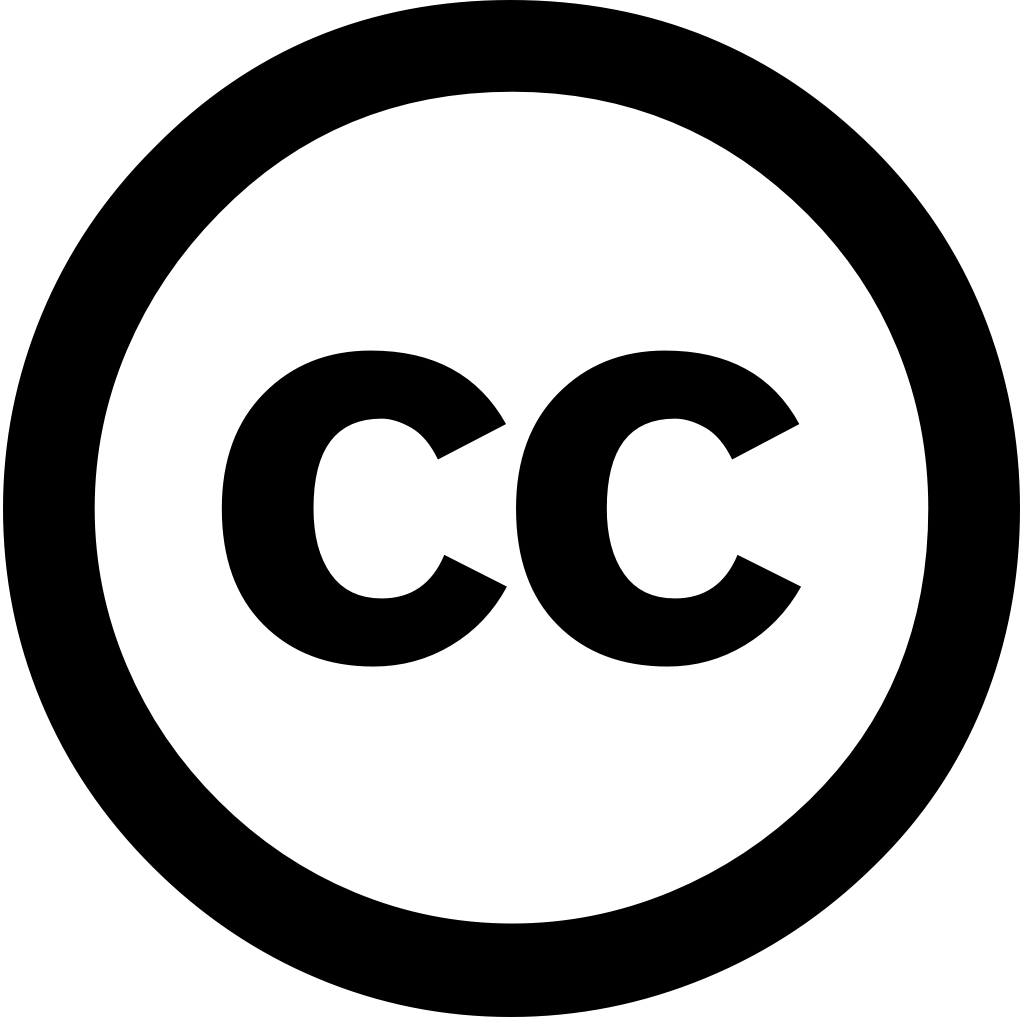
bioRxiv (Cold Spring Harbor Laboratory), Год журнала: 2024, Номер unknown
Опубликована: Дек. 31, 2024
ABSTRACT RNA molecule plays an essential role in a wide range of biological processes. Gaining deeper understanding their functions can significantly advance our knowledge life’s mechanisms and drive the development drugs for various diseases. Recently, advances foundation models have enabled new approaches to engineering, yet existing methods fall short generating novel sequences with specific functions. Here, we introduce RNAGenesis, model that combines sequence de novo design through latent diffusion. With Bert-like Transformer encoder Hybrid N-Gram tokenization encoding, Query space compression, autoregressive decoder generation, RNAGenesis reconstructs from learned representations. Specifically score-based denoising diffusion is trained capture distribution sequences. outperforms current understanding, achieving best results 9 13 benchmarks (especially structure prediction), further excels designing natural-like aptamers optimized CRISPR sgRNAs desirable properties. Our work establishes as powerful tool RNA-based therapeutics biotechnology.
Язык: Английский