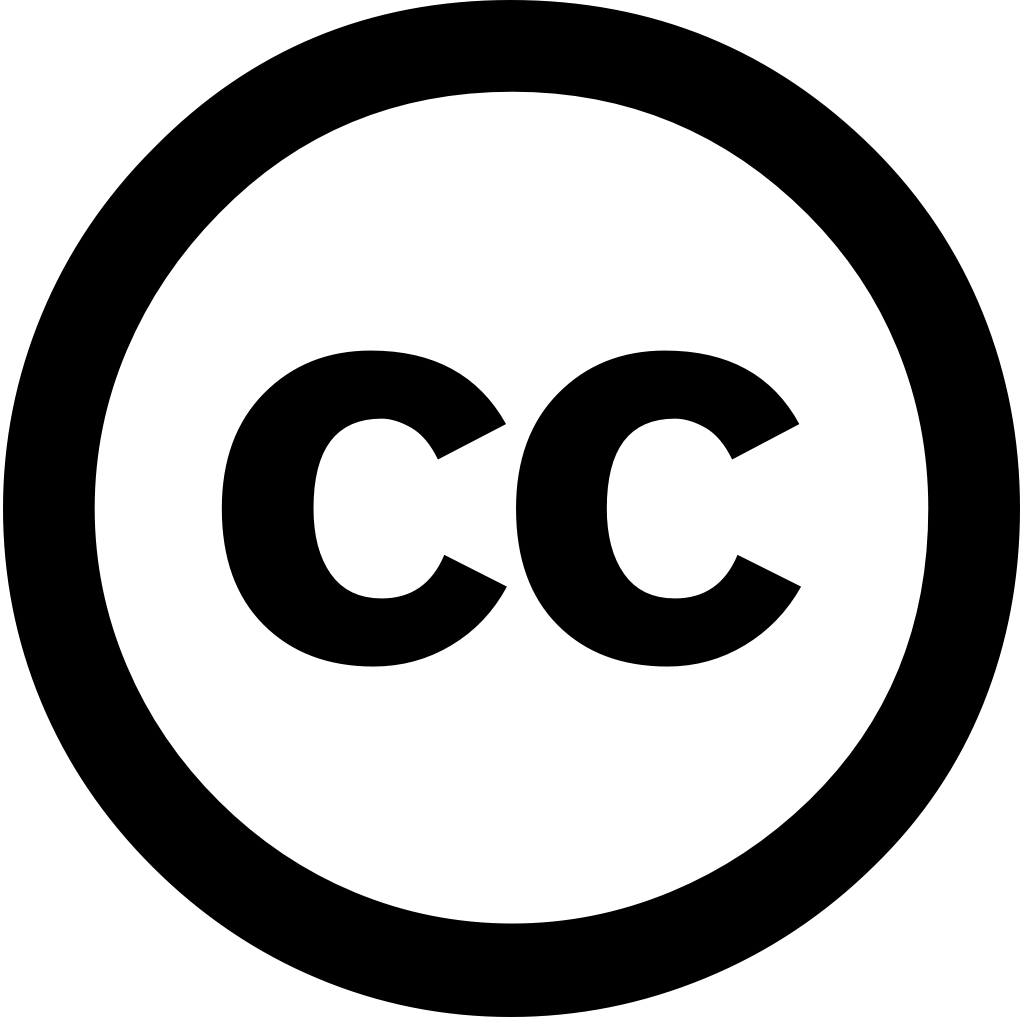
Research Square (Research Square), Год журнала: 2024, Номер unknown
Опубликована: Ноя. 27, 2024
Язык: Английский
Research Square (Research Square), Год журнала: 2024, Номер unknown
Опубликована: Ноя. 27, 2024
Язык: Английский
Frontiers in Medicine, Год журнала: 2025, Номер 11
Опубликована: Янв. 10, 2025
Generative artificial intelligence (GenAI) is rapidly transforming various sectors, including healthcare and education. This paper explores the potential opportunities risks of GenAI in graduate medical education (GME). We review existing literature provide commentary on how could impact GME, five key areas opportunity: electronic health record (EHR) workload reduction, clinical simulation, individualized education, research analytics support, decision support. then discuss significant risks, inaccuracy overreliance AI-generated content, challenges to authenticity academic integrity, biases AI outputs, privacy concerns. As technology matures, it will likely come have an important role future but its integration should be guided by a thorough understanding both benefits limitations.
Язык: Английский
Процитировано
2Deleted Journal, Год журнала: 2025, Номер unknown
Опубликована: Апрель 2, 2025
Imaging disciplines, such as ophthalmology, offer a wide range of opportunities for the beneficial use artificial intelligence (AI). The analysis images and data by trained algorithms has potential to facilitate making diagnosis patient care not just in ophthalmology. If AI brings about advances clinical practice that benefit patients, this is ethically be welcomed; however, respect self-determination patients security must guaranteed. Traceability explainability would strengthen trust automated decision-making enable ultimate medical responsibility. It should noted are only good unbiased used train them. likely lead loss skills on part doctors (deskilling), counteracted, example through improved training. Accompanying ethics research necessary identify those aspects require regulation. In principle, taken ensure serves people adapts their needs, other way round.
Язык: Английский
Процитировано
1Frontiers in Cell and Developmental Biology, Год журнала: 2025, Номер 12
Опубликована: Янв. 28, 2025
Purpose Using deep learning model to observe the blinking characteristics and evaluate changes their correlation with tear film in children long-term use of orthokeratology (ortho-K). Methods 31 (58 eyes) who had used ortho-K for more than 1 year age gender-matched controls were selected follow-up our ophthalmology clinic from 2021/09 2023/10 this retrospective case-control study. Both groups underwent comprehensive ophthalmological examinations, including Ocular Surface Disease Index (OSDI) scoring, Keratograph 5M, LipiView. A system based on U-Net Swim-Transformer was proposed observation characteristics. The frequency incomplete blinks (IB), complete (CB) rate (IBR) within 20 s, as well duration closing, closed, opening phases blink wave calculated by system. Relative IPH% defined ratio mean s maximum value indicate extent blinking. Furthermore, accuracy, precision, sensitivity, specificity, F1 score overall U-Net-Swin-Transformer model, its consistency built-in algorithm evaluated well. Independent t-test Mann-Whitney test analyze patterns between wearer group control group. Spearman’s rank relationship stability. Results Our demonstrated high performance (accuracy = 98.13%, precision 96.46%, sensitivity 98.10%, specificity 0.9727) patterns. OSDI scores, conjunctival redness, lipid layer thickness (LLT), meniscus height did not change significantly two groups. Notably, exhibited shorter first (11.75 ± 7.42 vs. 14.87 7.93 p 0.030) average non-invasive break-up times (NIBUT) (13.67 7.0 16.60 7.24 0.029) compared They a higher IB (4.26 2.98 2.36 2.55, < 0.001), IBR (0.81 0.28 0.46 0.39, relative (0.3229 0.1539 0.2233 0.1960, 0.004) prolonged eye-closing phase (0.18 0.08 0.15 0.07 0.032) (0.35 0.12 0.14 0.015) controls. In addition, analysis revealed negative NIBUT (for first-NIBUT, r −0.292, 0.004; avg-NIBUT, −0.3512, 0.001) ortho-K. Conclusion U-net achieved optimal Children presented an increase eye closing phase. increased associated decreased stability, indicating importance monitoring children’s status clinical follow-up.
Язык: Английский
Процитировано
0Progress in Retinal and Eye Research, Год журнала: 2025, Номер unknown, С. 101353 - 101353
Опубликована: Март 1, 2025
Язык: Английский
Процитировано
0British Journal of Ophthalmology, Год журнала: 2024, Номер 108(10), С. 1333 - 1334
Опубликована: Сен. 20, 2024
Язык: Английский
Процитировано
0IP International Journal of Ocular Oncology and Oculoplasty, Год журнала: 2024, Номер 10(3), С. 135 - 139
Опубликована: Ноя. 2, 2024
To evaluate the efficacy of AI chatbots (OpenAI ChatGPT GPT-3.5, Google Bard, and WebMD) compared to human ophthalmology trainees in triage referrals for common ophthalmic conditions. A single-center study was conducted at MKCG Medical College, Berhampur, Odisha, involving six trainees. The performance assessed based on diagnostic accuracy categorization. Key indicators included top three suggested diagnoses concordance recommendations investigations referrals. Physician respondents identified correct diagnosis among suggestions 95% cases. Bard achieved 90% accuracy, 85%, WebMD 20%. High observed between physician demonstrate promising potential supporting referral decisions While expertise remains crucial, tools can augment improve efficiency, enhance patient care. Future research should focus refining algorithms, integrating clinical data, exploring real-world implementation strategies.
Язык: Английский
Процитировано
0Research Square (Research Square), Год журнала: 2024, Номер unknown
Опубликована: Ноя. 27, 2024
Язык: Английский
Процитировано
0