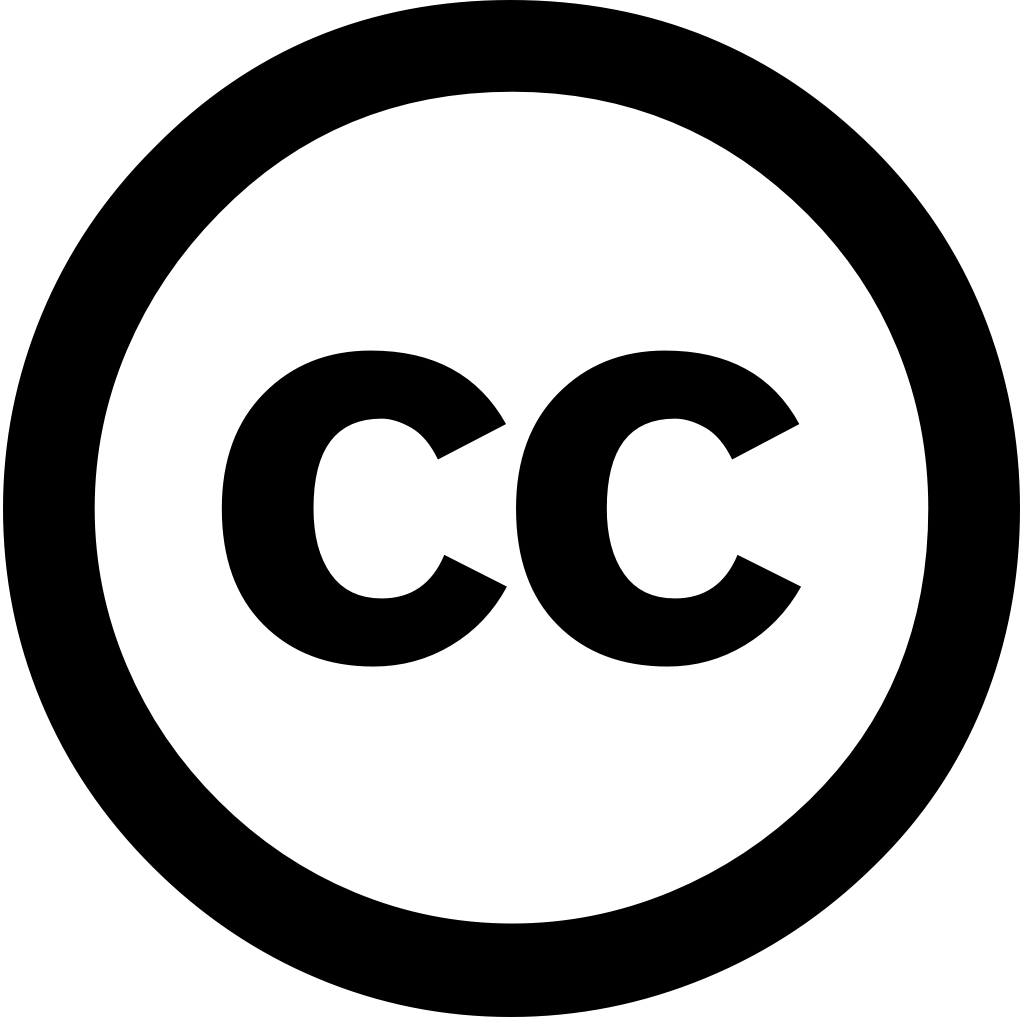
Journal of Environmental Management, Год журнала: 2025, Номер 382, С. 125381 - 125381
Опубликована: Апрель 18, 2025
Enzymes derived from extremophilic organisms, also known as extremozymes, offer sustainable and efficient solutions for industrial applications. Valued their resilience low environmental impact, extremozymes have found use catalysts in various processes, ranging dairy production to pharmaceutical manufacturing. However, discovery of novel is often hindered by challenges such culturing difficulties, underrepresentation extreme environments reference databases, limitations traditional sequence-based screening methods. In this work, we present a computational pipeline designed discover enzymes metagenomic data environments. This represents versatile approach that promotes reuse recycling existing datasets minimises the need additional sampling. its core, algorithm integrates both bioinformatic techniques recent advances structural prediction, enabling rapid accurate identification enzymes. due design, relies heavily on which can limit effectiveness situations where scarce or when encountering protein families. As proof-of-concept, applied deep-sea hydrothermal vents, with focus β-galactosidases. The identified 11 potential candidate proteins, out 10 showed vitro activity. One selected enzymes, βGal_UW07, strong enzyme exhibited optimal activity at 70 °C was exceptionally resistant high pH presence metal ions reducing agents. Overall, our results indicate highly play key role bioprospecting, leveraging minimising situ interventions pristine regions.
Язык: Английский