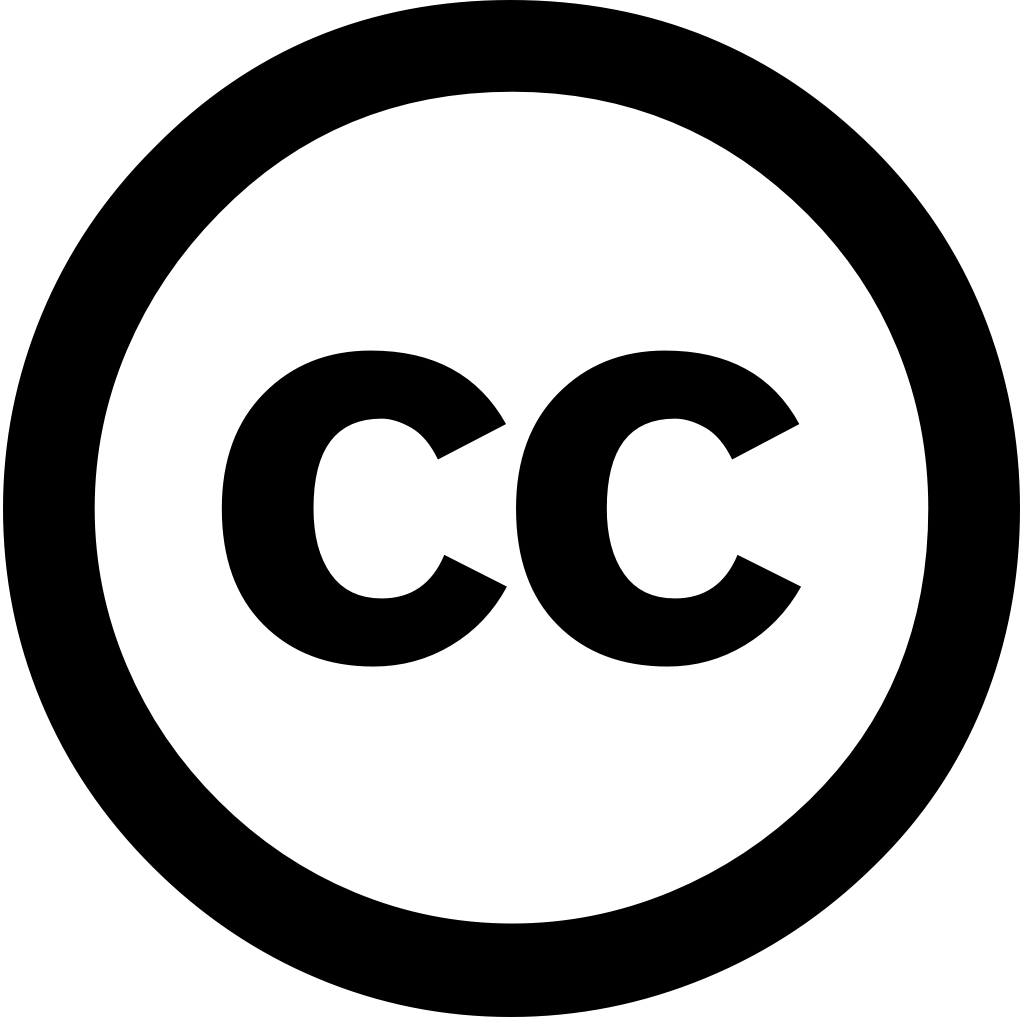
Applied Energy, Год журнала: 2024, Номер 378, С. 124789 - 124789
Опубликована: Ноя. 9, 2024
Язык: Английский
Applied Energy, Год журнала: 2024, Номер 378, С. 124789 - 124789
Опубликована: Ноя. 9, 2024
Язык: Английский
Advances in healthcare information systems and administration book series, Год журнала: 2025, Номер unknown, С. 291 - 322
Опубликована: Янв. 17, 2025
Diabetes Mellitus (DM) is a metabolic disorder when the sugar level in blood elevated consistently. The presence of one global health challenges, several research works focusing on early detection and management innovative machine learning technologies were developed recent years. In this book chapter, we introduce novel approach to classify diabetes mellitus by leveraging Internet Medical Things (IoMT) generative AI models. IoT devices continuously monitor critical data transmit them central model for analysis preprocessing done. preprocessed act as input models predict diabetes. imbalanced dataset converted into balanced using two called VAE GAN. We used five ML classification kNN, SVM, DT, LR RF with boosting. Hard voting performed determine final class. Our experiment result shows that proposed ensemble produces an accuracy 81% which outperformed other model's
Язык: Английский
Процитировано
0Biomedicines, Год журнала: 2025, Номер 13(2), С. 427 - 427
Опубликована: Фев. 10, 2025
The application of artificial intelligence (AI) and machine learning (ML) in medicine healthcare has been extensively explored across various areas. AI ML can revolutionize cardiovascular disease management by significantly enhancing diagnostic accuracy, prediction, workflow optimization, resource utilization. This review summarizes current advancements concerning disease, including their clinical investigation use primary cardiac imaging techniques, common categories, research, patient care, outcome prediction. We analyze discuss commonly used models, algorithms, methodologies, highlighting roles improving outcomes while addressing limitations future applications. Furthermore, this emphasizes the transformative potential practice decision making, reducing human error, monitoring support, creating more efficient workflows for complex conditions.
Язык: Английский
Процитировано
0Sensors, Год журнала: 2025, Номер 25(9), С. 2847 - 2847
Опубликована: Апрель 30, 2025
Federated learning (FL) enables collaborative model training across multiple institutions without the sharing of raw patient data, making it particularly suitable for smart healthcare applications. However, recent studies revealed that merely gradients provides a false sense security, as private information can still be inferred through gradient inversion attacks (GIAs). While differential privacy (DP) provable guarantees, traditional DP methods apply uniform protection, leading to excessive protection low-sensitivity data and insufficient high-sensitivity which degrades performance increases risks. This paper proposes new notion, sensitivity-aware privacy, better balance protection. Our idea is sensitivity each sample objectively measured using real-world attacks. To implement this we develop corresponding defense mechanism adjusts levels based on variation in leakage risks Furthermore, method extends naturally multi-attack scenarios. Extensive experiments medical imaging datasets demonstrate that, under equivalent risk, our achieves an average improvement 13.5% over state-of-the-art methods.
Язык: Английский
Процитировано
0International Journal of Data Science and Analytics, Год журнала: 2025, Номер unknown
Опубликована: Март 24, 2025
Язык: Английский
Процитировано
0Applied Energy, Год журнала: 2024, Номер 378, С. 124789 - 124789
Опубликована: Ноя. 9, 2024
Язык: Английский
Процитировано
2