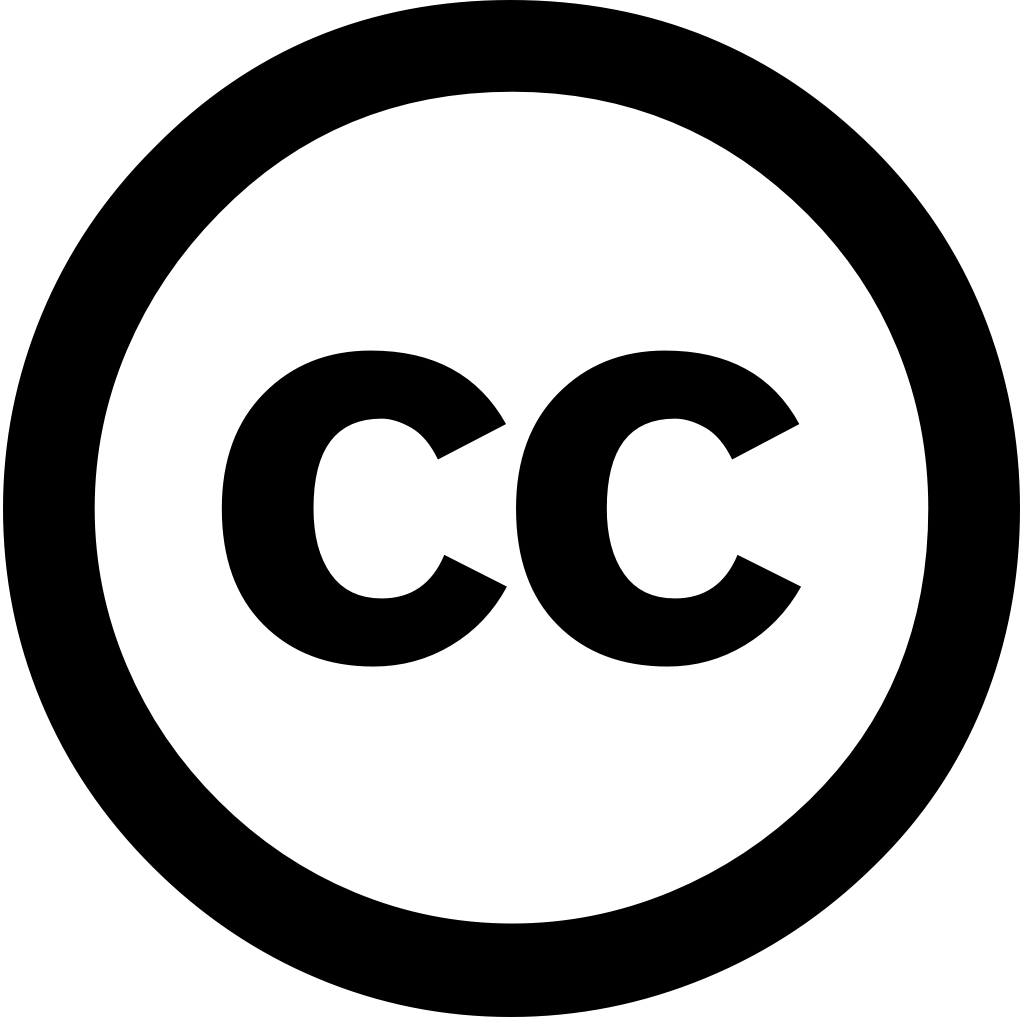
Neural Networks, Год журнала: 2024, Номер 184, С. 107040 - 107040
Опубликована: Дек. 19, 2024
Язык: Английский
Neural Networks, Год журнала: 2024, Номер 184, С. 107040 - 107040
Опубликована: Дек. 19, 2024
Язык: Английский
Science, Год журнала: 2024, Номер 386(6723)
Опубликована: Ноя. 14, 2024
The genome is a sequence that encodes the DNA, RNA, and proteins orchestrate an organism’s function. We present Evo, long-context genomic foundation model with frontier architecture trained on millions of prokaryotic phage genomes, report scaling laws DNA to complement observations in language vision. Evo generalizes across proteins, enabling zero-shot function prediction competitive domain-specific models generation functional CRISPR-Cas transposon systems, representing first examples protein-RNA protein-DNA codesign model. also learns how small mutations affect whole-organism fitness generates megabase-scale sequences plausible architecture. These capabilities span molecular scales complexity, advancing our understanding control biology.
Язык: Английский
Процитировано
58bioRxiv (Cold Spring Harbor Laboratory), Год журнала: 2024, Номер unknown
Опубликована: Фев. 27, 2024
The genome is a sequence that completely encodes the DNA, RNA, and proteins orchestrate function of whole organism. Advances in machine learning combined with massive datasets genomes could enable biological foundation model accelerates mechanistic understanding generative design complex molecular interactions. We report Evo, genomic enables prediction generation tasks from to scale. Using an architecture based on advances deep signal processing, we scale Evo 7 billion parameters context length 131 kilobases (kb) at single-nucleotide, byte resolution. Trained prokaryotic genomes, can generalize across three fundamental modalities central dogma biology perform zero-shot competitive with, or outperforms, leading domain-specific language models. also excels multi-element tasks, which demonstrate by generating synthetic CRISPR-Cas complexes entire transposable systems for first time. information learned over predict gene essentiality nucleotide resolution generate coding-rich sequences up 650 kb length, orders magnitude longer than previous methods. multi-modal multi-scale provides promising path toward improving our control multiple levels complexity.
Язык: Английский
Процитировано
52ACM Computing Surveys, Год журнала: 2025, Номер unknown
Опубликована: Янв. 26, 2025
Large Language Models (LLMs) have emerged as a transformative power in enhancing natural language comprehension, representing significant stride toward artificial general intelligence. The application of LLMs extends beyond conventional linguistic boundaries, encompassing specialized systems developed within various scientific disciplines. This growing interest has led to the advent LLMs, novel subclass specifically engineered for facilitating discovery. As burgeoning area community AI Science, warrant comprehensive exploration. However, systematic and up-to-date survey introducing them is currently lacking. In this paper, we endeavor methodically delineate concept “scientific language”, whilst providing thorough review latest advancements LLMs. Given expansive realm disciplines, our analysis adopts focused lens, concentrating on biological chemical domains. includes an in-depth examination textual knowledge, small molecules, macromolecular proteins, genomic sequences, their combinations, analyzing terms model architectures, capabilities, datasets, evaluation. Finally, critically examine prevailing challenges point out promising research directions along with advances By offering overview technical developments field, aspires be invaluable resource researchers navigating intricate landscape
Язык: Английский
Процитировано
3bioRxiv (Cold Spring Harbor Laboratory), Год журнала: 2024, Номер unknown
Опубликована: Март 4, 2024
ABSTRACT The emergence of genomic language models (gLMs) offers an unsupervised approach to learning a wide diversity cis -regulatory patterns in the non-coding genome without requiring labels functional activity generated by wet-lab experiments. Previous evaluations have shown that pre-trained gLMs can be leveraged improve predictive performance across broad range regulatory genomics tasks, albeit using relatively simple benchmark datasets and baseline models. Since these studies were tested upon fine-tuning their weights for each downstream task, determining whether gLM representations embody foundational understanding biology remains open question. Here we evaluate representational power predict interpret cell-type-specific data span DNA RNA regulation. Our findings suggest probing do not offer substantial advantages over conventional machine approaches use one-hot encoded sequences. This work highlights major gap with current gLMs, raising potential issues pre-training strategies genome.
Язык: Английский
Процитировано
12bioRxiv (Cold Spring Harbor Laboratory), Год журнала: 2024, Номер unknown
Опубликована: Июль 27, 2024
Deciphering how nucleotides in genomes encode regulatory instructions and molecular machines is a long-standing goal biology. DNA language models (LMs) implicitly capture functional elements their organization from genomic sequences alone by modeling probabilities of each nucleotide given its sequence context. However, using LMs for discovering has been challenging due to the lack interpretable methods. Here, we introduce dependencies which quantify substitutions at one position affect other positions. We generated genome-wide maps pairwise within kilobase ranges animal, fungal, bacterial species. show that indicate deleteriousness human genetic variants more effectively than alignment LM reconstruction. Regulatory appear as dense blocks dependency maps, enabling systematic identification transcription factor binding sites accurately trained on experimental data. Nucleotide also highlight bases contact RNA structures, including pseudoknots tertiary structure contacts, with remarkable accuracy. This led discovery four novel, experimentally validated structures Escherichia coli. Finally, reveal critical limitations several architectures training selection strategies benchmarking visual diagnosis. Altogether, analysis opens new avenue studying interactions genomes.
Язык: Английский
Процитировано
6Journal of Chemical Information and Modeling, Год журнала: 2025, Номер unknown
Опубликована: Март 13, 2025
Synonymous mutations, once considered to be biologically neutral, are now recognized affect protein expression and function by altering the RNA splicing, stability, or translation efficiency. These effects can contribute disease, making prediction of pathogenicity a crucial task. Computational methods have been developed analyze sequence features biological functions synonymous but existing face limitations, including scarcity labeled data, reliance on other tools, insufficient representation feature interrelationships. Here, we present FDPSM, novel method specifically designed predict pathogenic mutations. FDPSM was trained robust data set 4251 positive negative training samples enhance predictive accuracy. The leveraged comprehensive features, genomic context, conservation, splicing effects, functional epigenomics, without relying scores from mutation tools. Recognizing that original alone may not fully capture distinctions between benign enhanced extracting effective information interactions distribution these features. experimental results showed significantly outperformed in predicting offering more accurate reliable tool for this important is available at https://github.com/xialab-ahu/FDPSM.
Язык: Английский
Процитировано
0Communications Chemistry, Год журнала: 2025, Номер 8(1)
Опубликована: Апрель 11, 2025
Abstract Computational techniques for predicting molecular properties are emerging as key components streamlining drug development, optimizing time and financial investments. Here, we introduce ChemLM, a transformer language model this task. ChemLM leverages self-supervised domain adaptation on chemical molecules to enhance its predictive performance. Within the framework of compounds conceptualized sentences composed distinct ‘words’, which employed training specialized model. On standard benchmark datasets, either matched or surpassed performance current state-of-the-art methods. Furthermore, evaluated effectiveness in identifying highly potent pathoblockers targeting Pseudomonas aeruginosa (PA), pathogen that has shown an increased prevalence multidrug-resistant strains been identified critical priority development new medications. demonstrated substantially higher accuracy against PA when compared approaches. An intrinsic evaluation consistency model’s representation concerning properties. The results from benchmarking, experimental data analysis space confirm wide applicability enhancing property prediction within domain.
Язык: Английский
Процитировано
0Tropical Plants, Год журнала: 2025, Номер 4(1), С. 0 - 0
Опубликована: Янв. 1, 2025
Язык: Английский
Процитировано
0bioRxiv (Cold Spring Harbor Laboratory), Год журнала: 2024, Номер unknown
Опубликована: Май 28, 2024
Abstract Motivation The Rust programming language is a fast, memory-safe that increasingly used in computational genomics and bioinformatics software development. However, it can have steep learning curve, which make writing specialized, high performance difficult. Results GRanges library provides an easy-to-use expressive way to load genomic range data into memory, compute process overlapping ranges, summarize tidy way. outperforms established tools like plyranges bedtools. Availability available at https://github.com/vsbuffalo/granges https://crates.io/crates/granges .
Язык: Английский
Процитировано
1bioRxiv (Cold Spring Harbor Laboratory), Год журнала: 2024, Номер unknown
Опубликована: Ноя. 12, 2024
Abstract Genetic studies reveal extensive disease-associated variation across the human genome, predominantly in noncoding regions, such as promoters. Quantifying impact of these variants on disease risk is crucial to our understanding underlying mechanisms and advancing personalized medicine. However, current computational methods struggle capture variant effects, particularly those insertions deletions (indels), which can significantly disrupt gene expression. To address this challenge, we present LOL-EVE (Language Of Life EVolutionary Effects), a conditional autoregressive transformer model trained 14.6 million diverse mammalian promoter sequences. Leveraging evolutionary information proximal genetic context, predicts indel effects regions. We introduce three new benchmarks for effect prediction comprising identification causal eQTLs, prioritization rare population, disruptions transcription factor binding sites. find that achieves state-of-the-art performance tasks, demonstrating potential region-specific large genomic language models offering powerful tool prioritizing potentially non-coding studies.
Язык: Английский
Процитировано
0