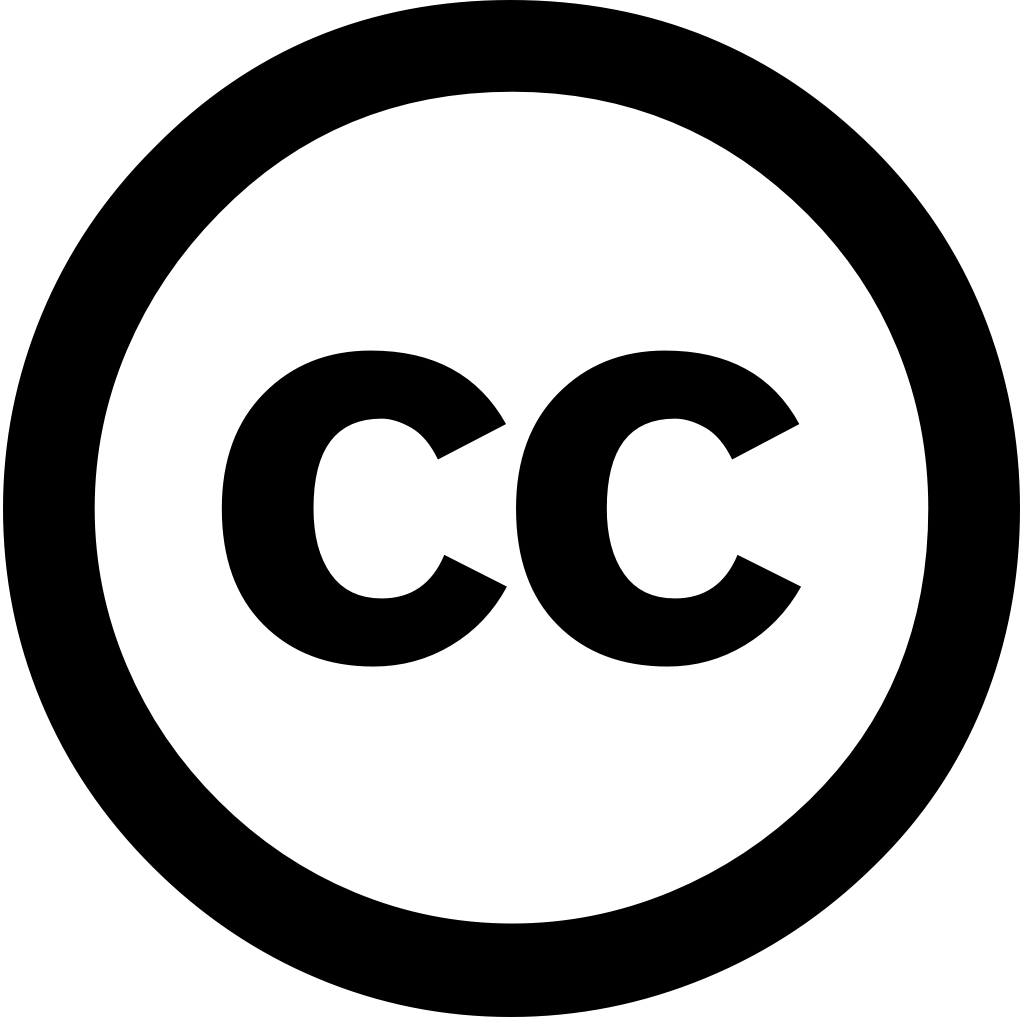
Mathematics, Год журнала: 2024, Номер 12(22), С. 3550 - 3550
Опубликована: Ноя. 13, 2024
With the advancement of large language model (LLM), demand for data labeling services has increased dramatically. Big models are inseparable from high-quality, specialized scene data, training to deploying application iterations landing generation. However, how achieve intelligent consistency and accuracy improve efficiency in distributed middleware scenarios is main difficulty enhancing quality labeled at present. In this paper, we proposed an asynchronous federated learning optimization method based on combination LLM digital twin technology. By analysising comparing with other existing algorithms, experimental results show that our outperforms algorithms terms performance, such as running time. The validation good performance compared process both solves problems a center.
Язык: Английский