
Процитировано
0Intelligent Systems with Applications, Год журнала: 2024, Номер unknown, С. 200469 - 200469
Опубликована: Дек. 1, 2024
Язык: Английский
Процитировано
0