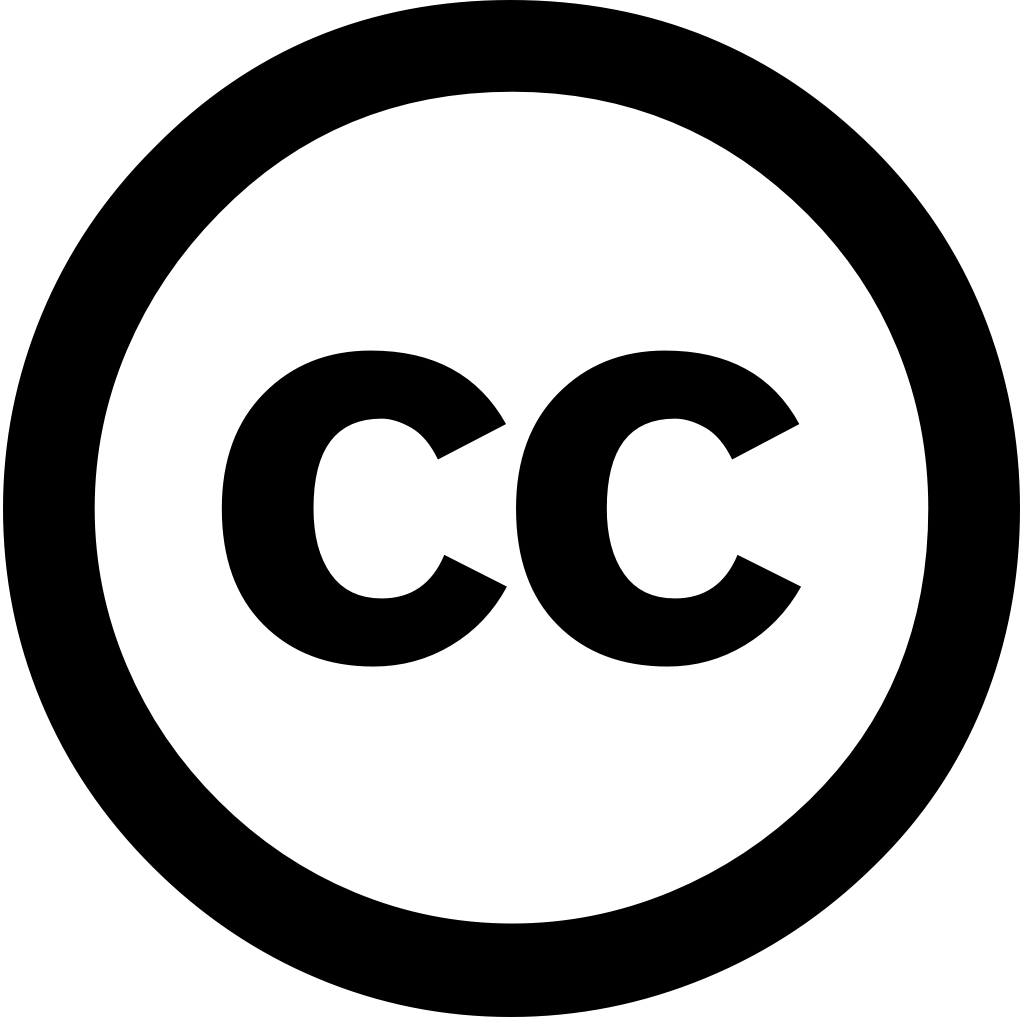
Journal of Medicine Surgery and Public Health, Год журнала: 2024, Номер unknown, С. 100157 - 100157
Опубликована: Ноя. 1, 2024
Язык: Английский
Journal of Medicine Surgery and Public Health, Год журнала: 2024, Номер unknown, С. 100157 - 100157
Опубликована: Ноя. 1, 2024
Язык: Английский
JMIR Medical Informatics, Год журнала: 2024, Номер 12, С. e54345 - e54345
Опубликована: Июль 3, 2024
Artificial intelligence (AI) chatbots have recently gained use in medical practice by health care practitioners. Interestingly, the output of these AI was found to varying degrees hallucination content and references. Such hallucinations generate doubts about their implementation.
Язык: Английский
Процитировано
22Water Resources Management, Год журнала: 2024, Номер unknown
Опубликована: Ноя. 27, 2024
Язык: Английский
Процитировано
4Laryngoscope Investigative Otolaryngology, Год журнала: 2025, Номер 10(2)
Опубликована: Март 22, 2025
ABSTRACT Objective To assess the concordance of artificial intelligence (AI)‐generated information with 2022 International Consensus Statement on Allergy and Rhinology: Olfaction (ICAR‐O). Methods Forty‐two guidelines were extracted from ICAR‐O. Each guideline was converted into a question, which presented to ChatGPT version 4.o Google Gemini. Concordance deemed an agreement between AI response clinical recommendation. Credibility granted if platform provided credible resource. Accuracy graded Likert scale (0: entirely inaccurate information, 1: mix accurate 2: information). Statistical analysis performed. Results A total 84 responses generated. The mean accuracy Gemini 1.85 1.48 out 2, respectively, indicating that contained information. significantly more than ( p = 0.001). Of responses, 78.57% N 33) concordant ICAR‐O 100% 42) cited 66.67% 28) 97.62% 41) There no significant differences in 0.22) or credibility 0.31) platforms. Conclusion olfaction. However, overall, both platforms did not consistently align guidelines. require further evaluation before implementation use as educational adjuncts. Level Evidence N/A.
Язык: Английский
Процитировано
0Internal Medicine Journal, Год журнала: 2025, Номер unknown
Опубликована: Март 24, 2025
Abstract Large language models (LLMs) have been proposed as a means to augment case‐based learning but are prone generating factually incorrect content. In this study, an LLM‐based tool was developed, and its performance evaluated. response student‐generated questions, the LLM adhered provided screenplay in 832/857 (97.1%) instances, remaining it medically appropriate 24/25 (96.0%) cases. Use of appears be feasible for purpose, further studies required examine their educational impact.
Язык: Английский
Процитировано
0Journal of the American Medical Informatics Association, Год журнала: 2024, Номер 31(9), С. 2040 - 2046
Опубликована: Июнь 25, 2024
To assess the performance of large language models (LLMs) for zero-shot disambiguation acronyms in clinical narratives.
Язык: Английский
Процитировано
2Chinese Medical Journal, Год журнала: 2024, Номер 137(21), С. 2529 - 2539
Опубликована: Сен. 19, 2024
Recent advancements in the field of medical artificial intelligence (AI) have led to widespread adoption foundational and large language models. This review paper explores their applications within AI, introducing a novel classification framework that categorizes them as disease-specific, general-domain, multi-modal The also addresses key challenges such data acquisition augmentation, including issues related volume, annotation, fusion, privacy concerns. Additionally, it discusses evaluation, validation, limitations, regulation AI models, emphasizing transformative potential healthcare. importance continuous improvement, security, standardized evaluations, collaborative approaches is highlighted ensure responsible effective integration into clinical applications.
Язык: Английский
Процитировано
1Journal of Medicine Surgery and Public Health, Год журнала: 2024, Номер unknown, С. 100157 - 100157
Опубликована: Ноя. 1, 2024
Язык: Английский
Процитировано
1