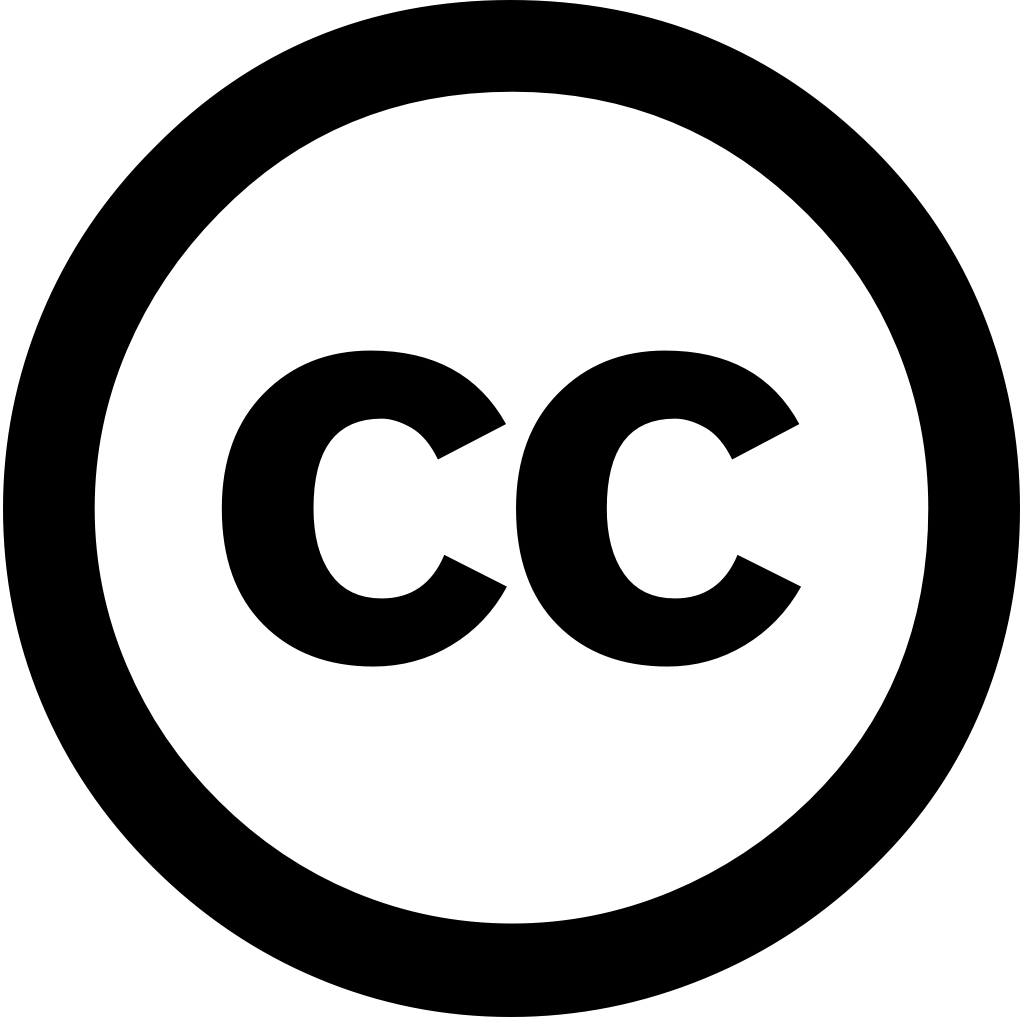
Research Square (Research Square), Год журнала: 2025, Номер unknown
Опубликована: Март 25, 2025
Язык: Английский
Research Square (Research Square), Год журнала: 2025, Номер unknown
Опубликована: Март 25, 2025
Язык: Английский
BMC Psychiatry, Год журнала: 2025, Номер 25(1)
Опубликована: Янв. 2, 2025
The high comorbidity and symptom overlap of generalized anxiety disorder (GAD), posttraumatic stress (PTSD), social (SAD), has led to the study their shared disorder-specific neural substrates. However, morphometric similarity network (MSN) differences among these disorders remain unknown. MSN derived from T1-weighted images in patients GAD, PTSD, SAD, health controls (HC) using a Siemens 3T magnetic resonance imaging system. Covariance analysis post hoc tests were used investigate group differences. In addition, relationship between clinical characteristics was analyzed. Increased (MS) left bankssts (BA22, superior temporal cortex, STC) right precentral gyrus, decreased MS gyrus cuneus_part1/part2, rostral middle frontal cortex (rMFC) STC common GAD PTSD relative HC SAD. Compared other three groups, SAD exhibited alterations increased rMFC STC, cuneus inferior parietal cortex. Additionally, regional found compared A mild positive correlation value Hamilton Anxiety Rating Scale scores (uncorrected p = 0.041) PTSD. Our provides first evidence for distinct brain abnormalities underlying pathophysiology which may aid differential diagnosis determining potential intervention targets.
Язык: Английский
Процитировано
0Research Square (Research Square), Год журнала: 2025, Номер unknown
Опубликована: Март 25, 2025
Язык: Английский
Процитировано
0