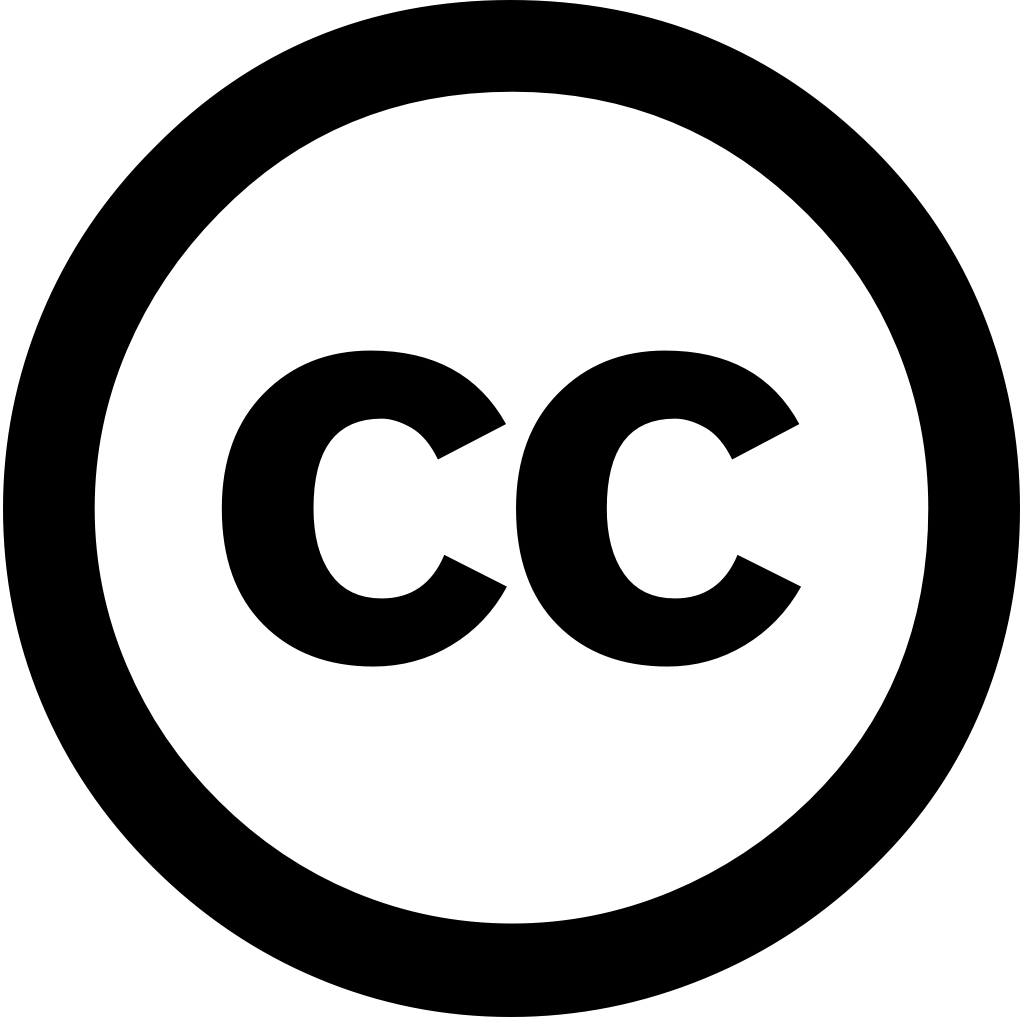
Materials Science and Engineering R Reports, Год журнала: 2020, Номер 144, С. 100595 - 100595
Опубликована: Дек. 26, 2020
Язык: Английский
Materials Science and Engineering R Reports, Год журнала: 2020, Номер 144, С. 100595 - 100595
Опубликована: Дек. 26, 2020
Язык: Английский
The Innovation, Год журнала: 2021, Номер 2(4), С. 100179 - 100179
Опубликована: Окт. 29, 2021
•"Can machines think?" The goal of artificial intelligence (AI) is to enable mimic human thoughts and behaviors, including learning, reasoning, predicting, so on.•"Can AI do fundamental research?" coupled with machine learning techniques impacting a wide range sciences, mathematics, medical science, physics, etc.•"How does accelerate New research applications are emerging rapidly the support by infrastructure, data storage, computing power, algorithms, frameworks. Artificial promising (ML) well known from computer science broadly affecting many aspects various fields technology, industry, even our day-to-day life. ML have been developed analyze high-throughput view obtaining useful insights, categorizing, making evidence-based decisions in novel ways, which will promote growth fuel sustainable booming AI. This paper undertakes comprehensive survey on development application different information materials geoscience, life chemistry. challenges that each discipline meets, potentials handle these challenges, discussed detail. Moreover, we shed light new trends entailing integration into scientific discipline. aim this provide broad guideline sciences potential infusion AI, help motivate researchers deeply understand state-of-the-art AI-based thereby continuous sciences.
Язык: Английский
Процитировано
943Nature, Год журнала: 2023, Номер 620(7972), С. 47 - 60
Опубликована: Авг. 2, 2023
Язык: Английский
Процитировано
708Antibiotics, Год журнала: 2020, Номер 9(1), С. 24 - 24
Опубликована: Янв. 13, 2020
More than 3000 antimicrobial peptides (AMPs) have been discovered, seven of which approved by the U.S. Food and Drug Administration (FDA). Now commercialized, these mostly utilized for topical medications, though some injected into body to treat severe bacterial infections. To understand translational potential AMPs, we analyzed FDA-approved drugs in FDA drug database. We examined their physicochemical properties, secondary structures, mechanisms action, compared them with AMP All AMPs were discovered Gram-positive soil bacteria, 98% known also come from natural sources (skin secretions frogs toxins different species). However, can undesirable properties as drugs, including instability toxicity. Thus, design construction effective require an understanding effects on human body. This review provides overview guide development that potentially be used drugs.
Язык: Английский
Процитировано
490ACS Catalysis, Год журнала: 2019, Номер 10(3), С. 2260 - 2297
Опубликована: Дек. 16, 2019
The discovery and development of catalysts catalytic processes are essential components to maintaining an ecological balance in the future. Recent revolutions made data science could have a great impact on traditional catalysis research both industry academia accelerate catalysts. Machine learning (ML), subfield science, can play central role this paradigm shift away from use approaches. In review, we present user's guide for ML that believe will be helpful scientists performing field summarize recent progress has been utilizing create homogeneous heterogeneous focus review is design, synthesis, characterization materials/compounds as well their applications catalyzed processes. technique not only enhances ways discover but also serves powerful tool establish deeper understanding relationships between properties activities, selectivities, stabilities. This knowledge facilitates establishment principles employed design enhance efficiencies. Despite such advantages ML, it noteworthly current ML-assisted real remains its infancy, mainly because complexity associated with fact time-dependent dynamic event. discuss how seamless integration experiment, theory, used catalyst future studies aimed at society's need produce energy, materials, chemicals. Moreover, limitations difficulties originating complex nature discussed order make community aware challenges addressed effective practical field.
Язык: Английский
Процитировано
454Chemical Science, Год журнала: 2020, Номер 11(12), С. 3316 - 3325
Опубликована: Янв. 1, 2020
We present an extension of our Molecular Transformer model combined with a hyper-graph exploration strategy for automatic retrosynthesis route planning without human intervention. The single-step retrosynthetic sets new state the art predicting reactants as well reagents, solvents and catalysts each step. introduce four metrics (coverage, class diversity, round-trip accuracy Jensen-Shannon divergence) to evaluate models, using forward prediction reaction classification always based on transformer architecture. hypergraph is constructed fly, nodes are filtered further expanded Bayesian-like probability. critically assessed end-to-end framework several examples from literature academic exams. Overall, frameworks have excellent performance few weaknesses related training data. use introduced opens up possibility optimize entire by focusing only.
Язык: Английский
Процитировано
368Accounts of Chemical Research, Год журнала: 2021, Номер 54(4), С. 849 - 860
Опубликована: Фев. 2, 2021
ConspectusThe ongoing revolution of the natural sciences by advent machine learning and artificial intelligence sparked significant interest in material science community recent years. The intrinsically high dimensionality space realizable materials makes traditional approaches ineffective for large-scale explorations. Modern data tools developed increasingly complicated problems are an attractive alternative. An imminent climate catastrophe calls a clean energy transformation overhauling current technologies within only several years possible action available. Tackling this crisis requires development new at unprecedented pace scale. For example, organic photovoltaics have potential to replace existing silicon-based large extent open up fields application. In years, light-emitting diodes emerged as state-of-the-art technology digital screens portable devices enabling applications with flexible displays. Reticular frameworks allow atom-precise synthesis nanomaterials promise revolutionize field realize multifunctional nanoparticles from gas storage, separation, electrochemical storage nanomedicine. decade, advances all these been facilitated comprehensive application simulation property prediction, optimization, chemical exploration enabled considerable computing power algorithmic efficiency.In Account, we review most contributions our group thriving science. We start summary important classes has involved in, focusing on small molecules electronic crystalline materials. Specifically, highlight data-driven employed speed discovery derive design strategies. Subsequently, focus lies methodologies employed, elaborating high-throughput virtual screening, inverse molecular design, Bayesian supervised learning. discuss general ideas, their working principles, use cases examples successful implementations efforts. Furthermore, elaborate pitfalls remaining challenges methods. Finally, provide brief outlook foresee increasing adaptation implementation scale campaigns.
Язык: Английский
Процитировано
323Chemical Reviews, Год журнала: 2021, Номер 121(16), С. 10218 - 10239
Опубликована: Июнь 7, 2021
Machine learning (ML) techniques applied to chemical reactions have a long history. The present contribution discusses applications ranging from small molecule reaction dynamics computational platforms for planning. ML-based can be particularly relevant problems involving both computation and experiments. For one, Bayesian inference is powerful approach develop models consistent with knowledge Second, methods also used handle that are formally intractable using conventional approaches, such as exhaustive characterization of state-to-state information in reactive collisions. Finally, the explicit simulation networks they occur combustion has become possible machine-learned neural network potentials. This review provides an overview questions been addressed machine techniques, outlook challenges this diverse stimulating field. It concluded ML chemistry practiced conceived today potential transform way which field approaches reactions, research academic teaching.
Язык: Английский
Процитировано
310Chemical Reviews, Год журнала: 2020, Номер 120(15), С. 7219 - 7347
Опубликована: Июль 15, 2020
The ever increasing industrial production of commodity and specialty chemicals inexorably depletes the finite primary fossil resources available on Earth. forecast population growth over next 3 decades is a very strong incentive for identification alternative other than petro-based ones. In contrast with resources, renewable biomass virtually inexhaustible reservoir chemical building blocks. Shifting current paradigm from almost exclusively to bio-based raw materials requires more vibrant political messages; it profound revision concepts technologies which processes rely. Only small fraction molecules extracted bears significant commercial potentials be considered as ubiquitous platforms upon new, industry can thrive. Owing its inherent assets in terms unique process experience, scalability, reduced environmental footprint, flow chemistry arguably has major role play this context. This review covers selection C2 C6 existing markets including polyols (ethylene glycol, 1,2-propanediol, 1,3-propanediol, glycerol, 1,4-butanediol, xylitol, sorbitol), furanoids (furfural 5-hydroxymethylfurfural) carboxylic acids (lactic acid, succinic fumaric malic itaconic levulinic acid). aim illustrate various aspects upgrading platform toward or using new that fall under umbrella continuous technology could change future perspectives biorefineries.
Язык: Английский
Процитировано
307Journal of Chemical Information and Modeling, Год журнала: 2020, Номер 60(12), С. 5714 - 5723
Опубликована: Апрель 6, 2020
The discovery of functional molecules is an expensive and time-consuming process, exemplified by the rising costs small molecule therapeutic discovery. One class techniques growing interest for early stage drug de novo molecular generation optimization, catalyzed development new deep learning approaches. These can suggest novel structures intended to maximize a multiobjective function, e.g., suitability as against particular target, without relying on brute-force exploration chemical space. However, utility these approaches stymied ignorance synthesizability. To highlight severity this issue, we use data-driven computer-aided synthesis planning program quantify how often proposed state-of-the-art generative models cannot be readily synthesized. Our analysis demonstrates that there are several tasks which generate unrealistic despite performing well popular quantitative benchmarks. Synthetic complexity heuristics successfully bias toward synthetically tractable space, although doing so necessarily detracts from primary objective. This suggests improve in real workflows, algorithm warranted.
Язык: Английский
Процитировано
294Nature, Год журнала: 2023, Номер 624(7990), С. 86 - 91
Опубликована: Ноя. 29, 2023
To close the gap between rates of computational screening and experimental realization novel materials1,2, we introduce A-Lab, an autonomous laboratory for solid-state synthesis inorganic powders. This platform uses computations, historical data from literature, machine learning (ML) active to plan interpret outcomes experiments performed using robotics. Over 17 days continuous operation, A-Lab realized 41 compounds a set 58 targets including variety oxides phosphates that were identified large-scale ab initio phase-stability Materials Project Google DeepMind. Synthesis recipes proposed by natural-language models trained on literature optimized active-learning approach grounded in thermodynamics. Analysis failed syntheses provides direct actionable suggestions improve current techniques materials design. The high success rate demonstrates effectiveness artificial-intelligence-driven platforms discovery motivates further integration knowledge
Язык: Английский
Процитировано
289