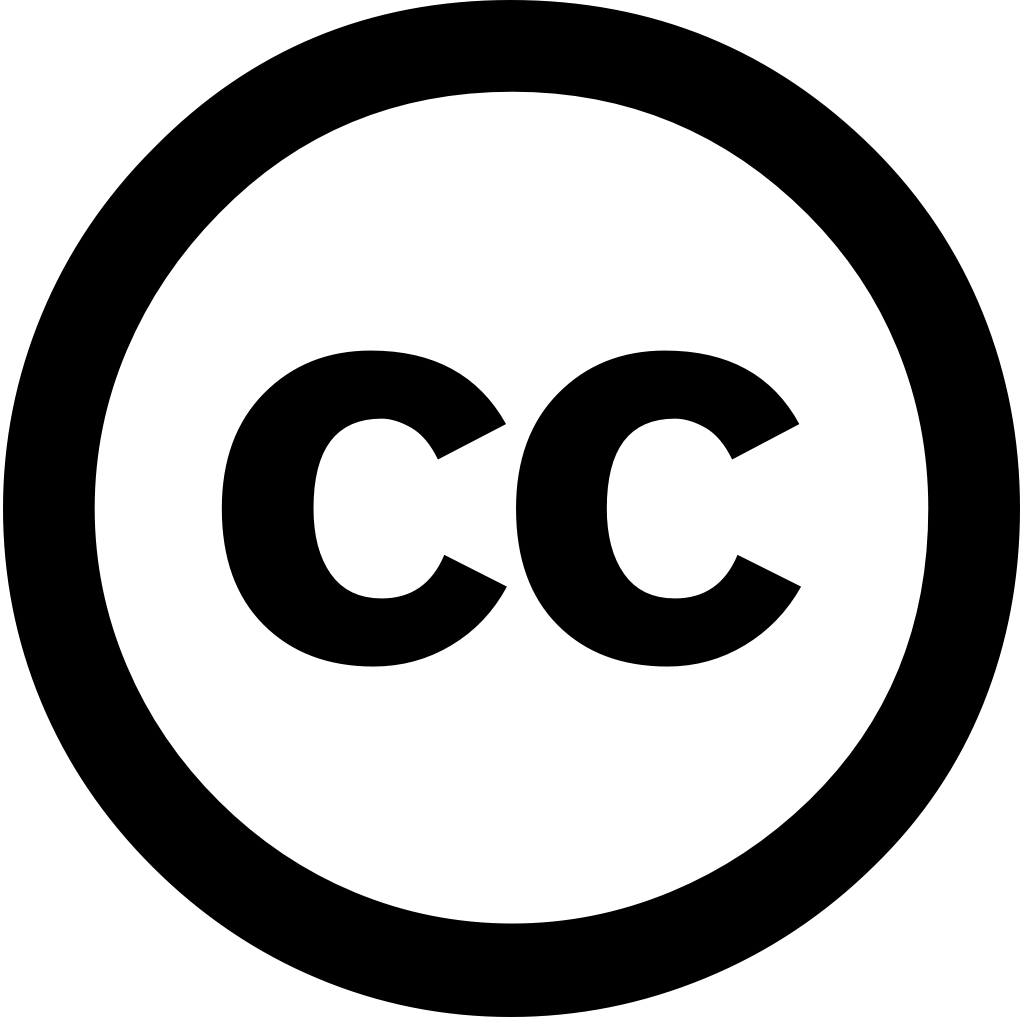
BMC Anesthesiology, Год журнала: 2025, Номер 25(1)
Опубликована: Апрель 10, 2025
Effective management of postoperative pain remains a significant challenge in obstetric care due to the variability perception and response influenced by physical, medical, psychosocial factors. Current standardized protocols often fail accommodate this variability, necessitating more tailored approaches. This study aims improve following cesarean sections developing personalized using machine learning (ML) models. The analyzed efficacy eight ML models, including XGBoost, Random Forest, Neural Networks, data from two distinct hospital cohorts. Performance metrics such as Root Mean Squared Error (RMSE) Coefficient Determination (R²) were evaluated through internal external validations. SHAP value analysis was used identify key predictors influencing outcomes. XGBoost model demonstrated superior performance, achieving lowest RMSE highest R². Key factors impacting included esketamine use, anesthesia method, anesthetic drug type, with significantly delaying first activation patient-controlled intravenous analgesia (PCIA). highlights potential refine strategies care, suggesting that approaches, particularly incorporating specific methods, could enhance patient Not applicable.
Язык: Английский