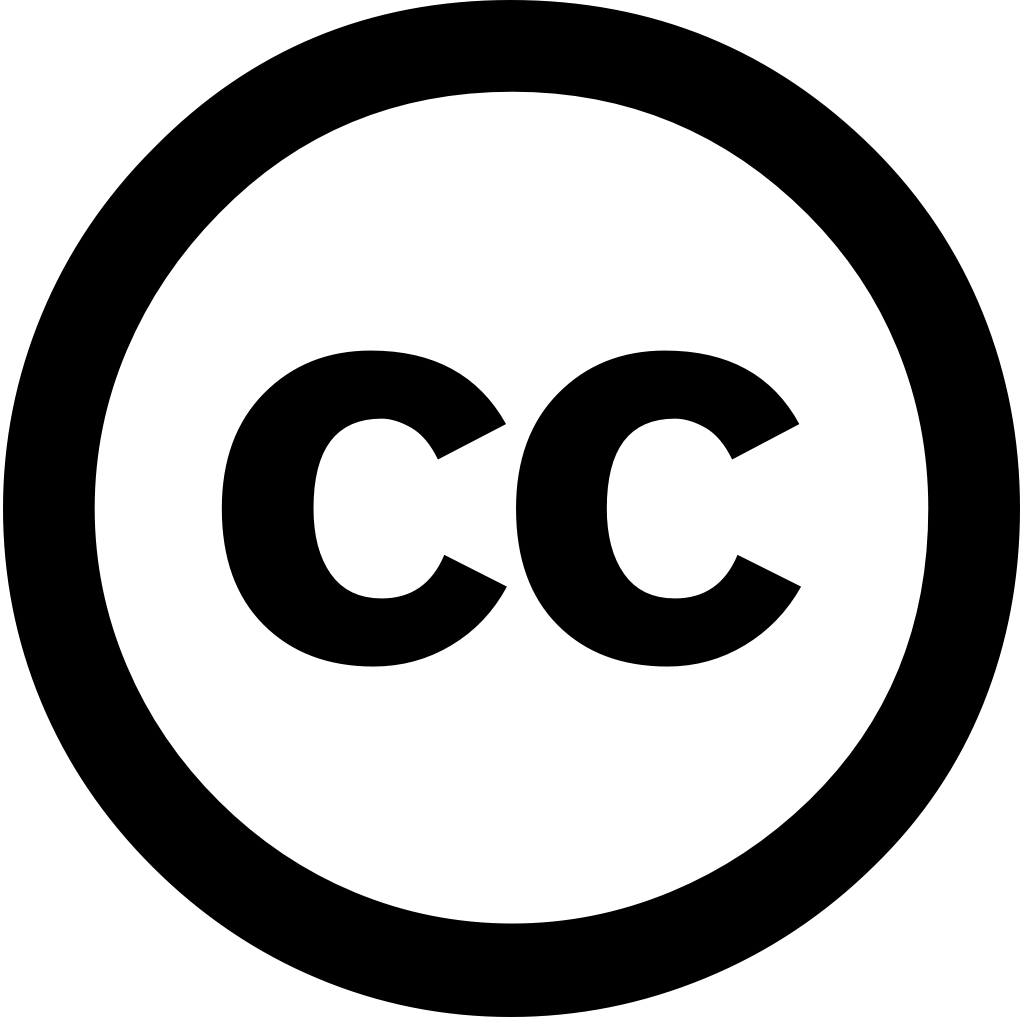
Biology Direct, Год журнала: 2025, Номер 20(1)
Опубликована: Апрель 21, 2025
Mitotic catastrophe is well-known as a major pathway of endogenous tumor death, but the prognostic significance its heterogeneity regarding bladder cancer (BLCA) remains unclear. Our study focused on digging deeper into TCGA and GEO databases. Through differential expression analysis well Weighted Gene Co-expression Network Analysis (WGCNA), we identified dysregulated mitotic catastrophe-associated genes, followed by univariate cox regression ten machine learning algorithms to construct robust models. Based stratification, revealed intergroup differences enrichment analysis, immune infiltration assessment, genomic variant analysis. Subsequently multivariate survshap(t) model screened core gene it Mendelian randomization. Integration qRT-PCR, immunohistochemistry, single-cell explored landscape. In addition, ceRNA axis containing upstream non-coding RNAs after detailed activation, immunoregulation, methylation functions genes. Finally, performed drug screening molecular docking experiments based in DSigDB database. efforts culminated establishment an accurate 16 genes Coxboost Random Survival Forest (RSF) algorithm. Detailed from multiple perspectives strong link between scores many key indicators: landscape, personalized treatment. ANLN was model, that portends poor prognosis, further corroborated randomization Interestingly, significantly upregulated cells specifically clustered epithelial provided pathways mediate cell division. regulated patterns also inseparable overall levels. Further potential regulation MIR4435-2HG, hsa-miR-15a-5p, highlighted range therapeutic agents including Phytoestrogens. The developed powerful predictive tool for BLCA prognosis impact dimensions, which then guided clinical decision-making. Furthermore, target.
Язык: Английский