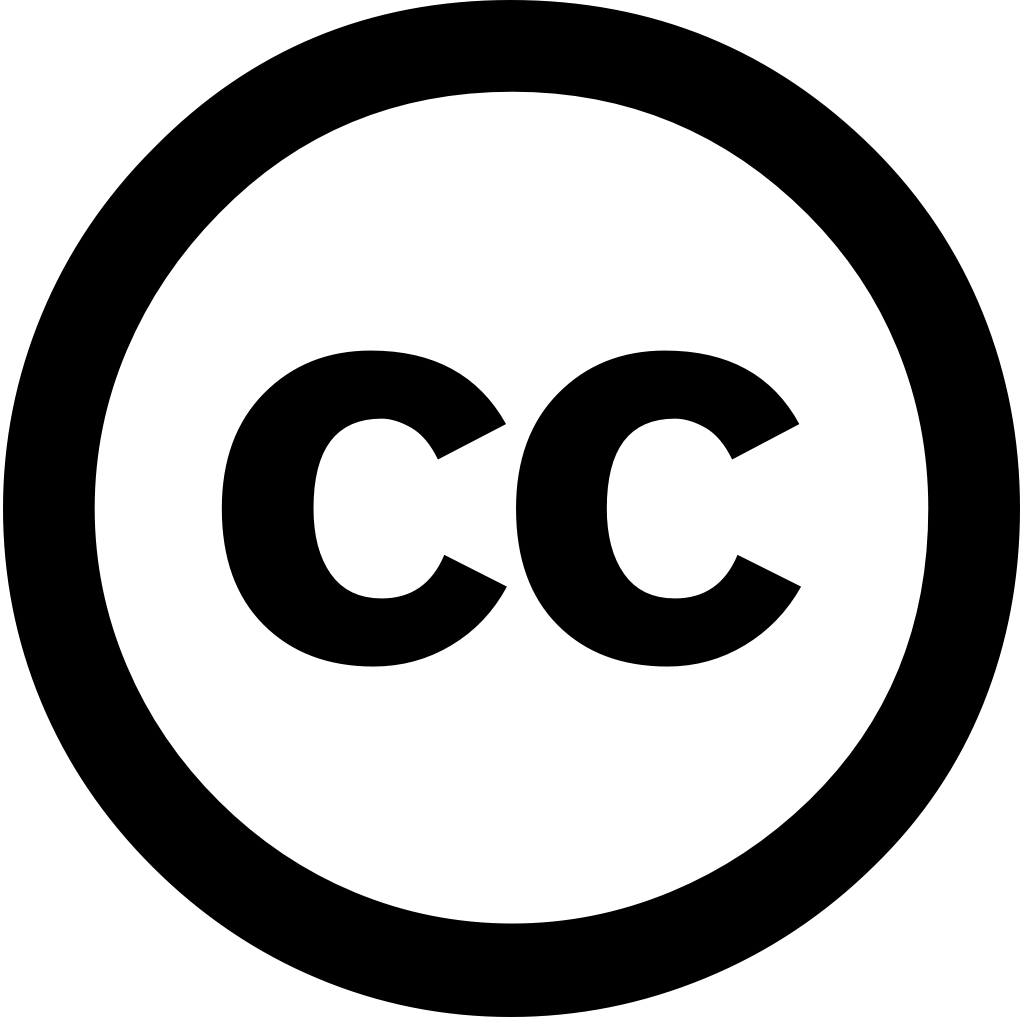
Applied Sciences, Год журнала: 2024, Номер 14(22), С. 10286 - 10286
Опубликована: Ноя. 8, 2024
Human Activity Recognition (HAR) is an essential area of research in Artificial Intelligence and Machine Learning, with numerous applications healthcare, sports science, smart environments. While several advancements the field, such as attention-based models Graph Neural Networks, have made great strides, this work focuses on data augmentation methods that tackle issues like scarcity task variability HAR. In work, we investigate expand use mixup cutout to sensor-based skeleton-based HAR datasets. These were first widely used Computer Vision Natural Language Processing. We both techniques, customized for time-series skeletal data, improve robustness performance by diversifying overcoming drawbacks having limited training data. Specifically, customize datasets goal improving model accuracy without adding more Our results show using techniques improves generalization activity recognition human This showcases potential transformers Networks offering a novel method enhancing time series tasks.
Язык: Английский