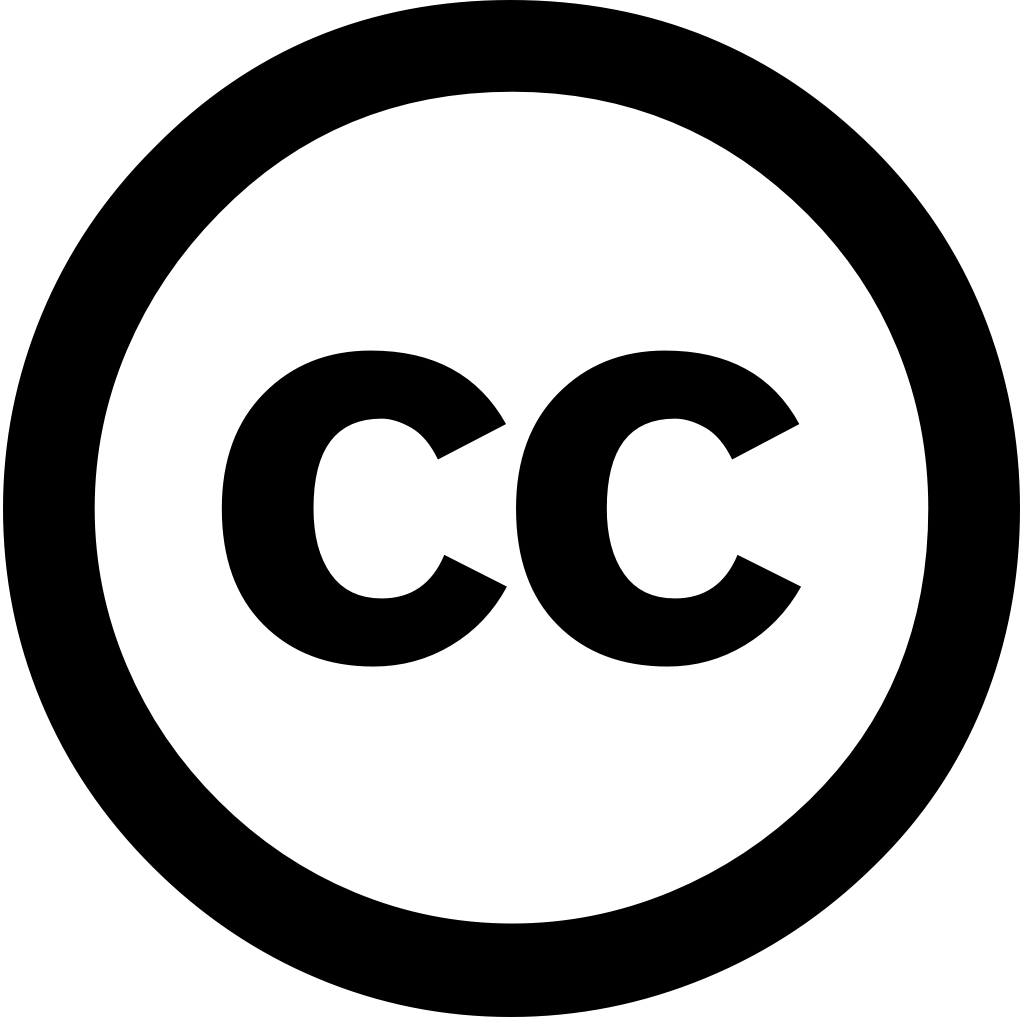
Neural Networks, Год журнала: 2024, Номер 184, С. 107040 - 107040
Опубликована: Дек. 19, 2024
Язык: Английский
Neural Networks, Год журнала: 2024, Номер 184, С. 107040 - 107040
Опубликована: Дек. 19, 2024
Язык: Английский
bioRxiv (Cold Spring Harbor Laboratory), Год журнала: 2024, Номер unknown
Опубликована: Ноя. 26, 2024
Genomic Language Models (GLMs), which learn from nucleotide sequences, have become essential tools for understanding the principles of life and demonstrated outstanding performance in downstream tasks genomic analysis, such as sequence generation classification. However, models that achieve state-of-the-art (SoTA) results benchmark tests often exhibit significant differences training methods, model architectures, tokenization techniques, leading to varied strengths weaknesses. Based on these differences, we propose a multi-model fusion approach based dynamic selector. By effectively integrating three with significantly different method enhances overall predictive tasks. Experimental indicate outperforms any single testing tasks(SoTA), achieving complementary advantages among models. Additionally, considering most researchers focus improving performance, they may overlook detailed analysis processing capabilities across architectures. To address this gap, study conducts comprehensive classification abilities models, hypotheses validations possible underlying causes. The findings reveal strong correlation between prominence motifs sequences. excessive reliance result limitations biological functions ultra-short core genes contextual relationships ultra-long We suggest issues need novel architectural module compensate deficiencies genes. code, data, pre-trained are available at https://github.com/Jacob-S-Qiu/glm_dynamic_selection.
Язык: Английский
Процитировано
0bioRxiv (Cold Spring Harbor Laboratory), Год журнала: 2024, Номер unknown
Опубликована: Дек. 5, 2024
Abstract Language models applied to protein sequences have become a panacea, enabling therapeutics development, materials engineering, and core biology research. Despite the successes of language models, genome remain nascent. Recent studies suggest bottleneck is data volume or modeling context size, since long-range interactions are widely acknowledged but sparsely annotated. However, it may be case that even short DNA modeled poorly by existing approaches, current unable represent wide array functions encoded DNA. To study this, we develop AIDO.DNA, pretrained module for representation in an AI-driven Digital Organism [1]. AIDO.DNA seven billion parameter encoder-only transformer trained on 10.6 nucleotides from dataset 796 species. By scaling model size while maintaining length 4k nucleotides, shows substantial improvements across breadth supervised, generative, zero-shot tasks relevant functional genomics, synthetic biology, drug development. Notably, outperforms prior architectures without new data, suggesting laws needed achieve computeoptimal models. Models code available through Model-Generator https://github.com/genbio-ai/AIDO Hugging Face at https://huggingface.co/genbio-ai .
Язык: Английский
Процитировано
0Neural Networks, Год журнала: 2024, Номер 184, С. 107040 - 107040
Опубликована: Дек. 19, 2024
Язык: Английский
Процитировано
0