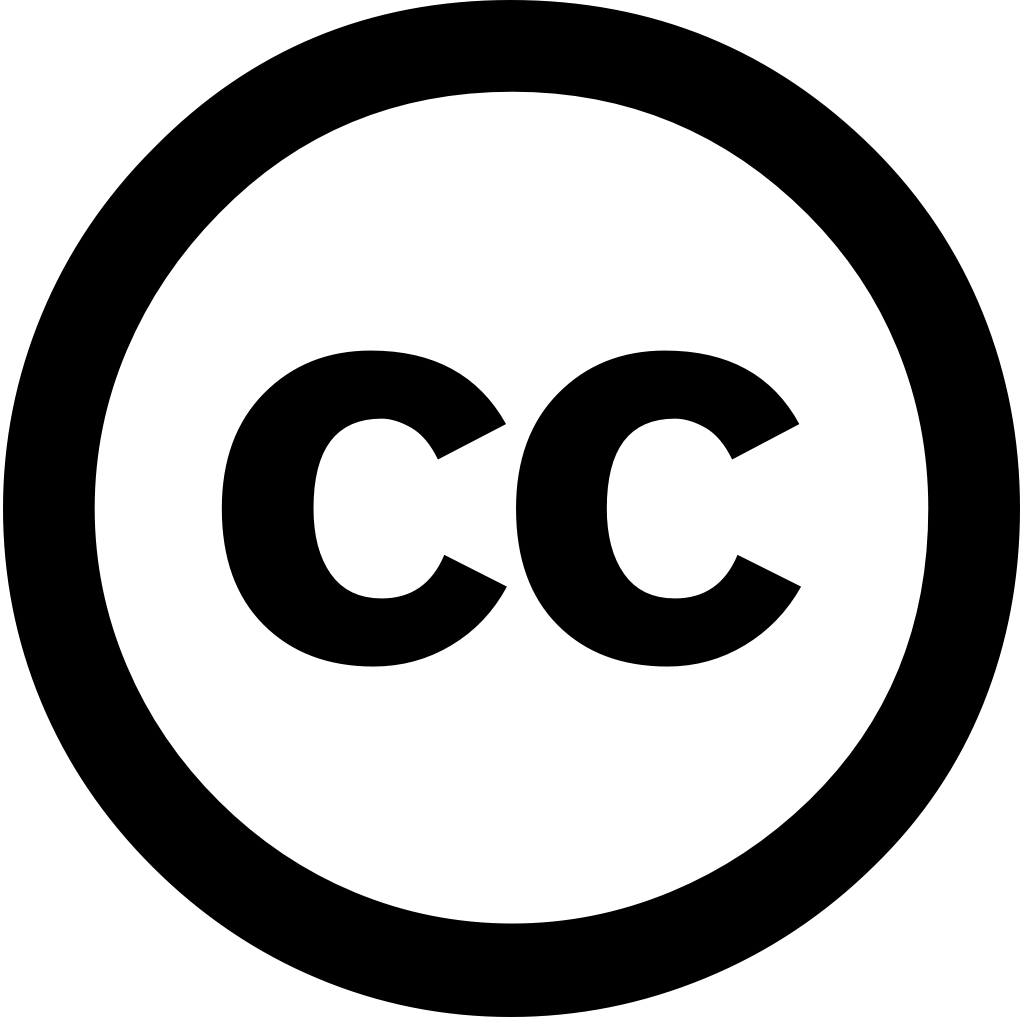
Research Square (Research Square), Год журнала: 2024, Номер unknown
Опубликована: Июнь 11, 2024
Язык: Английский
Research Square (Research Square), Год журнала: 2024, Номер unknown
Опубликована: Июнь 11, 2024
Язык: Английский
Опубликована: Май 25, 2024
The application of knowledge distillation to reduce hallucination in large language models represents a novel and significant advancement enhancing the reliability accuracy AI-generated content. research presented demonstrates efficacy transferring from high-capacity teacher model more compact student model, leading substantial improvements exact match notable reductions rates. methodology involved use temperature scaling, intermediate layer matching, comprehensive evaluation using MMLU benchmark, which assessed model’s performance across diverse set tasks. Experimental results indicated that distilled outperformed baseline generating accurate contextually appropriate responses while maintaining computational efficiency. findings underscore potential as scalable solution for improving robustness models, making them applicable real-world scenarios demand high factual accuracy. Future directions include exploring multilingual multi-modal distillation, integrating reinforcement learning, developing refined metrics further enhance performance.
Язык: Английский
Процитировано
20Research Square (Research Square), Год журнала: 2024, Номер unknown
Опубликована: Июнь 6, 2024
Язык: Английский
Процитировано
16Research Square (Research Square), Год журнала: 2024, Номер unknown
Опубликована: Июнь 4, 2024
Язык: Английский
Процитировано
15Опубликована: Июнь 6, 2024
The increasing use of AI-generated content has highlighted the critical issue hallucinations, where models produce factually incorrect or misleading outputs. Addressing this challenge, a novel approach dynamically supplements federated search engine results in real-time to significantly reduce hallucinations and enhance response accuracy. methodology involves integrating data from multiple engines into responses generated by Mistral Large model, thereby providing more accurate contextually appropriate output. Comprehensive evaluation using Microsoft PromptBench dataset demonstrates substantial improvements accuracy, relevance, reduction hallucinations. Quantitative performance metrics, statistical analysis, detailed case studies confirm effectiveness dynamic supplementation approach. findings suggest significant implications for developing reliable AI applications across various domains, emphasizing potential hybrid systems that combine strengths large language information retrieval. Future research directions include refining triggering mechanisms, expanding sources, optimizing process further scalability.
Язык: Английский
Процитировано
12Research Square (Research Square), Год журнала: 2024, Номер unknown
Опубликована: Июнь 7, 2024
Язык: Английский
Процитировано
9Research Square (Research Square), Год журнала: 2024, Номер unknown
Опубликована: Апрель 5, 2024
Abstract This study explores the enhancement of contextual understanding and factual accuracy in Language Learning Models (LLMs), specifically Mistral LLM, through integration external knowledge bases. We developed a novel methodology for dynamically incorporating real-time information from diverse sources, aiming to address inherent limitations LLMs rooted their training datasets. Our experiments demonstrated significant improvements accuracy, precision, recall, F1 score, alongside qualitative enhancements response relevance accuracy. The research also tackled computational challenges integrating knowledge, ensuring model's efficiency practical applicability. work not only highlights potential bases augment capabilities but sets stage future advancements creating more intelligent, adaptable, contextually aware AI systems. findings contribute broader field NLP by offering insights into overcoming traditional LLMs, presenting step toward developing systems with enhanced real-world applicability accessibility.
Язык: Английский
Процитировано
8Опубликована: Май 10, 2024
Evaluating the improvisational capabilities of large language models (LLMs) like ChatGPT-4, Mistral, and Anthropic Claude across textual, visual, psychological domains provides critical insights into their functionality potential applications. The research demonstrates significant variances in ability these to generate creative, contextually appropriate responses, visually coherent images from textual descriptions, emotionally nuanced interactions. ChatGPT-4 excelled improvisation, showcasing its capacity produce linguistically rich innovative content that pushes boundaries traditional text-based AI Mistral distinguished itself generation visual content, effectively translating abstract prompts detailed relevant images, indicating utility creative design fields. performed exceptionally well adaptability, interpreting responding emotional cues with a high degree empathy accuracy, suitable for customer service therapeutic findings underscore diverse LLMs, highlighting transform industries require understanding complex content. Future should focus on enhancing reliability varied scenarios, improving ethical deployment, exploring hybrid approaches leverage unique strengths.
Язык: Английский
Процитировано
6Опубликована: Июнь 11, 2024
Tokenization methods have long been a critical component in the performance of language models, yet traditional static approaches often fall short capturing dynamic nature language. The novel concept implementing tokenization dictionary within Llama model presents significant advancement, offering real-time adaptability response to evolving linguistic patterns. adaptive algorithm continuously updates token set based on frequency and context, thereby enhancing model's ability generate coherent contextually relevant outputs. Comprehensive evaluation across multiple benchmark datasets reveals substantial improvements metrics such as perplexity, F1 Score, BLEU ROUGE underscoring efficacy tokenization. implications these findings extend various domains, including healthcare, legal analysis, education, customer service, demonstrating broad applicability transformative potential tokenized dictionaries. This research not only advances understanding processes but also provides robust framework for efficiency accuracy large models real-world applications.
Язык: Английский
Процитировано
6Опубликована: Июнь 10, 2024
Artificial intelligence (AI) systems, particularly those capable of natural language processing, are increasingly becoming integral to diverse aspects human life and interaction. Understanding the cultural biases embedded within AI, especially in how it aligns with specific values, is crucial for ensuring its effective equitable deployment. This research examines alignment AI-generated responses mainstream Chinese such as Confucian harmony, Daoist balance, collectivism, respect authority, family-centric principles. By analyzing both English, study highlights discrepancies inherent AI offering valuable insights into their implications development. The findings reveal that while demonstrates general significant variations exist between contexts, emphasizing importance linguistic specificity interactions. Quantitative metrics thematic analyses demonstrate necessity culturally aware contributing broader discourse on ethical development providing guidance creating more inclusive adaptable systems.
Язык: Английский
Процитировано
4Research Square (Research Square), Год журнала: 2024, Номер unknown
Опубликована: Июнь 11, 2024
Язык: Английский
Процитировано
3