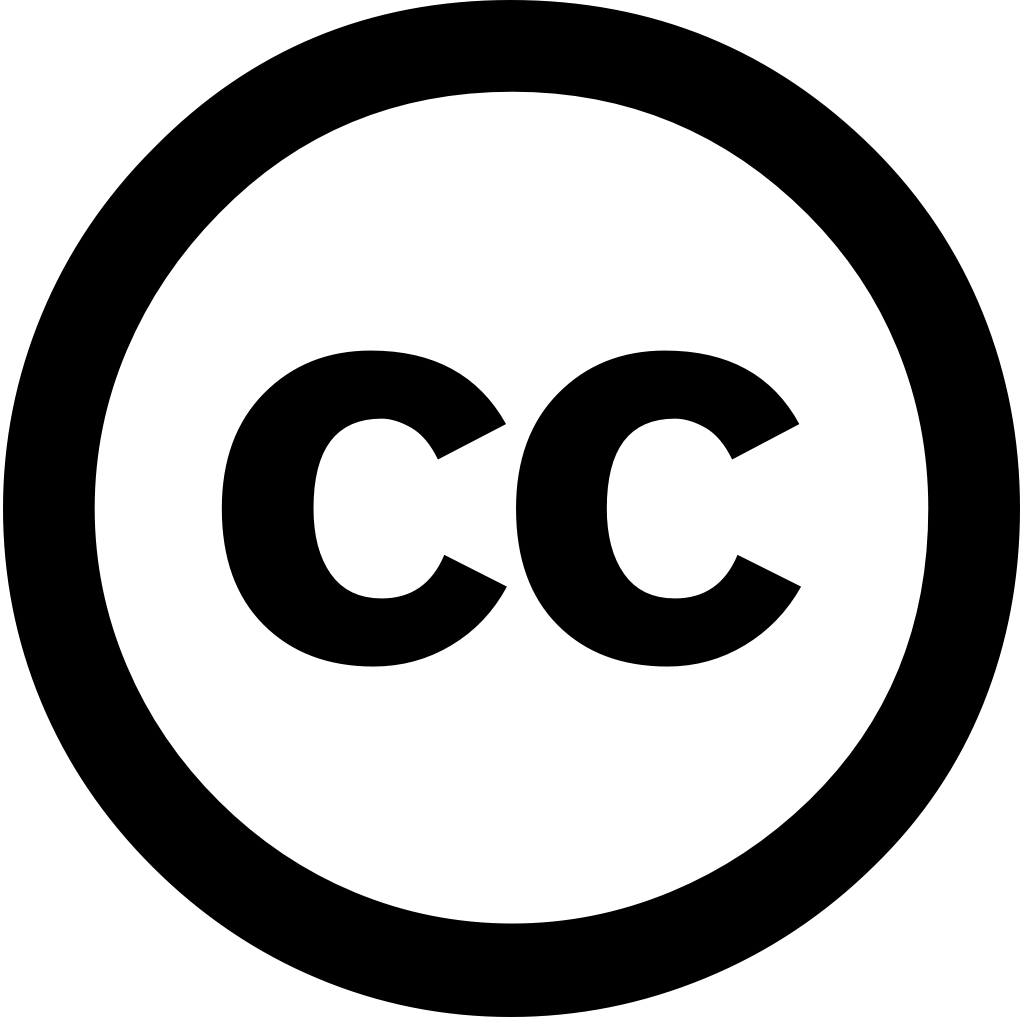
Applied Sciences, Год журнала: 2024, Номер 14(24), С. 11510 - 11510
Опубликована: Дек. 10, 2024
The increasing adoption of renewable energy sources and the emergence distributed generation have significantly transformed traditional landscape, leading to rise local markets. These markets facilitate decentralized trading among different market participants at community level, fostering greater autonomy sustainability. As gain momentum, application artificial intelligence techniques, particularly reinforcement learning, has gained substantial interest in optimizing strategies by interacting with environment maximizing rewards addressing decision complexities learning. This paper comprehensively reviews projects initiated global level machine learning approaches solution for State-of-the-art algorithms are classified into model-free model-based methods. classification examines various transactions considering agent type, methods, policy, state space, action selection state, action, reward function outputs. findings this work will serve as a valuable resource researchers, stakeholders, policymakers accelerate more efficient, sustainable, resilient future.
Язык: Английский