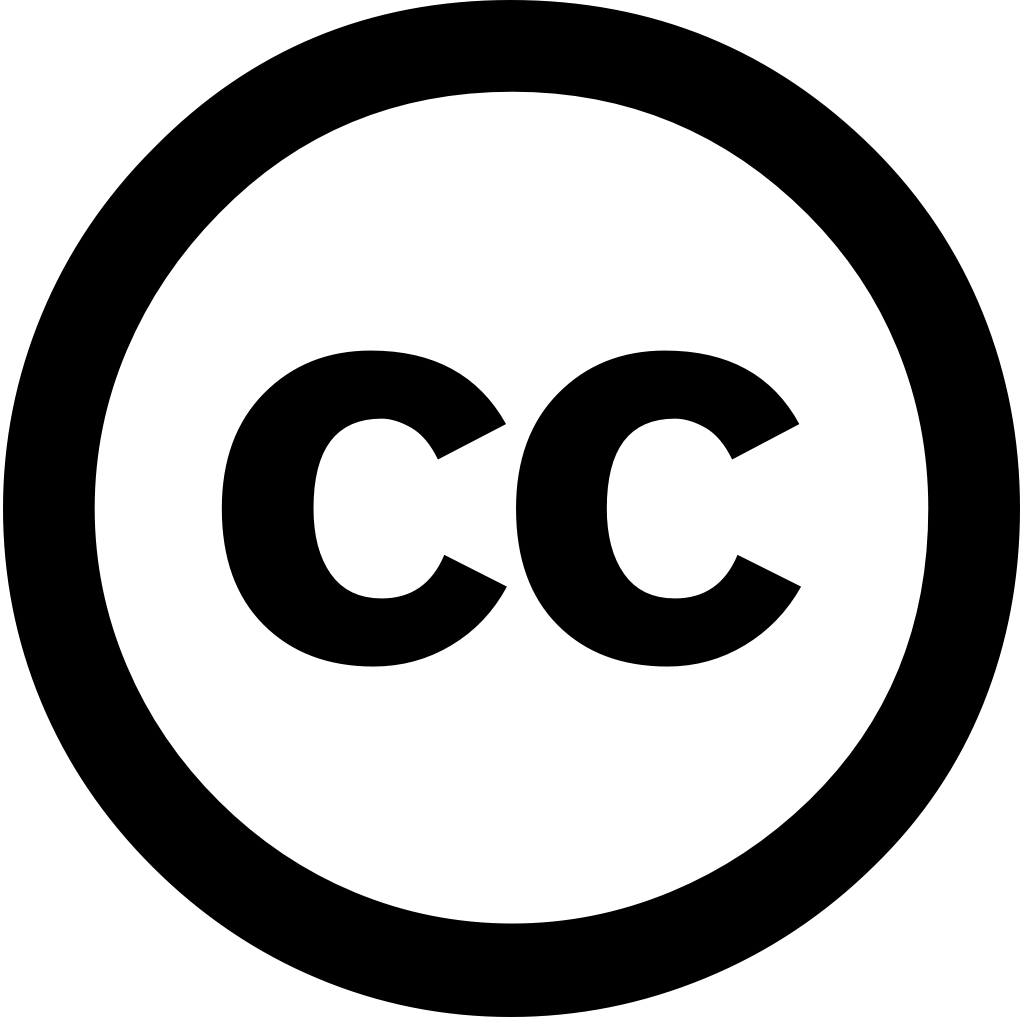
iMeta, Год журнала: 2025, Номер unknown
Опубликована: Апрель 25, 2025
Abstract Since its initial release in 2022, ggClusterNet has become a vital tool for microbiome research, enabling microbial co‐occurrence network analysis and visualization over 300 studies. To address emerging challenges, including multi‐factor experimental designs, multi‐treatment conditions, multi‐omics data, we present comprehensive upgrade with four key components: (1) A pipeline integrating computation (Pearson/Spearman/SparCC correlations), visualization, topological characterization of node properties, multi‐network comparison statistical testing, stability (robustness) analysis, module identification analysis; (2) Network mining functions multi‐factor, multi‐treatment, spatiotemporal‐scale Facet.Network() module.compare.m.ts() ; (3) Transkingdom construction using microbiota, multi‐omics, other relevant diverse layouts such as MatCorPlot2() cor_link3() (4) corBionetwork.st() algorithms tailored complex exploration, model_maptree2() , model_Gephi.3() cir.squ() . The updates 2 enable researchers to explore interactions, offering robust, efficient, user‐friendly, reproducible, visually versatile networks indicator correlation patterns. R package is open‐source available on GitHub ( https://github.com/taowenmicro/ggClusterNet ).
Язык: Английский