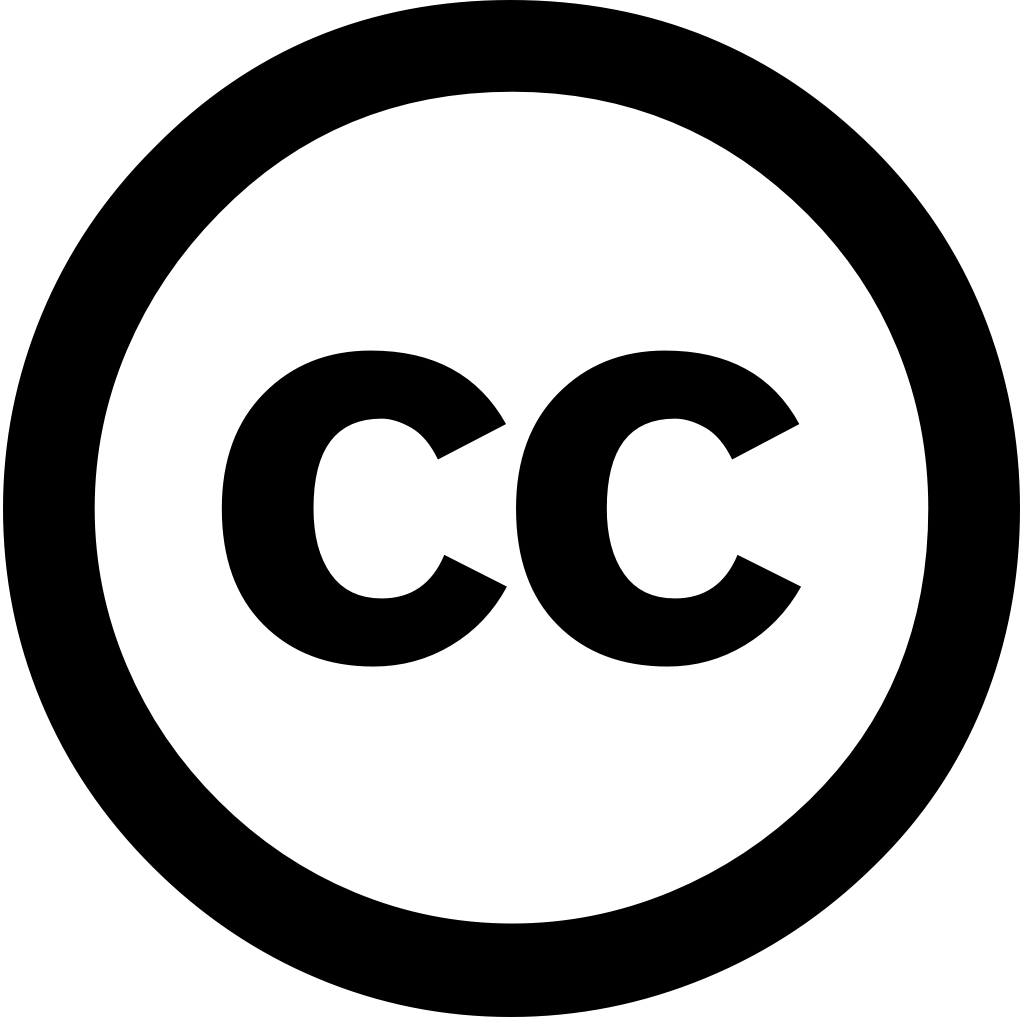
Journal of the Korean Society of Radiology, Год журнала: 2024, Номер 85(5), С. 861 - 861
Опубликована: Янв. 1, 2024
Large language models (LLMs) have revolutionized the global landscape of technology beyond field natural processing. Owing to their extensive pre-training using vast datasets, contemporary LLMs can handle tasks ranging from general functionalities domain-specific areas, such as radiology, without need for additional fine-tuning. Importantly, are on a trajectory rapid evolution, addressing challenges hallucination, bias in training data, high costs, performance drift, and privacy issues, along with inclusion multimodal inputs. The concept small, on-premise open source has garnered growing interest, fine-tuning medical domain knowledge, efficiency managing drift be effectively simultaneously achieved. This review provides conceptual actionable guidance, an overview current technological future directions radiologists.
Язык: Английский