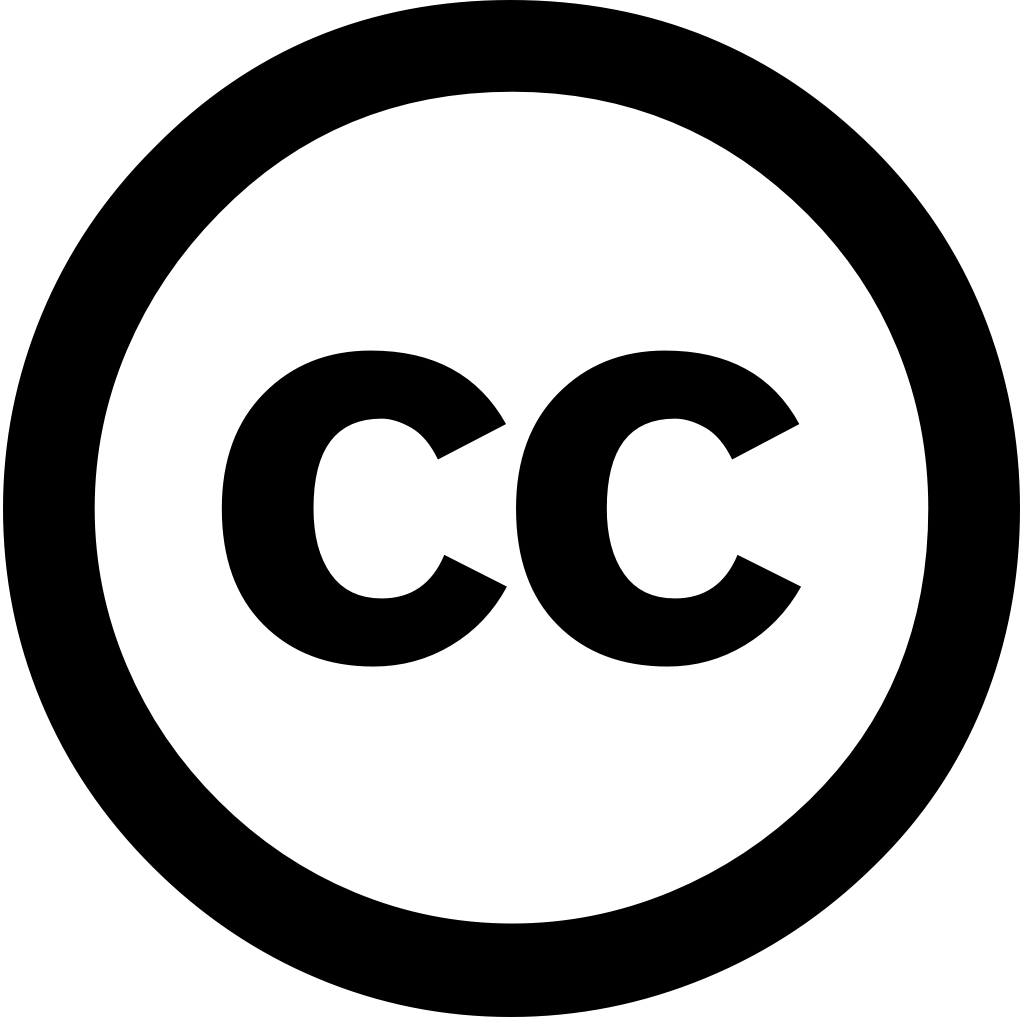
Frontiers in Medicine, Год журнала: 2025, Номер 12
Опубликована: Апрель 11, 2025
Introduction Reintubation is an adverse postoperative complication in patients with Type A aortic dissection (AAD) that correlates to poor outcomes. This study aims employ machine learning algorithms establish a practical platform for the prediction of reintubation. Methods total 861 diagnosed AAD and undergoing surgical procedures, 688 as training testing cohort from single center, 173 validation four centers were enrolled. The least absolute shrinkage selection operator (LASSO) was used screening risk variables associated reintubation subsequent model construction. Subsequently, seven machine-learning models built. best discrimination calibration performance predict Finally, SHapley Additive exPlanation (SHAP) employed explain model. Results performed 107 (12.43%). LASSO analysis identified re-admission intensive care unit (ICU), continuous renal replacement therapy, length stay ICU, duration invasive mechanical ventilation significant factors XGBoost selected final due its better than other models, AUC, sensitivity, specificity 0.969, 0.8889, 0.8611 cohort. SHAP values demonstrated effects individual features on overall web calculator developed based clinical use. Conclusion We have validated high-performing AAD. It can provide valuable guidance clinicians predicting developing timely preventative measures.
Язык: Английский