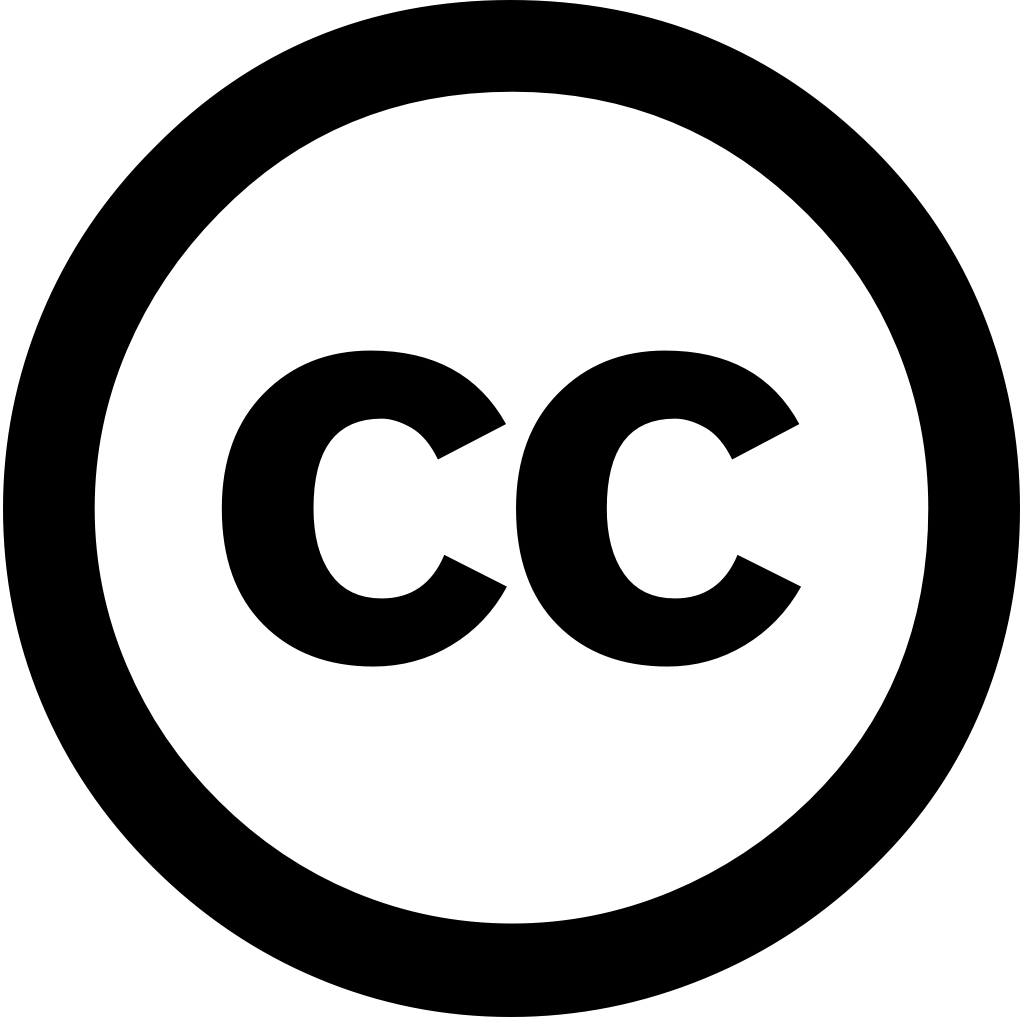
Big Data and Cognitive Computing, Год журнала: 2024, Номер 8(12), С. 192 - 192
Опубликована: Дек. 16, 2024
Background/Objectives: The COVID-19 pandemic, caused by Severe Acute Respiratory Syndrome Coronavirus 2 (SARS-CoV-2), led to significant global health challenges, including the urgent need for accurate symptom severity prediction aimed at optimizing treatment. While machine learning (ML) and deep (DL) models have shown promise in predicting using imaging clinical data, there is limited research utilizing comprehensive tabular datasets. This study aims address this gap leveraging a detailed dataset develop robust categorizing severity, thereby enhancing decision making. Methods: A unique was created questionnaire responses from 5654 individuals, demographic information, comorbidities, travel history, medical data. Both unsupervised supervised ML techniques were employed, k-means clustering categorize into mild, moderate, severe clusters. In addition, classification models, namely, Support Vector Machine (SVM), Adaptive Boosting (AdaBoost), eXtreme Gradient (XGBoost), random forest, neural network (DNN) used predict levels. Feature importance analyzed forest model its robustness with high-dimensional data ability capture complex non-linear relationships, statistical significance evaluated through ANOVA Chi-square tests. Results: Our showed that fatigue, joint pain, headache most important features severity. SVM, AdaBoost, achieved an accuracy of 94%, while XGBoost 96%. DNN performance handling patterns 98% accuracy. terms precision recall metrics, both demonstrated performance, particularly moderate class. recorded 97% recall, 99% recall. approach improved reducing noise dimensionality. Statistical tests confirmed additional like Body Mass Index (BMI), age, dominant variant type. Conclusions: Integrating advanced offers promising classification. method provides reliable tool healthcare professionals optimize patient care resource management, managing potential future pandemics. Future work should focus on incorporating further enhance applicability.
Язык: Английский