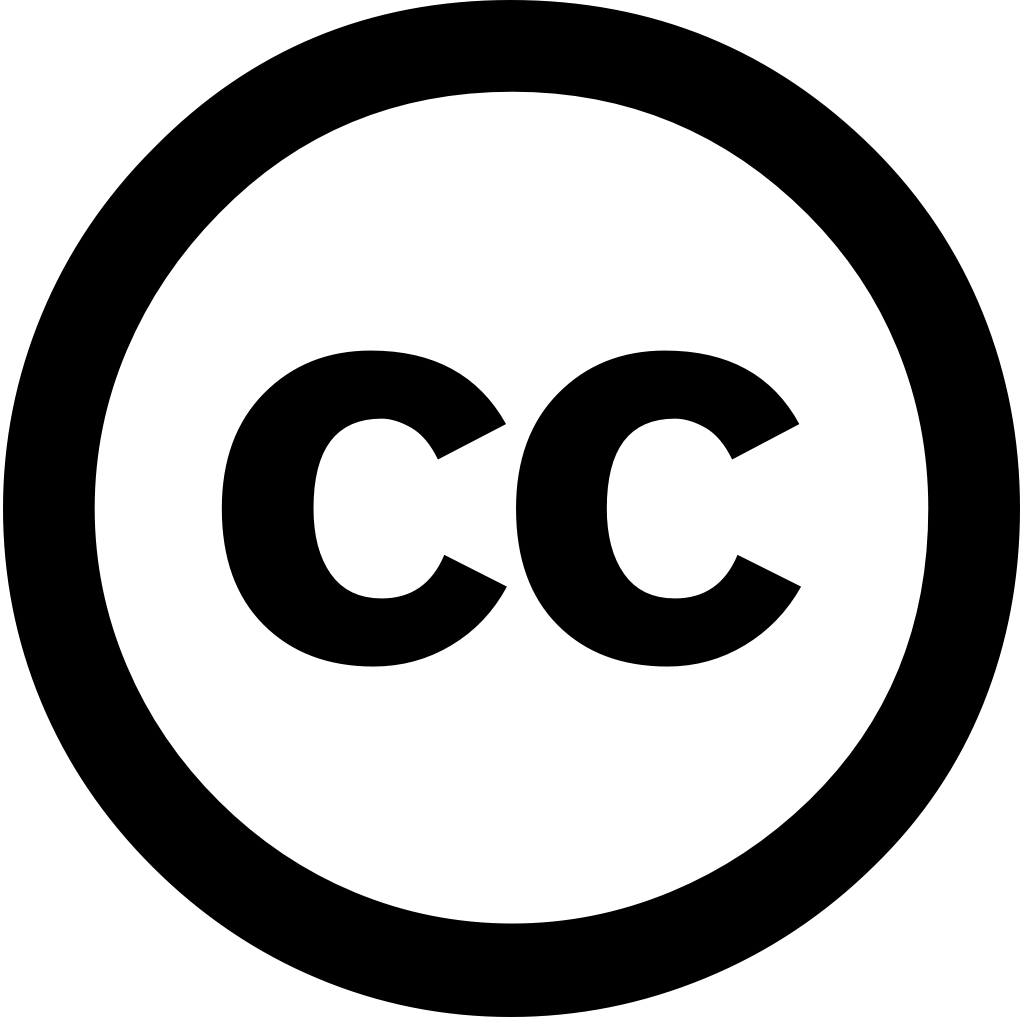
bioRxiv (Cold Spring Harbor Laboratory), Год журнала: 2024, Номер unknown
Опубликована: Авг. 19, 2024
Abstract In the early drug design and discovery phase, virtual screening of diverse small molecule libraries is crucial. Machine learning (ML)-based algorithms have made this process easier faster. study, we applied ML-based to generate QSAR models for screening. The aim study statistically significant identify novel hits against IDH1 mutant receptor crucial glioblastoma multiforme (GBM). To construct models, used both cell lines data (U87 U251 cells) inhibitors reported in literature pIC 50 activity train our models. Furthermore, ligand-based 3D structure-based pharmacophore were also constructed validated.
Язык: Английский